Transfer Learning Strategies for Lithium-Ion Battery Capacity Estimation under Domain Shift Differences
Journal of energy storage(2024)
摘要
Transfer learning is widely used for estimating the state of lithium-ion batteries, but its effectiveness is often hindered by domain shift. Focusing on the capacity estimation of lithium-ion batteries in transferable scenarios, this paper proposes a partition rule for the degree of domain shift that takes into account both the similarities and differences in lithium-ion battery material systems and charging strategies. An investigation was conducted on the optimal selection among four common transfer learning strategies under varying degrees of domain shift, utilizing three distinct lithium-ion battery datasets from different material systems. The results show that direct transfer learning and fine-tune-based transfer learning are suitable when the degree of domain shift is minimal. On the other hand, a fine-tuning strategy based on maximum mean discrepancy loss and a domain-adversarial neural network are recommended when the degree of domain shift is substantial. This research offers a methodological approach for capacity estimation of lithium-ion batteries in transferable scenarios.
更多查看译文
关键词
Lithium -ion batteries,Capacity estimation,Transfer learning strategies,Domain shift
AI 理解论文
溯源树
样例
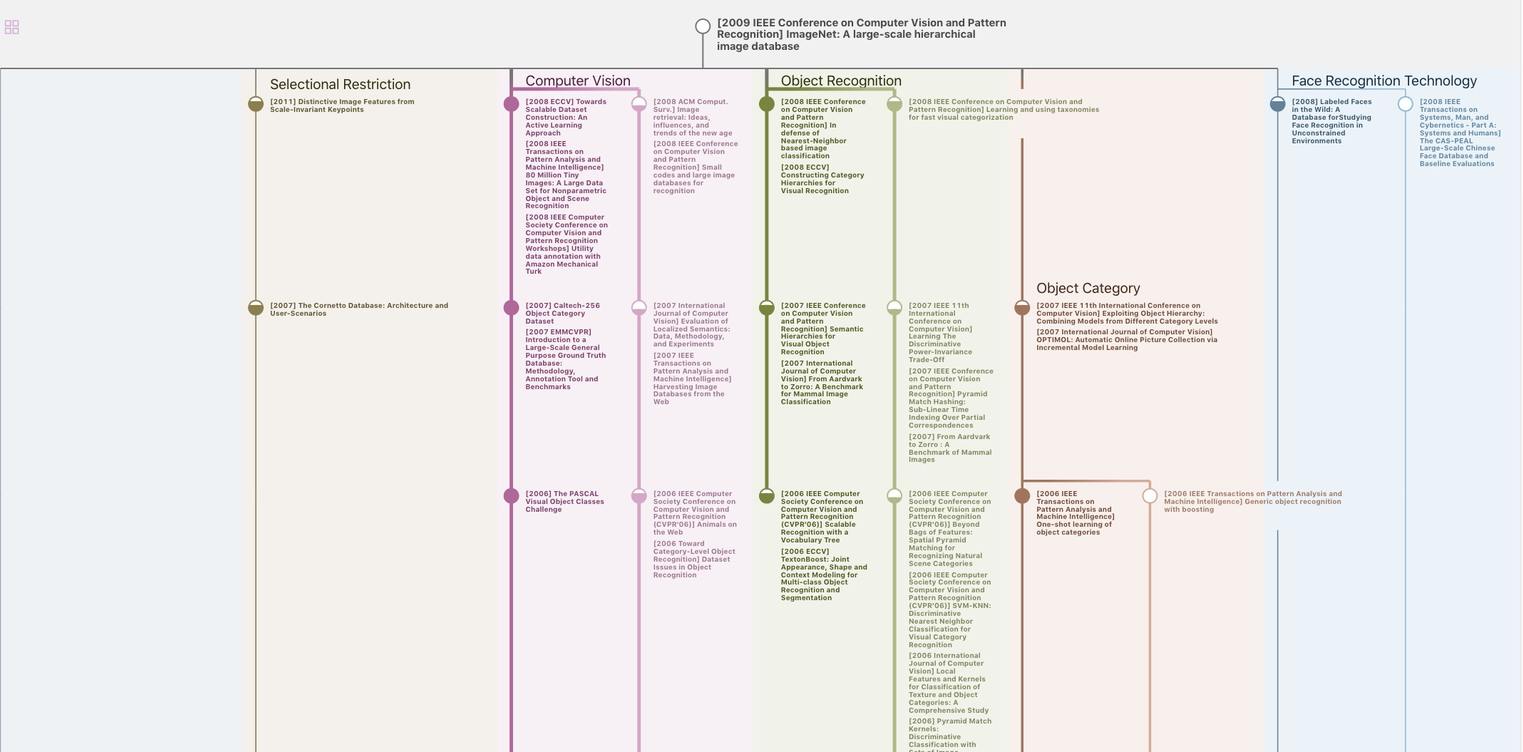
生成溯源树,研究论文发展脉络
Chat Paper
正在生成论文摘要