Intensive Longitudinal Assessment Following Index Trauma to Predict Development of PTSD using Machine Learning
Journal of Anxiety Disorders(2024)
摘要
There are significant challenges to identifying which individuals require intervention following exposure to trauma, and a need for strategies to identify and provide individuals at risk for developing PTSD with timely interventions. The present study seeks to identify a minimal set of trauma-related symptoms, assessed during the weeks following traumatic exposure, that can accurately predict PTSD. Participants were 2,185 adults (Mean age=36.4 years; 64% women; 50% Black) presenting for emergency care following traumatic exposure. Participants received a ‘flash survey’ with 6-8 varying symptoms (from a pool of 26 trauma symptoms) several times per week for eight weeks following the trauma exposure (each symptom assessed ~6 times). Features (mean, sd, last, worst, peak-end scores) from the repeatedly assessed symptoms were included as candidate variables in a CART machine learning analysis to develop a pragmatic predictive algorithm. PTSD (PCL-5≥38) was present for 669 (31%) participants at the 8-week follow-up. A classification tree with three splits, based on mean scores of nervousness, rehashing, and fatigue, predicted PTSD with an Area Under the Curve of 0.836. Findings suggest feasibility for a 3-item assessment protocol, delivered once per week, following traumatic exposure to assess and potentially facilitate follow-up care for those at risk.
更多查看译文
关键词
Posttraumatic Stress Disorder,Machine Learning,Mobile Assessment,Emergency Services,Trauma
AI 理解论文
溯源树
样例
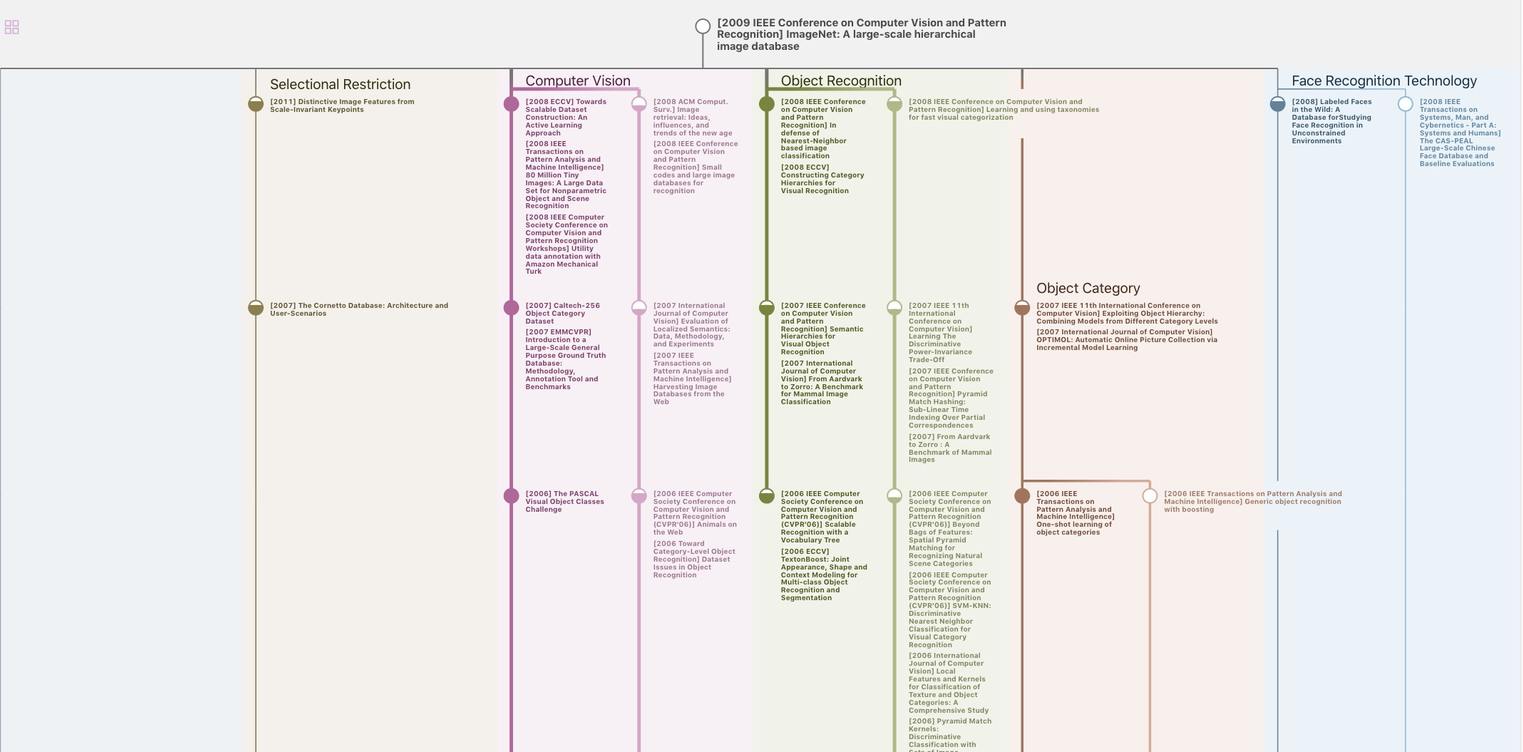
生成溯源树,研究论文发展脉络
Chat Paper
正在生成论文摘要