Unknown-class recognition adversarial network for open set domain adaptation fault diagnosis of rotating machinery
Journal of Intelligent Manufacturing(2024)
摘要
Transfer learning methods have received abundant attention and extensively utilized in cross-domain fault diagnosis, which suppose that the label sets in the source and target domains are coincident. However, the open set domain adaptation problem which include new fault modes in the target domain is not well solved. To address the problem, an unknown-class recognition adversarial network (UCRAN) is proposed for the cross-domain fault diagnosis. Specifically, a three-dimensional discriminator is designed to conduct domain-invariant learning on the source domain, target known domain and target unknown domain. Then, an entropy minimization is introduced to determine the decision boundaries. Finally, a posteriori inference method is developed to calculate the open set recognition weight, which are used to adaptively weigh the importance between known class and unknown class. The effectiveness and practicability of the proposed UCRAN is validated by a series of experiments. The experimental results show that compared to other existing methods, the proposed UCRAN realizes better diagnosis performance in different domain transfer task.
更多查看译文
关键词
Adversarial network,Open set domain adaptation,Unknown-class recognition,Fault diagnosis
AI 理解论文
溯源树
样例
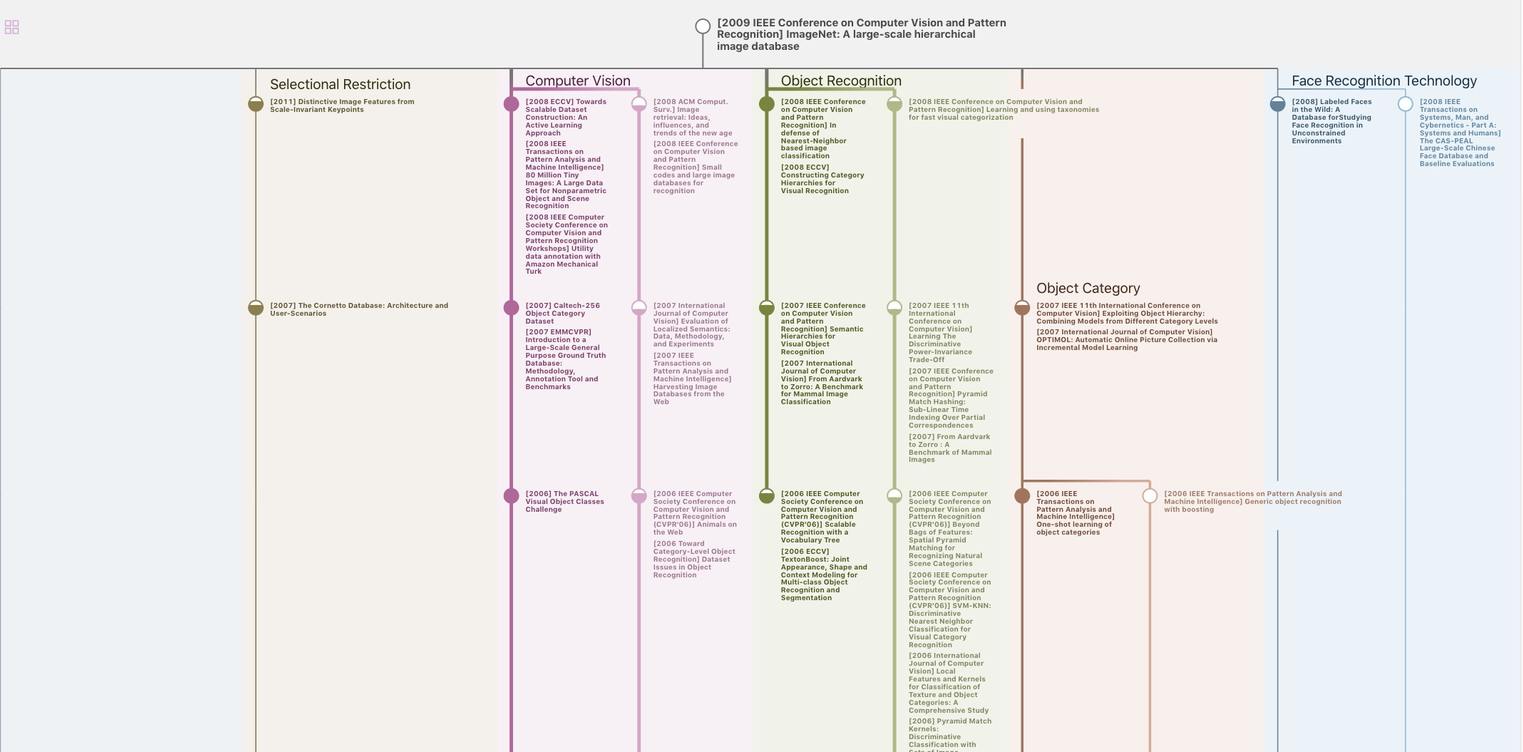
生成溯源树,研究论文发展脉络
Chat Paper
正在生成论文摘要