Reset-Free Reinforcement Learning via Multi-State Recovery and Failure Prevention for Autonomous Robots
Tsinghua Science and Technology(2024)
摘要
Reinforcement learning holds promise in enabling robotic tasks as it can learn optimal policies via trial and error. However, the practical deployment of reinforcement learning usually requires human intervention to provide episodic resets when a failure occurs. Since manual resets are generally unavailable in autonomous robots, we propose a reset-free reinforcement learning algorithm based on multi-state recovery and failure prevention to avoid failure-induced resets. The multi-state recovery provides robots with the capability of recovering from failures by self-correcting its behavior in the problematic state and, more importantly, deciding which previous state is the best to return to for efficient re-learning. The failure prevention reduces potential failures by predicting and excluding possible unsafe actions in specific states. Both simulations and real-world experiments are used to validate our algorithm with the results showing a significant reduction in the number of resets and failures during the learning.
更多查看译文
关键词
reinforcement learning,manual reset,multi-state recovery,failure prediction
AI 理解论文
溯源树
样例
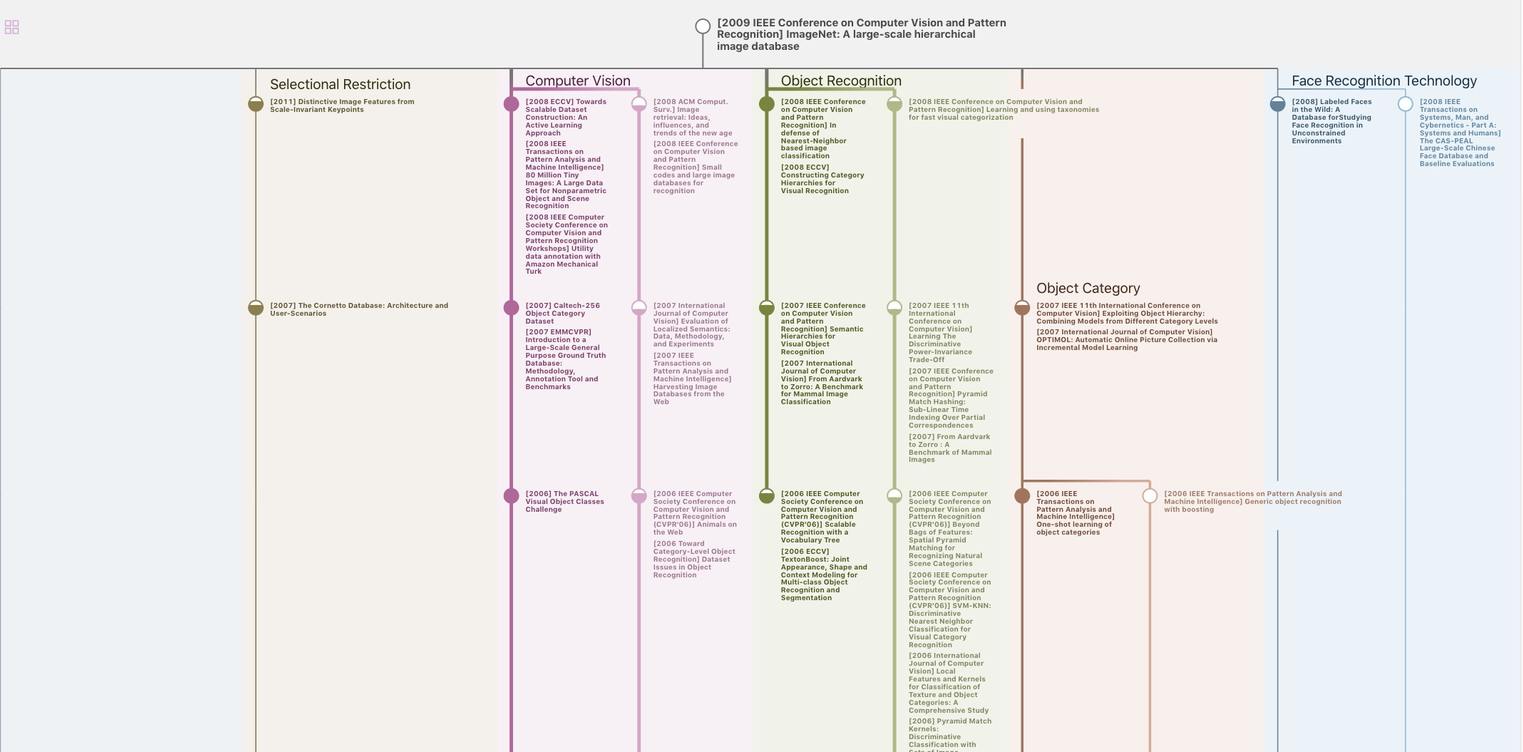
生成溯源树,研究论文发展脉络
Chat Paper
正在生成论文摘要