SCIseg: Automatic Segmentation of T2-weighted Intramedullary Lesions in Spinal Cord Injury.
medRxiv : the preprint server for health sciences(2024)
摘要
Purpose:To develop a deep learning tool for the automatic segmentation of T2-weighted intramedullary lesions in spinal cord injury (SCI).
Material and Methods:This retrospective study included a cohort of SCI patients from three sites enrolled between July 2002 and February 2023. A deep learning model, SCIseg , was trained in a three-phase process involving active learning for the automatic segmentation of intramedullary SCI lesions and the spinal cord. The data consisted of T2-weighted MRI acquired using different scanner manufacturers with heterogeneous image resolutions (isotropic/anisotropic), orientations (axial/sagittal), lesion etiologies (traumatic/ischemic/hemorrhagic) and lesions spread across the cervical, thoracic and lumbar spine. The segmentations from the proposed model were visually and quantitatively compared with other open-source baselines. Wilcoxon signed-rank test was used to compare quantitative MRI biomarkers (lesion volume, lesion length, and maximal axial damage ratio) computed from manual lesion masks and those obtained automatically with SCIseg predictions.
Results:MRI data from 191 SCI patients (mean age, 48.1 years ± 17.9 [SD]; 142 males) were used for model training and evaluation. SCIseg achieved the best segmentation performance for both the cord and lesions. There was no statistically significant difference between lesion length and maximal axial damage ratio computed from manually annotated lesions and those obtained using SCIseg .
Conclusion:Automatic segmentation of intramedullary lesions commonly seen in SCI replaces the tedious manual annotation process and enables the extraction of relevant lesion morphometrics in large cohorts. The proposed model segments lesions across different etiologies, scanner manufacturers, and heterogeneous image resolutions. SCIseg is open-source and accessible through the Spinal Cord Toolbox.
Summary:Automatic segmentation of the spinal cord and T2-weighted lesions in spinal cord injury on MRI scans across different treatment strategies, lesion etiologies, sites, scanner manufacturers, and heterogeneous image resolutions.
Key Results:An open-source, automatic method, SCIseg , was trained and evaluated on a dataset of 191 spinal cord injury patients from three sites for the segmentation of the spinal cord and T2-weighted lesions. SCIseg generalizes across traumatic and non-traumatic lesions, scanner manufacturers, and heterogeneous image resolutions, enabling the automatic extraction of lesion morphometrics in large multi-site cohorts. Quantitative MRI biomarkers, namely, lesion length and maximal axial damage ratio derived from the automatic predictions showed no statistically significant difference when compared with manual ground truth, implying reliability in SCIseg's predictions.
更多查看译文
AI 理解论文
溯源树
样例
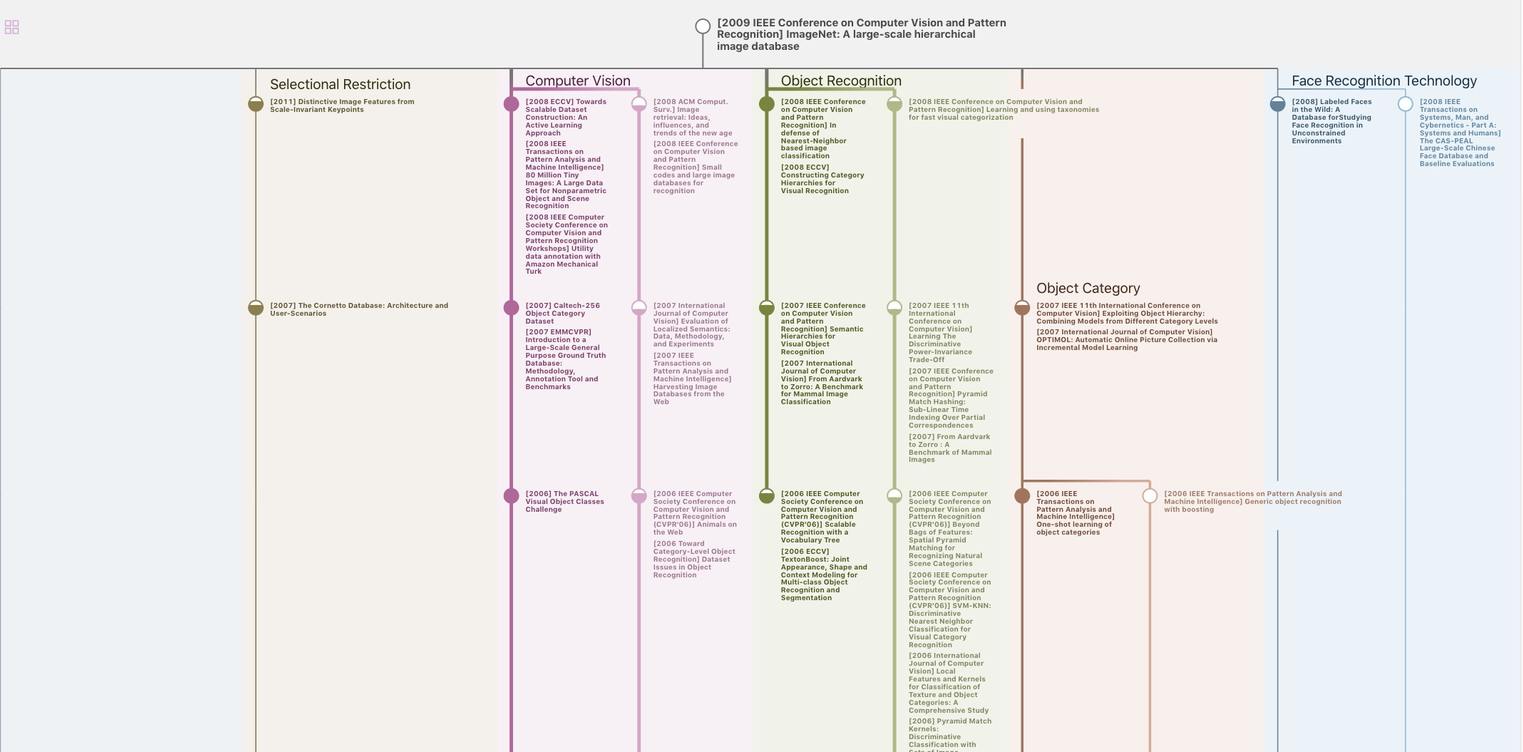
生成溯源树,研究论文发展脉络
Chat Paper
正在生成论文摘要