CABnet: A channel attention dual adversarial balancing network for multimodal image fusion
Image and Vision Computing(2024)
摘要
Infrared and visible image fusion aims to generate informative images by leveraging the distinctive strengths of infrared and visible modalities. These fused images play a crucial role in subsequent downstream tasks, including object detection, recognition, and segmentation. However, complementary information is often difficult to extract. Existing generative adversarial network-based methods generate fused images by modifying the distribution of source images' features to preserve instances and texture details in both infrared and visible images. Nevertheless, these approaches may result in a degradation of the fused image quality when the original image quality is low. Considering the balance of information from different modalities can improve the quality of the fused image. Hence, we introduce CABnet, a Channel Attention dual adversarial Balancing network. CABnet incorporates a channel attention mechanism to capture crucial channel features, thereby, enhancing complementary information. It also employs an adaptive factor to control the mixing distribution of infrared and visible images, which ensures the preservation of instances and texture details during the adversarial process. To enhance efficiency and reduce reliance on manual labeling, our training process adopts a semi-supervised learning strategy. Through qualitative and quantitative experiments across multiple datasets, CABnet surpasses existing state-of-the-art methods in fusion performance, notably achieving a 51.3% enhancement in signal to noise ratio and a 13.4% improvement in standard deviation.
更多查看译文
关键词
Image processing,Infrared and visible image fusion,Complementary information extract,Generative adversarial networks,Adaptive factor
AI 理解论文
溯源树
样例
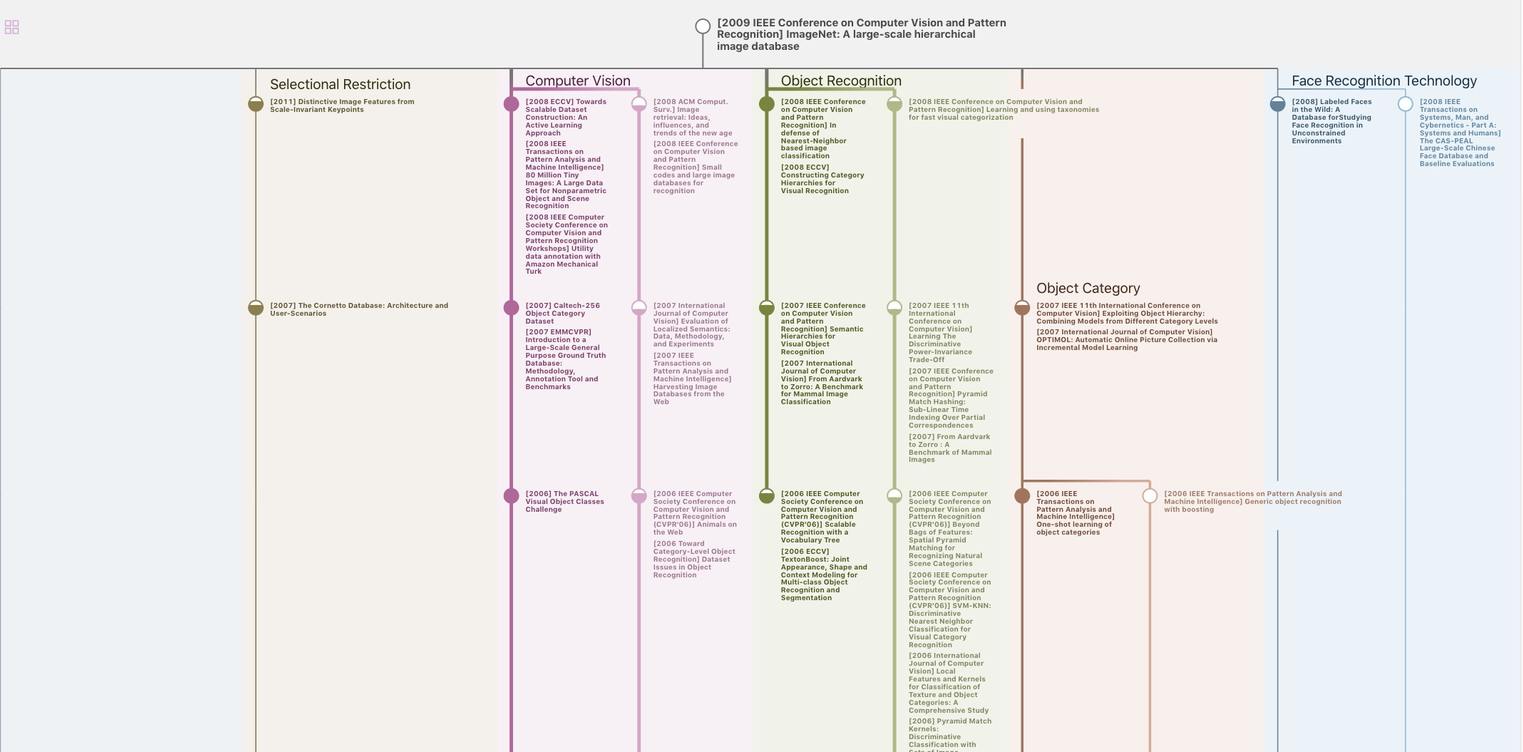
生成溯源树,研究论文发展脉络
Chat Paper
正在生成论文摘要