Assessment of Roughness Characterization Methods for Data-Driven Predictions
Flow, Turbulence and Combustion(2024)
摘要
A comparative analysis is undertaken to explore the impact of various roughness characterization methods as input variables on the performance of data-driven predictive models for estimating the roughness equivalent sand-grain size k_s . The first type of model, denoted as ENN_PS , incorporates the roughness height probability density function (p.d.f.) and power spectrum (PS), while the second type of model, ENN_PA , utilizes a finite set of 17 roughness statistical parameters as input variables. Furthermore, a simplified parameter-based model, denoted as ENN_PAM , is considered, which features only 6 input roughness parameters. The models are trained based on identical databases and evaluated using roughness samples similar to the training databases as well as an external testing database based on literature. While the predictions based on p.d.f. and PS achieves a stable error level of around 10 ENN_PAM , which is not observed for ENN_PA . We hypothesize that the successful training of ENN_PAM is attributed to the enhanced training efficiency linked to the lower input dimensionality.
更多查看译文
关键词
Turbulence,Roughness,Machine learning
AI 理解论文
溯源树
样例
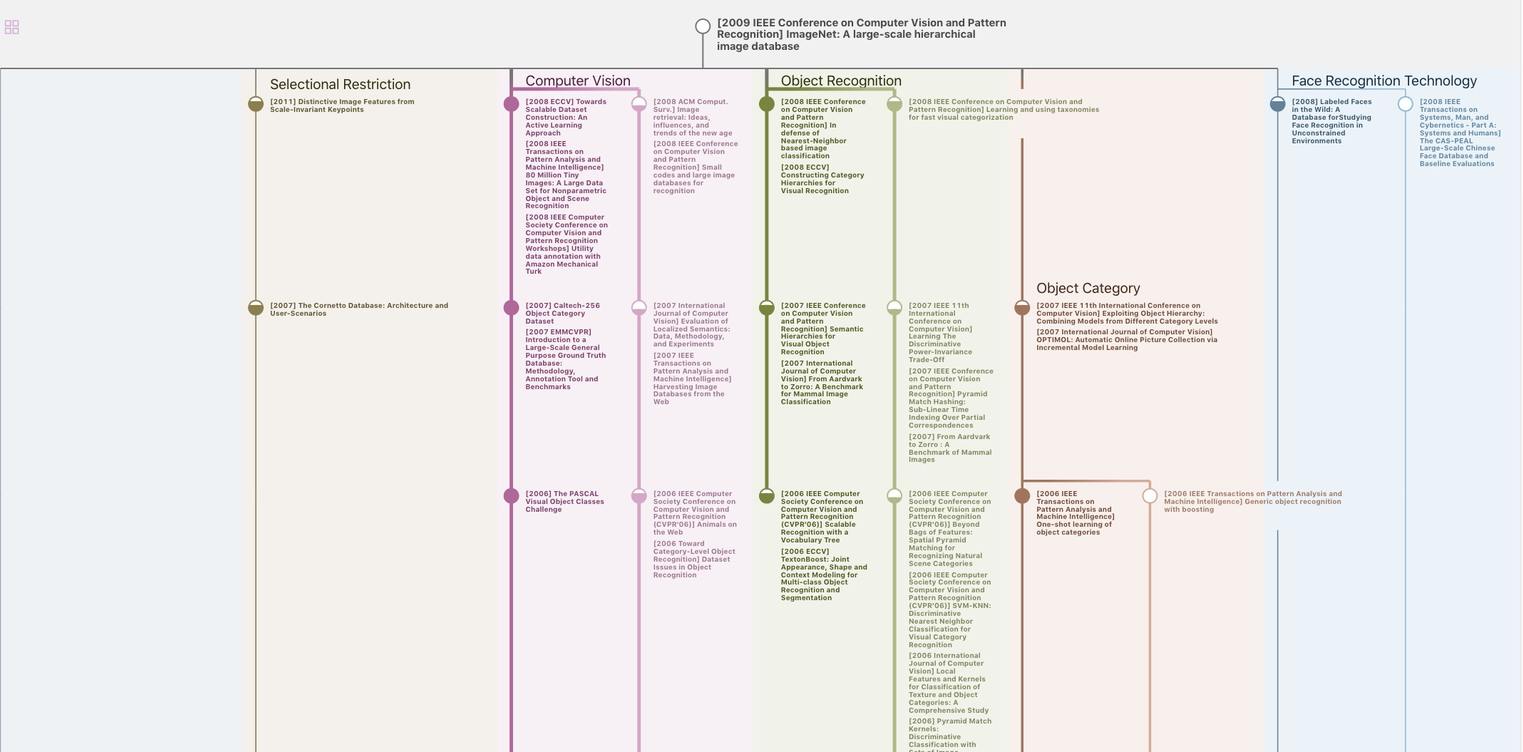
生成溯源树,研究论文发展脉络
Chat Paper
正在生成论文摘要