Power load forecasting based on spatial–temporal fusion graph convolution network
Technological Forecasting and Social Change(2024)
摘要
The cyclical behavior of electricity users facilitates the prediction of future electricity loads through the analysis of historical data, in addition to considering the spatial correlation among various electricity units. Building on these observations, this study transforms the power loads generated by regional-level user units into graph signals for a more effective visualization of spatial–temporal electricity consumption patterns. Specifically, the graph-based model harnesses its unique spatial–temporal dependencies to deduce the dynamics of electricity consumption patterns and enhance the precision of electricity generation predictions. Introducing a novel deep learning model, the Spatial-Temporal Fusion Graph Convolutional Network (FSTGRNN), which integrates temporal and spatial correlations for the prediction of electricity load values at future time steps. FSTGRNN is an end-to-end model that, firstly, delineates the spatial and temporal patterns of electric loads by constructing spatial relationship graphs using the FC-LSTM and GC-GRU models designed within the spatial–temporal block (ST-Block). Secondly, the spatial–temporal fusion block (STF-Block) in FSTGRNN, equipped with a gating mechanism, encodes the graph signals to capture both long-term dependencies and short-term correlations. The resulting integrated representation is utilized for the forecasting process. Experimental validation is conducted on two electricity load datasets, and the forecasting results clearly demonstrate the superiority and efficiency of the proposed deep learning system.
更多查看译文
关键词
Spatial–temporal correlation,Load forecasting,Graph neural network,Multi-task learning,Deep learning
AI 理解论文
溯源树
样例
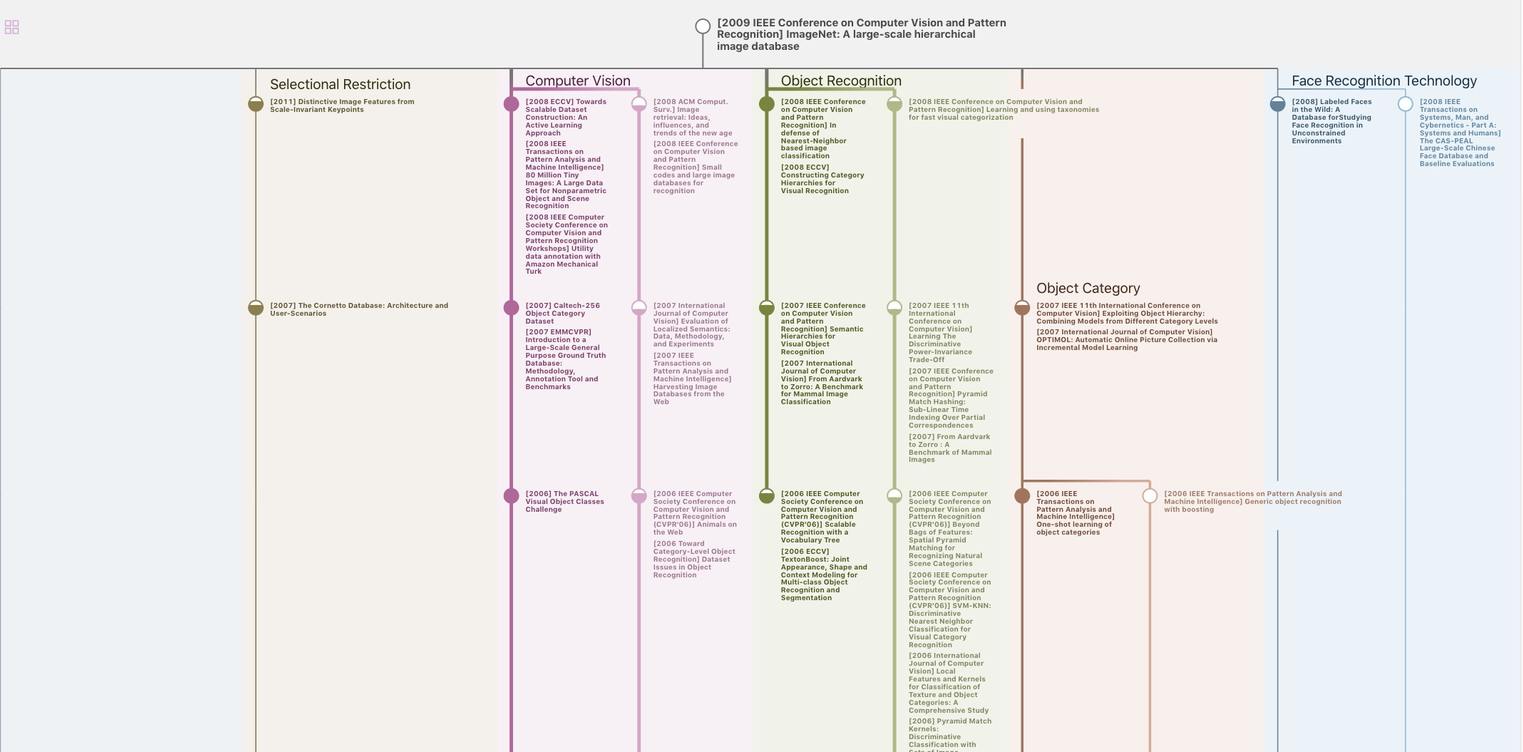
生成溯源树,研究论文发展脉络
Chat Paper
正在生成论文摘要