Tool wear status monitoring under laser-ultrasonic compound cutting based on acoustic emission and deep learning
Journal of Mechanical Science and Technology(2024)
摘要
In order to solve the problem of untimely monitoring of tool wear status during laser-ultrasonic compound cutting (LUCC), which leads to the reduction of process accuracy and product quality damage, a method of tool wear status monitoring based on acoustic emission (AE) and deep learning is proposed in this paper. The AE signal is collected and analyzed in the frequency and time-frequency domains by using Fourier transform (FT) and wavelet packet decomposition (WPD), it is found that the AE signal energy is mainly concentrated in the 1st, 2nd, 3rd, and 4th frequency bands, and the energy of each frequency band in the signal increases gradually when the tool wear degree increases, however, the percentage of energy of each frequency band in the total energy shows different change trend. According to the amount of flank wear of the tool, the tool wear degree is divided into four tool wear states. The SVM model and BP neural network model were established based on MATLAB software to realize the tool wear state monitoring. The results show that the tool wear monitoring based on BP neural network is better than that of SVM for tool wear status monitoring. The model identification correct rate reaches 99
更多查看译文
关键词
Tool wear condition monitoring,Acoustic emission signal,Time-frequency domain analysis,Deep learning
AI 理解论文
溯源树
样例
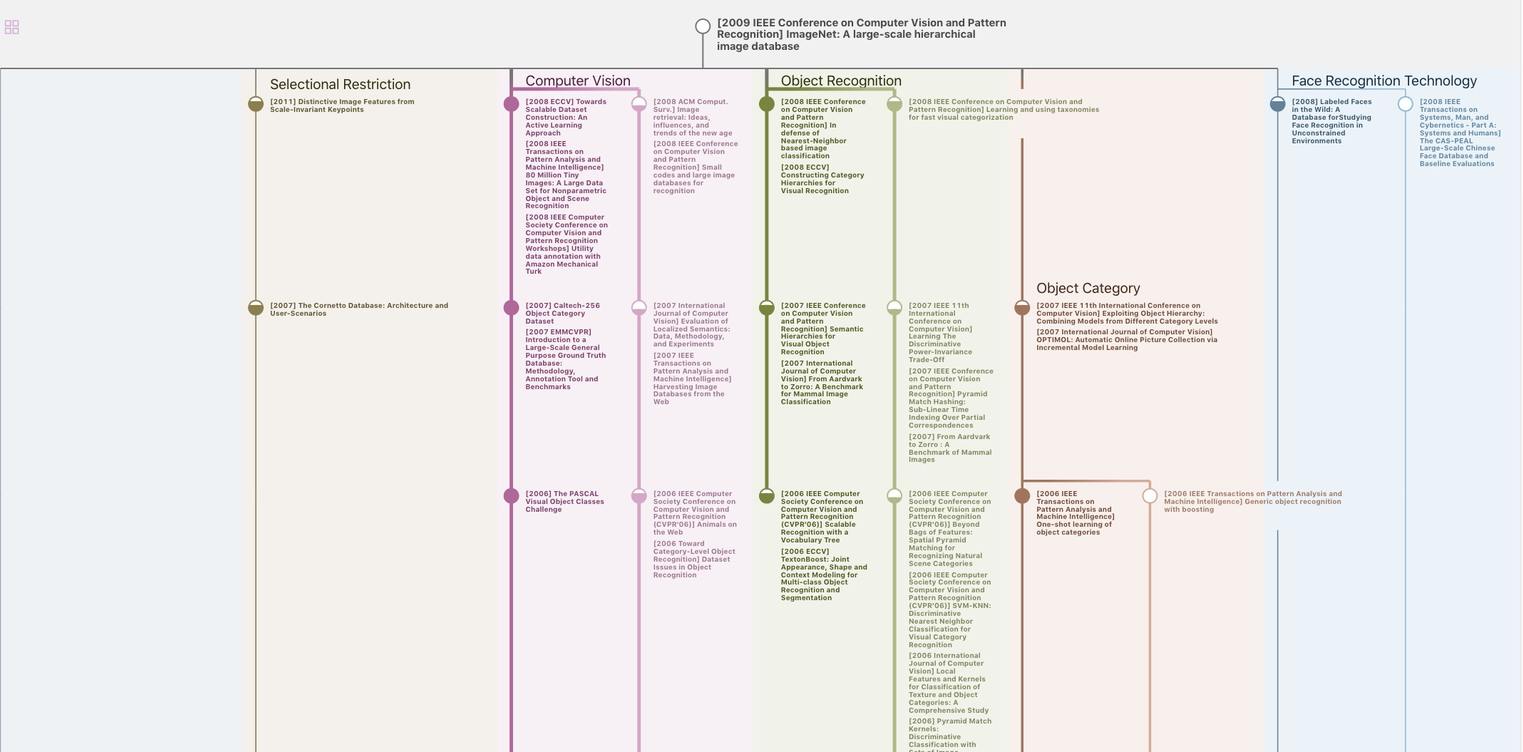
生成溯源树,研究论文发展脉络
Chat Paper
正在生成论文摘要