Abstract PO4-17-11: Normalized Breast Cancer Survival Outcomes in U.S. Tumor Registries
Cancer Research(2024)
摘要
Abstract Background The largest U.S. tumor registries, NCDB (National Cancer Database) and SEER (Surveillance, Epidemiology, and End Results Program), are vital sources of epidemiological data and are frequently utilized in research. Although the NCDB includes the majority of patients diagnosed with breast cancer, it is a hospital-based registry. In contrast, SEER is a population-based registry, but includes less than half of all breast cancer patients diagnosed each year. While the US Cancer Statistics Public Use Database (USCS) captures nearly all breast cancers, treatment and survival data are not included. As such, we sought to normalize the NCDB and SEER populations to mirror the USCS population and examine survival outcomes after normalization. Methods All patients diagnosed with stage I-IV breast cancer (2010-2018) were selected from the NCDB and SEER. Frequencies of patients by select characteristics were exported from the USCS. Rates from the USCS were then used to normalize the NCDB and SEER cohorts, using patient weighted frequencies for age, sex, race/ethnicity, tumor biomarkers [estrogen receptor (ER), HER2], and extent of disease (local, regional, distant; anatomic staging data not available in USCS). Of note, the USCS does not have individual patient-level data (only summary data for select variables), and thus, weighted frequencies were used for normalization. The weighted frequencies were calculated based on the total number of patients in the USCS database divided by the total number of patients in the NCDB or SEER separately for each variable (age, sex, race/ethnicity, etc). Weighted and unweighted data were summarized with N (%). Unweighted patient and disease characteristics (crude data) were compared using Chi-square tests. Overall survival (OS) was estimated using the Kaplan-Meier method before and after normalization. Results The USCS cohort included 2,473,739 patients; the NCDB included 1,441,556 and SEER 504,938. The median followup was 54.9 months for NCDB and 57 months for SEER. There were minimal differences between the cohorts based on age (age < 50y: USCS 18.1%, NCDB 19.3%, SEER 18.9%) or sex (female: USCS 99.1%, NCDB 99.1%, SEER 99.3%). However, there were notable differences in the racial/ethnic composition; non-Hispanic White: USCS 75%, NCDB 78.2%, SEER 68.2%; non-Hispanic Black: USCS 11.4%, NCDB 11.4%, SEER 9.9%; Hispanic: USCS 8.3%, NCDB 5.9%, SEER 11.7%; p< 0.001 for USCS vs NCDB and USCS vs SEER). There were minimal differences in tumor biomarkers (ER+: USCS 82.9%, NCDB 83%, SEER 84.9%; HER2+: USCS 14.5%, NCDB 14.1%, SEER 13.8%), but significant differences in extent of disease (local: USCS 66.1%, NCDB 80.2%, SEER 68.4%; distant: USCS 6%, NCDB 3.9%, SEER 3.9%; p< 0.001 for USCS vs NCDB and USCS vs SEER). For the variables that were similar without weighting (age, sex, tumor biomarkers), OS was also similar after weighting (Table). After normalizing the NCDB based on race, 8-year OS remained comparable (crude 77.2% vs weighted 77.3%); similar findings were noted after normalizing SEER (crude 75.8% vs weighted 75.3%). After normalizing the NCDB based on extent of disease, 8-year OS was notably worse (crude 77.2% vs weighted 74.2%); similar findings were noted for SEER (crude 75.8% vs weighted 74.4%). Conclusions While national tumor registries afford researchers the opportunity to study large breast cancer cohorts, they do not always fully represent the entire breast cancer population. This limitation should be considered when working with these data sets. Table. Citation Format: Tori Chanenchuk, Kerri-Anne Crowell, Samantha Thomas, Rachel Greenup, Jennifer Plichta. Normalized Breast Cancer Survival Outcomes in U.S. Tumor Registries [abstract]. In: Proceedings of the 2023 San Antonio Breast Cancer Symposium; 2023 Dec 5-9; San Antonio, TX. Philadelphia (PA): AACR; Cancer Res 2024;84(9 Suppl):Abstract nr PO4-17-11.
更多查看译文
AI 理解论文
溯源树
样例
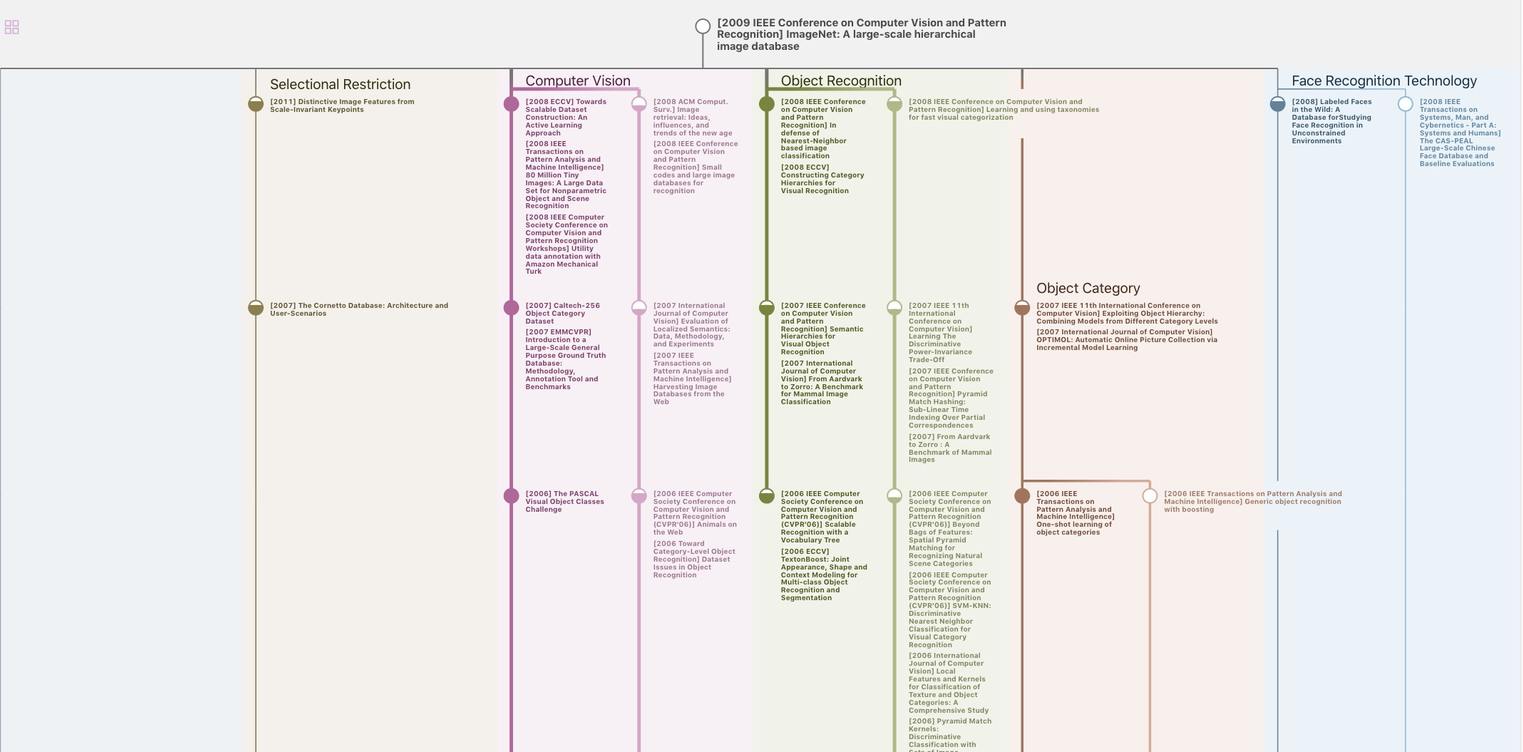
生成溯源树,研究论文发展脉络
Chat Paper
正在生成论文摘要