Evaluating an adaptive network model of human learning
Journal of Memory and Language(1988)
摘要
This paper explores the promise of simple adaptive networks as models of human learning. The least-mean-squares (LMS) learning rule of networks corresponds to the Rescorla-Wagner model of Pavlovian conditioning, suggesting interesting parallels in human and animal learning. We review three experiments in which subjects learned to classify patients according to symptoms which had differing correlations with two diseases. The LMS network model predicted the results of these experiments, comparing somewhat favorably with several competing learning models. We then extended the network model to deal with some attentional effects in human discrimination learning, wherein cue weight reflects attention to a cue. We further extended the model to include conjunctive features, enabling it to approximate classic results of the difficulty ordering of learning differing types of classifications. Despite the well-known limitations of one-layer network models, we nevertheless promote their use as benchmark models because of their explanatory power, simplicity, aesthetic grace, and approximation, in many circumstances, to multilayer network models. The successes of a simple model suggest greater accuracy of the LMS algorithm as against other learning rules, while its failures inform and constrain the class of more complex models needed to explain complex results.
更多查看译文
关键词
network model
AI 理解论文
溯源树
样例
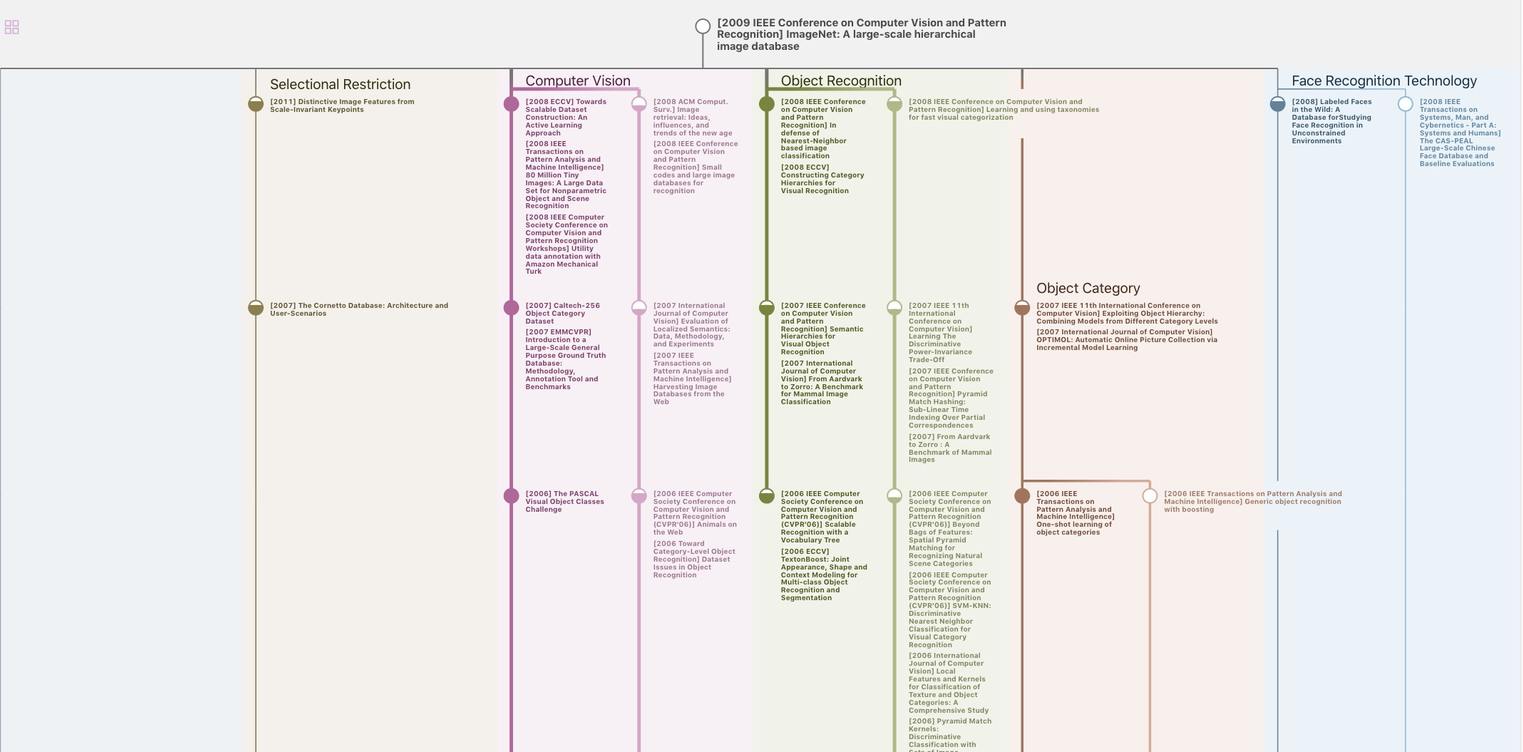
生成溯源树,研究论文发展脉络
Chat Paper
正在生成论文摘要