Data-driven forward-inverse problems of the 2-coupled mixed derivative nonlinear Schrödinger equation using deep learning
Nonlinear Dynamics(2024)
摘要
In recent years, generative adversarial networks(GAN) has achieved great success in generating realistic images. However, the instability of GAN and the lower accuracy of physics-informed neural networks(PINN) in solving highly complex partial differential equations make training models extremely challenging. This paper proposes a novel physics-informed GAN with gradient penalty (PIGAN-GP) and applies it to predict solutions of the 2-coupled mixed derivative nonlinear Schrödinger. The PIGAN-GP integrates PINN as part of the generator in the GAN framework, namely, utilizes PINN to solve the physical equation and generate predictions for the soliton positions and shapes. We predict the positions and shapes of nondegenerate solitons by the real and predicted solutions to demonstrate the high accuracy and stability of this PIGAN-GP network. Additionally, we also discuss the influence of noise levels and different initializations on the model parameter discovery using the PINN.
更多查看译文
关键词
Nondegenerate soliton,Physics-informed generative adversarial networks,2-coupled mixed derivative nonlinear Schrödinger equation
AI 理解论文
溯源树
样例
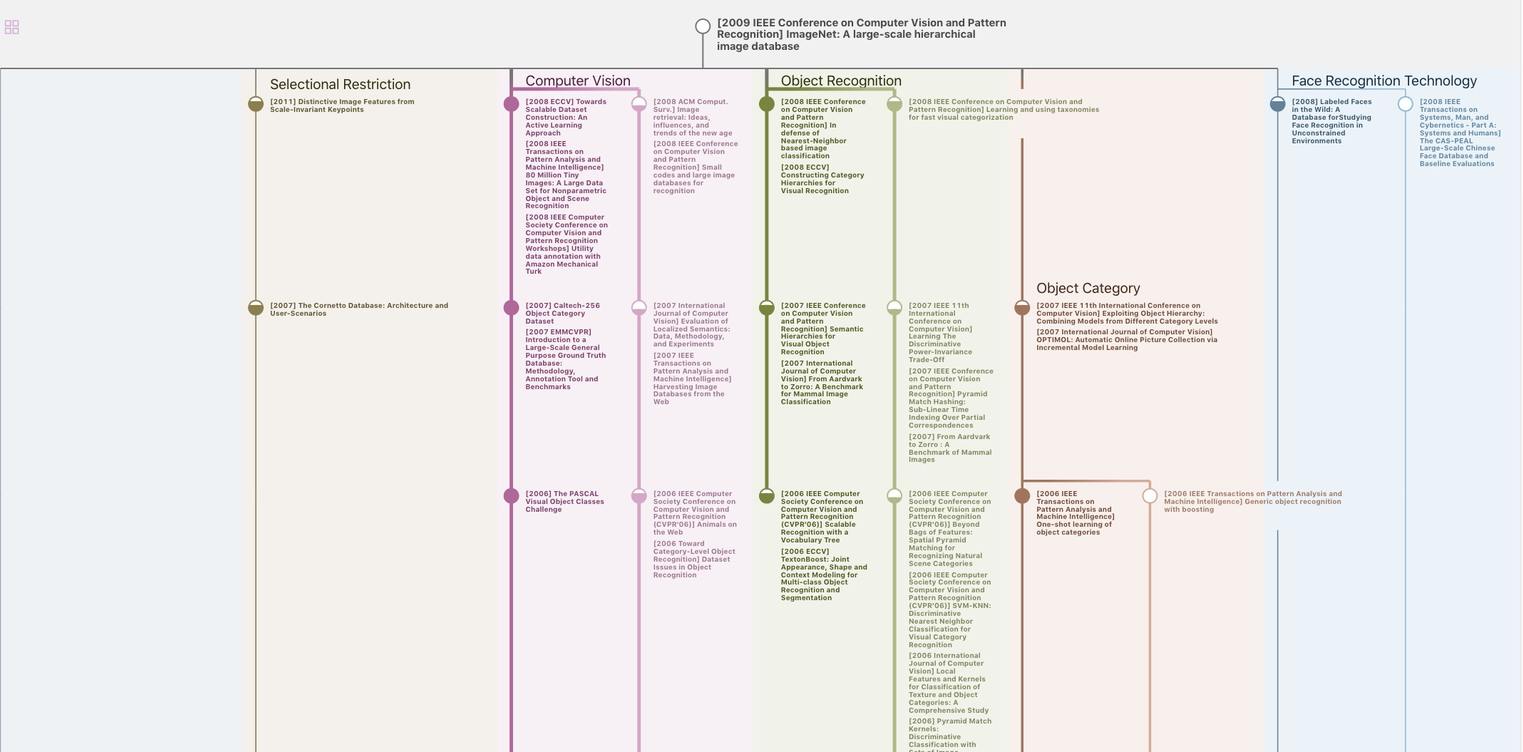
生成溯源树,研究论文发展脉络
Chat Paper
正在生成论文摘要