Identification research of chemical process leakage based on deep learning and correlation-distance graph coding
Process Safety and Environmental Protection(2024)
摘要
As a leak identification method in chemical processes, deep learning is limited by inadequate sample labels and highly coupled, nonlinear process variables, therefore difficult to model with a high identification accuracy. To address these problems, a fusion correlation-distance graph (CDG) coding aided deep learning method is proposed. First, the mechanism model is built based on dynamic process data with different leakage labels. Then, the weighted degree and spatial distribution of the dynamic dataset are computed through innovatively designed correlation and distance coding, aided by sliding window fusion into a correlation-distance matrix. Finally, the well-trained long short-term memory (LSTM) is constructed to learn the deep features of the leakage process and identify them. The applications in two industrial cases, CO2 capture and ammonia synthesis, show that CDG-LSTM presents state-of-the-art identification performance with R2 scores of 0.994 and 0.986. 6.6% and 18.4% improvement over LSTM. In addition, the proposed method effectively categorizes the unknown leak samples into known labels with nearby locations, providing a valuable reference for operators to determine the unknown leak locations.
更多查看译文
关键词
Correlation-distance coding,Chemical process,LSTM,Leak identification,Unlabeled samples
AI 理解论文
溯源树
样例
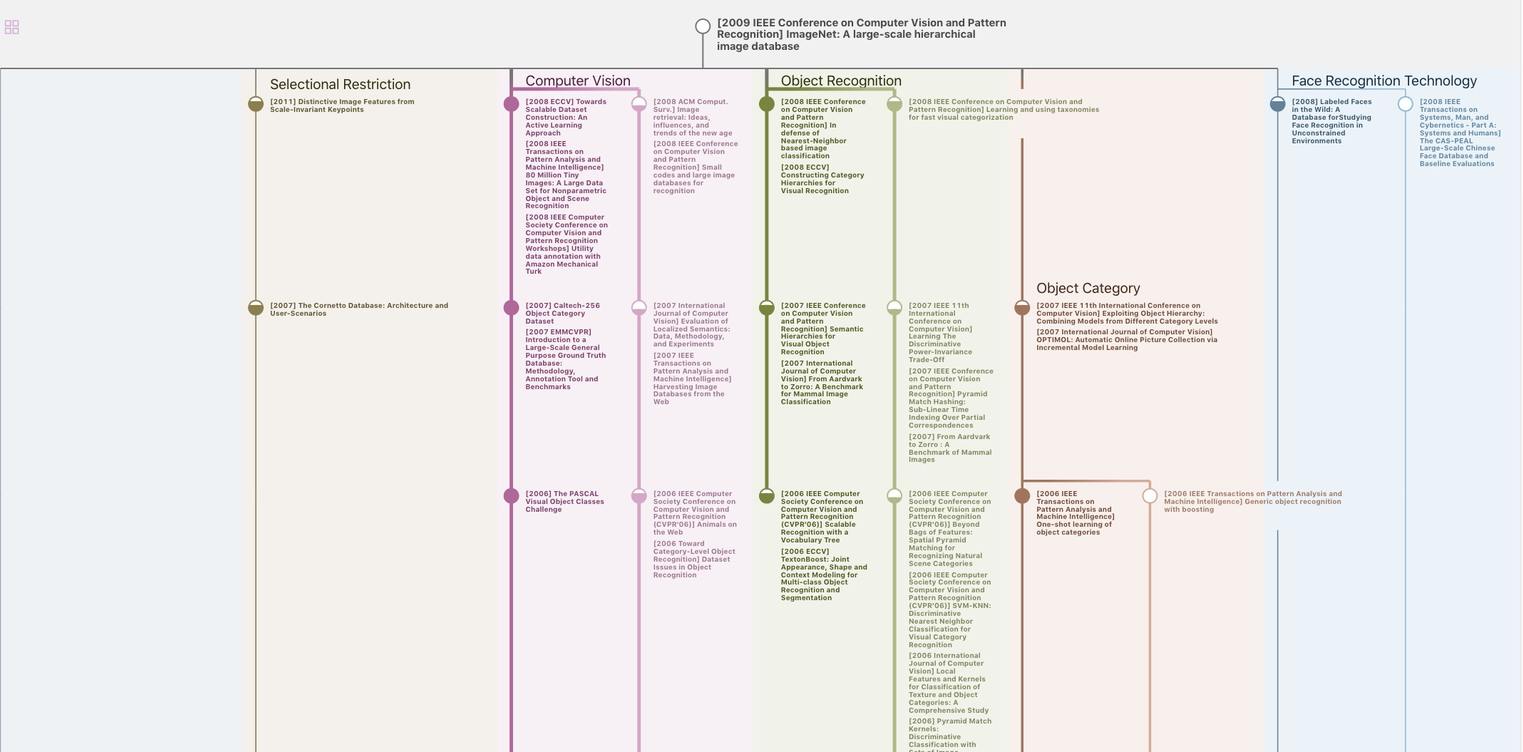
生成溯源树,研究论文发展脉络
Chat Paper
正在生成论文摘要