A Machine Learning Approach to Analysis of Canadian Provincial Power System Decarbonization
Energy Reports(2024)
摘要
Canada committed to reducing national greenhouse gas emissions by 40-45 % by 2030 and achieving net-zero by 2050, relying on low-carbon electrification across economic sectors. This study investigates the machine learning's potential to analyze least-cost optimization of Canadian provincial power systems to 2050, to identify robust pathways for net-zero electricity system greenhouse gas emissions, and to provide policy insights respecting wind and solar generation, reducing emissions from natural gas generation, and expanding interprovincial transmission. Surrogate modeling is applied as sensitivities to generation technology capital costs, electricity demand, and carbon pricing to results from an existing capacity expansion mixed-integer linear optimization model. Under almost all future scenarios, six provinces develop new wind generation, with substantial deployment in Alberta (mean 27.3 GW, max. 62.7 GW), Ontario (mean 6.8 GW, max. 19.3 GW), Saskatchewan (mean 3.6 GW, max 8.9 GW) and Manitoba (mean 1.5 GW, max 3.5 GW). With the potential exception of BC, where higher-cost wind generation results in solar generation becoming the primary low-carbon electrical energy source (mean 25.2 GW), future solar generation across Canada is more limited (mean 37.1 GW) and less certain than wind generation in all other Canadian provinces. Existing natural gas generation is maintained in almost all scenarios in all provinces, providing support for maintaining these resources for reliability and reserve requirements. Very limited natural gas is developed under any scenarios, with the exception of British Columbia (mean 4.3 GW) where more than 80 % of scenarios include new natural gas capacity. Additional transmission transfer capacity is developed in most scenarios between BC-Alberta (-60 % of scenarios), SaskatchewanManitoba (-85 %), and Ontario-Quebec (-75 %). Carbon prices, along with wind and solar capital costs, act as primary determinants of least-cost decarbonization pathways. These findings offer insights for Canadian policymakers into net-zero pathways for provincial electricity systems that remain robust despite variability in inputs.
更多查看译文
关键词
Provincial policy planning,Decarbonization pathways,Energy systems modeling,machine learning
AI 理解论文
溯源树
样例
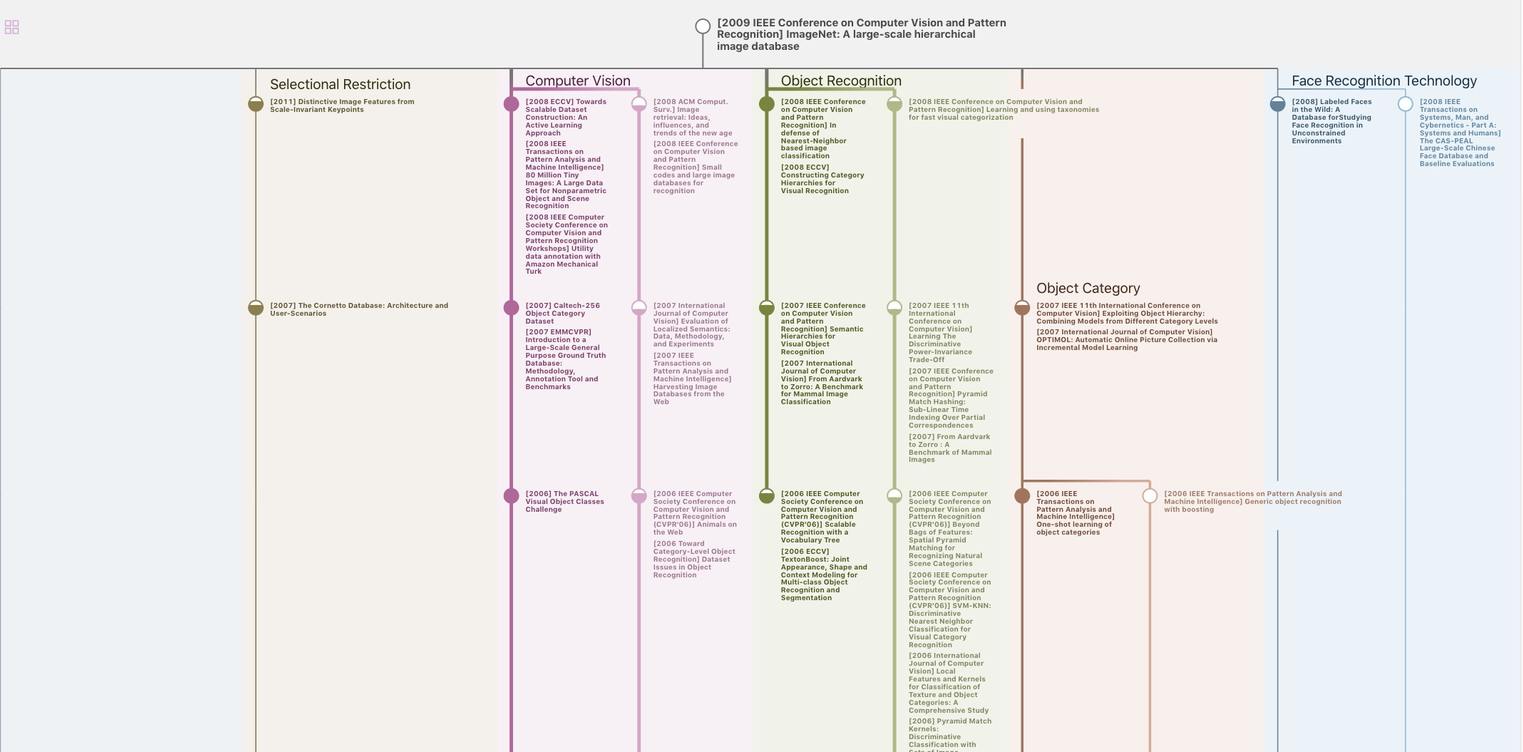
生成溯源树,研究论文发展脉络
Chat Paper
正在生成论文摘要