Search for a Dual-Convergence Sparse Feature Extractor With Visualization Vibration Signals Architecture Feature for Flip-Chip Defect Detection
IEEE Transactions on Industrial Informatics(2024)
摘要
The widespread use of flip-chip technology in the field of microelectronics packaging makes defect detection technology face great challenges, which requires the development of detection technology with less manual intervention, a lightweight network architecture, and high precision. Aiming at the dual-stability of the neural architecture search (NAS), and explicitly associating the network architecture searched by NAS with the architecture features of vibration signals. This study proposes a dual-convergence sparse feature extractor (DSFE) for visualization vibration signals architecture feature searching, which attempts to learn a representation whose architecture feature can uniquely represent the intrinsic information of the target signal. DSFE analyzed and summarized three deficiencies of gradient-based NAS, namely, “huge GPU memory consumption,” “high collapse probability,” and “rigid number of node precursor operations.” The corresponding solution modules are proposed in turn, which are “primary and secondary search spaces,” “skip_connect coefficient modification,” and “dynamic sparse selection of precursor operations.” The effectiveness of DSFE is verified with flip-chip vibration signals excited by the air-coupled ultrasonic wave. By comprehensive comparative experiments with fixed-structure networks and gradient-based NAS methods, it is proved that DSFE cannot only achieve dual-stability of detection precision and architecture searched, but also ensure less resource consumption and multifarious architecture, which can broaden the application scope of DSFE in practical engineering.
更多查看译文
关键词
Architecture feature,defect detection,flip-chip,neural architecture search (NAS),sparse representation,vibration signals
AI 理解论文
溯源树
样例
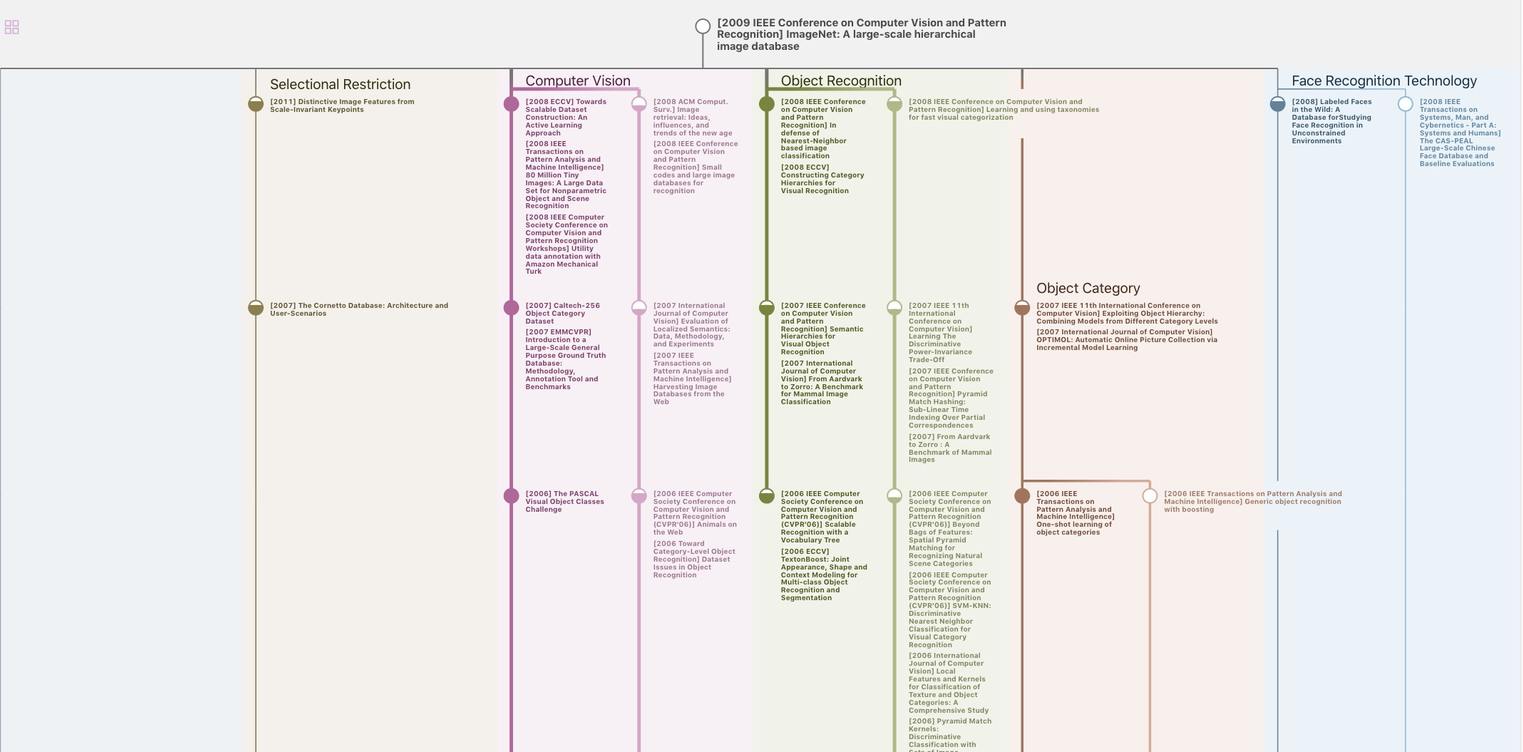
生成溯源树,研究论文发展脉络
Chat Paper
正在生成论文摘要