Creating realistic anterior segment optical coherence tomography images using generative adversarial networks.
The British journal of ophthalmology(2024)
摘要
AIMS:To develop a generative adversarial network (GAN) capable of generating realistic high-resolution anterior segment optical coherence tomography (AS-OCT) images.
METHODS:This study included 142 628 AS-OCT B-scans from the American University of Beirut Medical Center. The Style and WAvelet based GAN architecture was trained to generate realistic AS-OCT images and was evaluated through the Fréchet Inception Distance (FID) Score and a blinded assessment by three refractive surgeons who were asked to distinguish between real and generated images. To assess the suitability of the generated images for machine learning tasks, a convolutional neural network (CNN) was trained using a dataset of real and generated images over a classification task. The generated AS-OCT images were then upsampled using an enhanced super-resolution GAN (ESRGAN) to achieve high resolution.
RESULTS:The generated images exhibited visual and quantitative similarity to real AS-OCT images. Quantitative similarity assessed using FID scored an average of 6.32. Surgeons scored 51.7% in identifying real versus generated images which was not significantly better than chance (p value >0.3). The CNN accuracy improved from 78% to 100% when synthetic images were added to the dataset. The ESRGAN upsampled images were objectively more realistic and accurate compared with traditional upsampling techniques by scoring a lower Learned Perceptual Image Patch Similarity of 0.0905 compared with 0.4244 of bicubic interpolation.
CONCLUSIONS:This study successfully developed and leveraged GANs capable of generating high-definition synthetic AS-OCT images that are realistic and suitable for machine learning and image analysis tasks.
更多查看译文
AI 理解论文
溯源树
样例
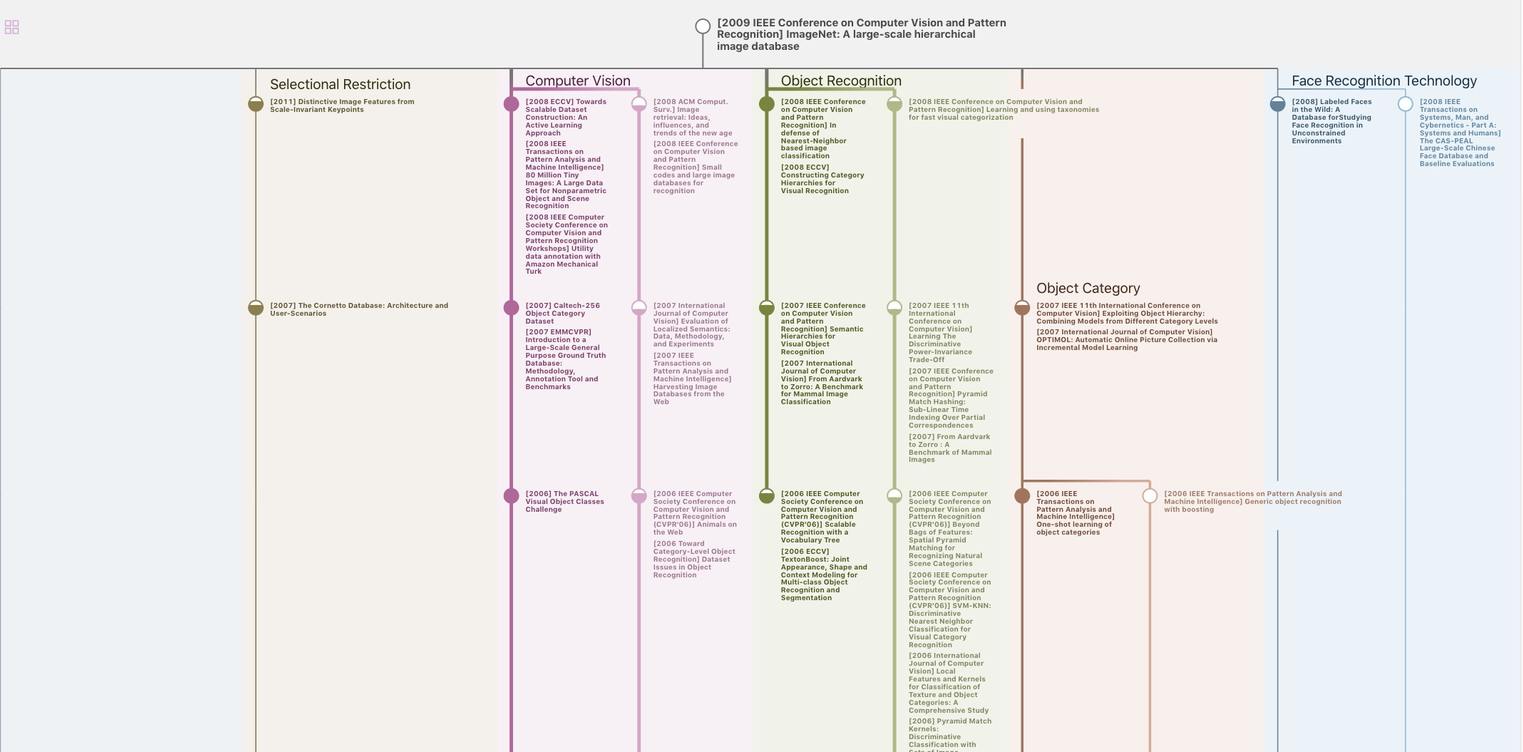
生成溯源树,研究论文发展脉络
Chat Paper
正在生成论文摘要