Prediction of Engineering Characteristics of Rock Masses Using Actual TBM Performance Data with Supervised and Unsupervised Learning Algorithms (a Case Study in Strong to Very Strong Igneous and Pyroclastic Rocks)
Rock Mechanics and Rock Engineering(2024)
Abstract
Knowing accurate values of rock engineering parameters in hard rock condition is the key in estimating and adjusting TBM performance. In this study, TBM penetration per revolution (Pr) as driving parameter, TBM operational parameters including cutterhead torque (Tq), field penetration index (FPI), thrust force (Th), cutter load (Fn), cutterhead rotational speed revolution per minute (RPM), and also cutterhead diameter as a mechanical machine specification are used to predict geomechanical parameters including rock quality designation (RQD) and unconfined compressive strength (UCS) based on reverse analysis in three types of strong rocks (Tuff, Andesite, Diorite) along the Southern lot of Kerman water transfer tunnel; KrWCT. A soft computing method called least squares-support vector machine (Ls-SVM), is trained using operating and driving data as well as rock engineering parameters gathered from the KrWCT project which is excavated in hard rock condition using double shield TBM. Due to the lack of access to the excavation face in mechanized tunneling, the collection of geomechanical data on the rock mass is possible only through the examination of excavated material and limited inspections during cutterhead repairs. Therefore, this paper has tried to predict and classify the rock engineering factors using some indicators related to machine performance, mechanical specification, and TBM operational parameters. Among the data collected during the pre-construction and construction phases of the project, the dataset was classified into 42 zones, and the reasonable distribution intervals of the main tunneling factors corresponding to each zone were defined. New empirical equations are developed to estimate the properties of igneous rocks based on linear and non-linear multivariable regression (MR). Several loss functions were used to assess the performance and precision of the applied methods. The results of the proposed models indicate an acceptable and reliable accuracy.
MoreTranslated text
Key words
Rock Engineering Parameters,Machine Learning,TBM,Hard Rock Condition,Reversed Analysis
AI Read Science
Must-Reading Tree
Example
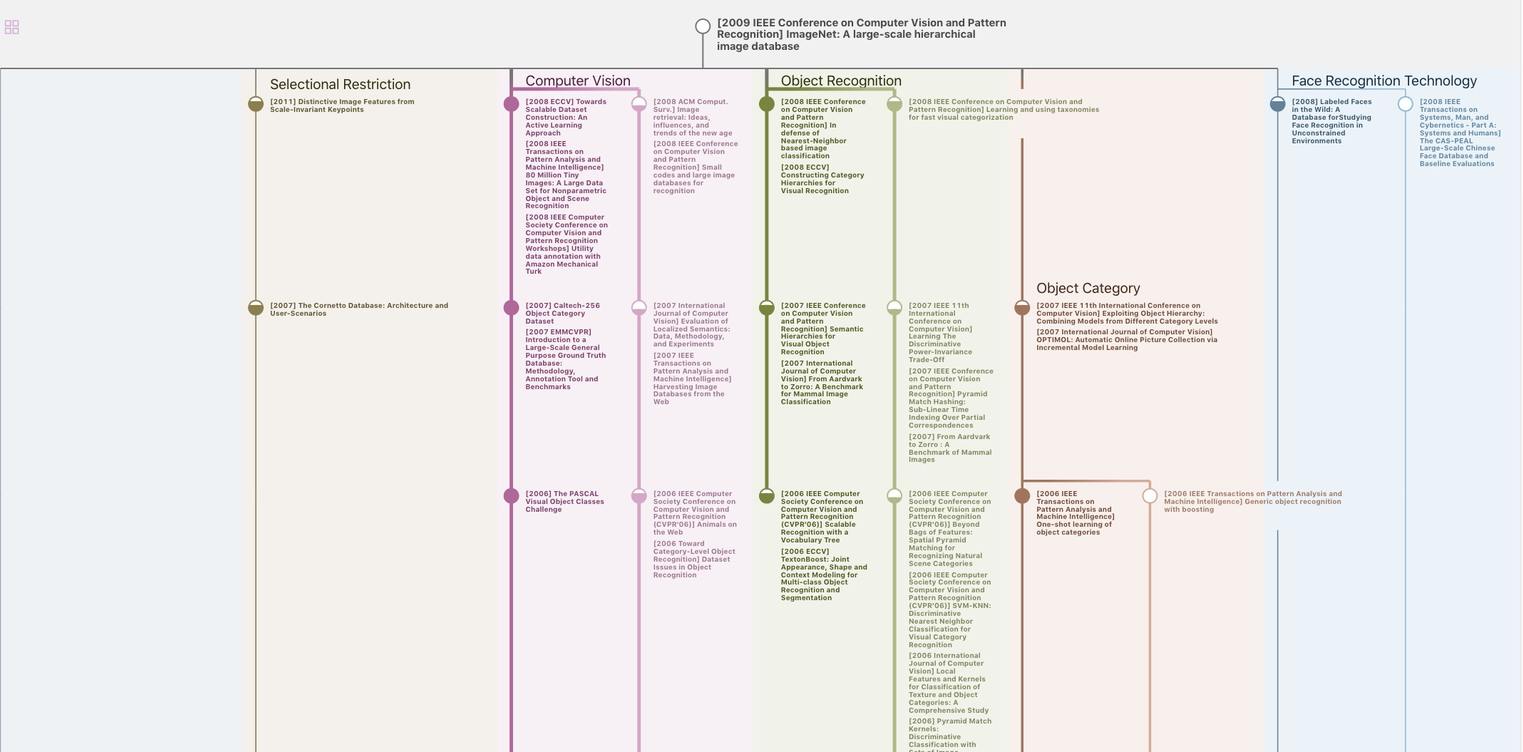
Generate MRT to find the research sequence of this paper
Chat Paper
Summary is being generated by the instructions you defined