A Category-Aware Curriculum Learning for Data-Free Knowledge Distillation
IEEE transactions on multimedia(2024)
摘要
Constructing effective proxy data is one of the core challenges in data-free knowledge distillation. The existing models ignore the influence of the category entanglement of the generated data on the distillation. To alleviate this issue, imitating the human learning process, a new category-aware curriculum learning mechanism is proposed in this paper to perform data-free knowledge distillation, called CCL-D. The main ideology of this category-aware curriculum learning mechanism is to provide a new learning mode for data generation and network training, which enables the model to realize the knowledge distillation process from easy to difficult through automated curriculum learning. In this novel learning mechanism, a category-aware monitoring module is proposed to constrain the category attribute of generated data. Based on this monitoring module, the curriculum learning process for data generation and network training is designed and applied. Initially, the generator is guided to obtain new data with clear category features. The utilization of data with apparent category features is easy for student network training, and it enables the student network to learn clear and significant category features at the early training stage. Subsequently, the generator is guided to generate data with category entanglement. Utilizing these new data with category entanglement problems can improve the recognition ability of the student network to interclass interference and enhance network robustness. The effectiveness of the CCL-D is verified on the six benchmark experimental datasets (MNIST, CIFAR-10, CIFAR-100, SVHN, Caltech-101, Tiny-Imagenet).
更多查看译文
关键词
data generation,knowledge distillation,category-aware,curriculum learning,image classification
AI 理解论文
溯源树
样例
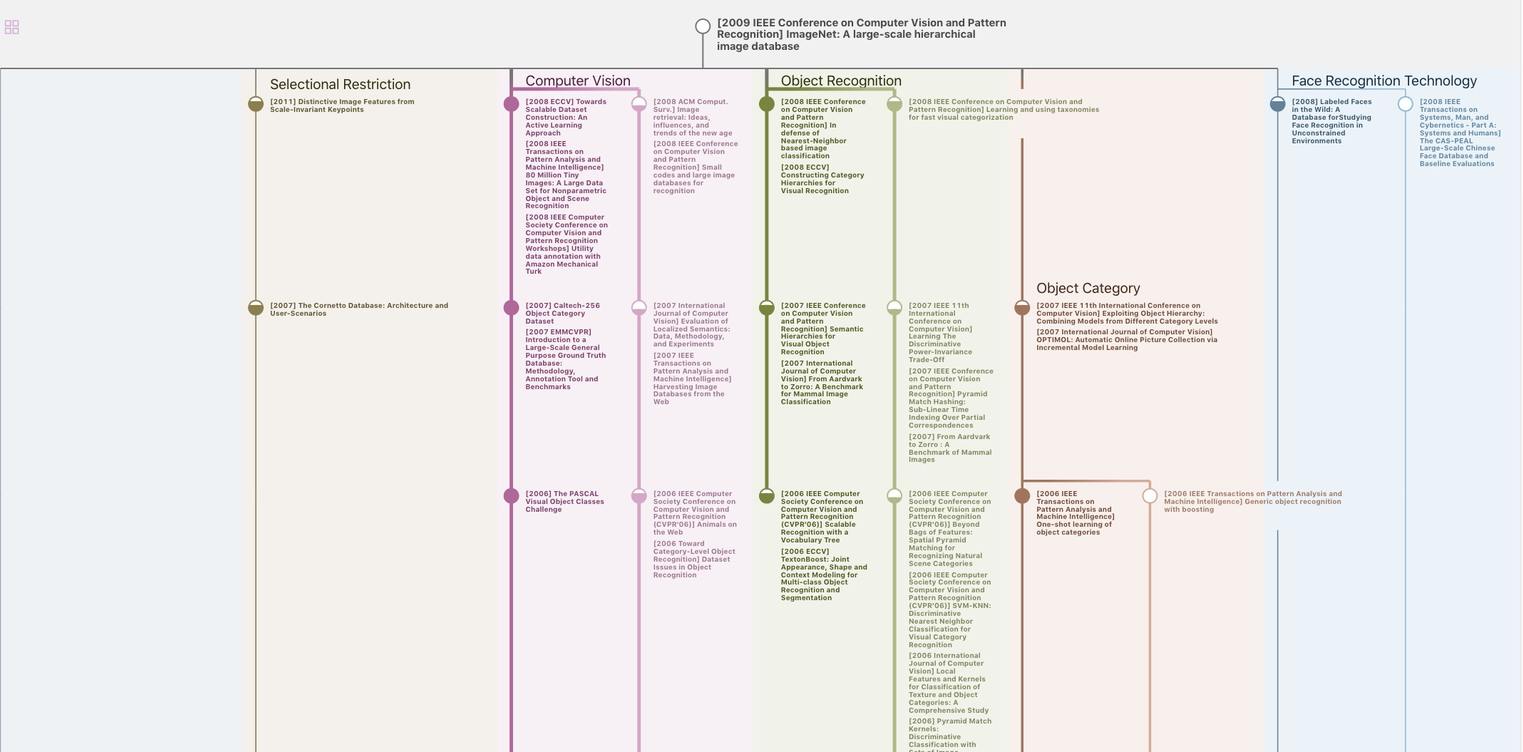
生成溯源树,研究论文发展脉络
Chat Paper
正在生成论文摘要