Neuromorphic Computing Spiking Neural Network Edge Detection Model for Content Based Image Retrieval.
Network(2024)
摘要
In contemporary times, content-based image retrieval (CBIR) techniques have gained widespread acceptance as a means for end-users to discern and extract specific image content from vast repositories. However, it is noteworthy that a substantial majority of CBIR studies continue to rely on linear methodologies such as gradient-based and derivative-based edge detection techniques. This research explores the integration of bioinspired Spiking Neural Network (SNN) based edge detection within CBIR. We introduce an innovative, computationally efficient SNN-based approach designed explicitly for CBIR applications, outperforming existing SNN models by reducing computational overhead by 2.5 times. The proposed SNN-based edge detection approach is seamlessly incorporated into three distinct CBIR techniques, each employing conventional edge detection methodologies including Sobel, Canny, and image derivatives. Rigorous experimentation and evaluations are carried out utilizing the Corel-10k dataset and crop weed dataset, a widely recognized and frequently adopted benchmark dataset in the realm of image analysis. Importantly, our findings underscore the enhanced performance of CBIR methodologies integrating the proposed SNN-based edge detection approach, with an average increase in mean precision values exceeding 3%. This study conclusively demonstrated the utility of our proposed methodology in optimizing feature extraction, thereby establishing its pivotal role in advancing edge centric CBIR approaches.
更多查看译文
关键词
Content-based image retrieval (CBIR),edge detection,human visual system (HVS),spiking neural network (SNN),feature extraction,image analysis
AI 理解论文
溯源树
样例
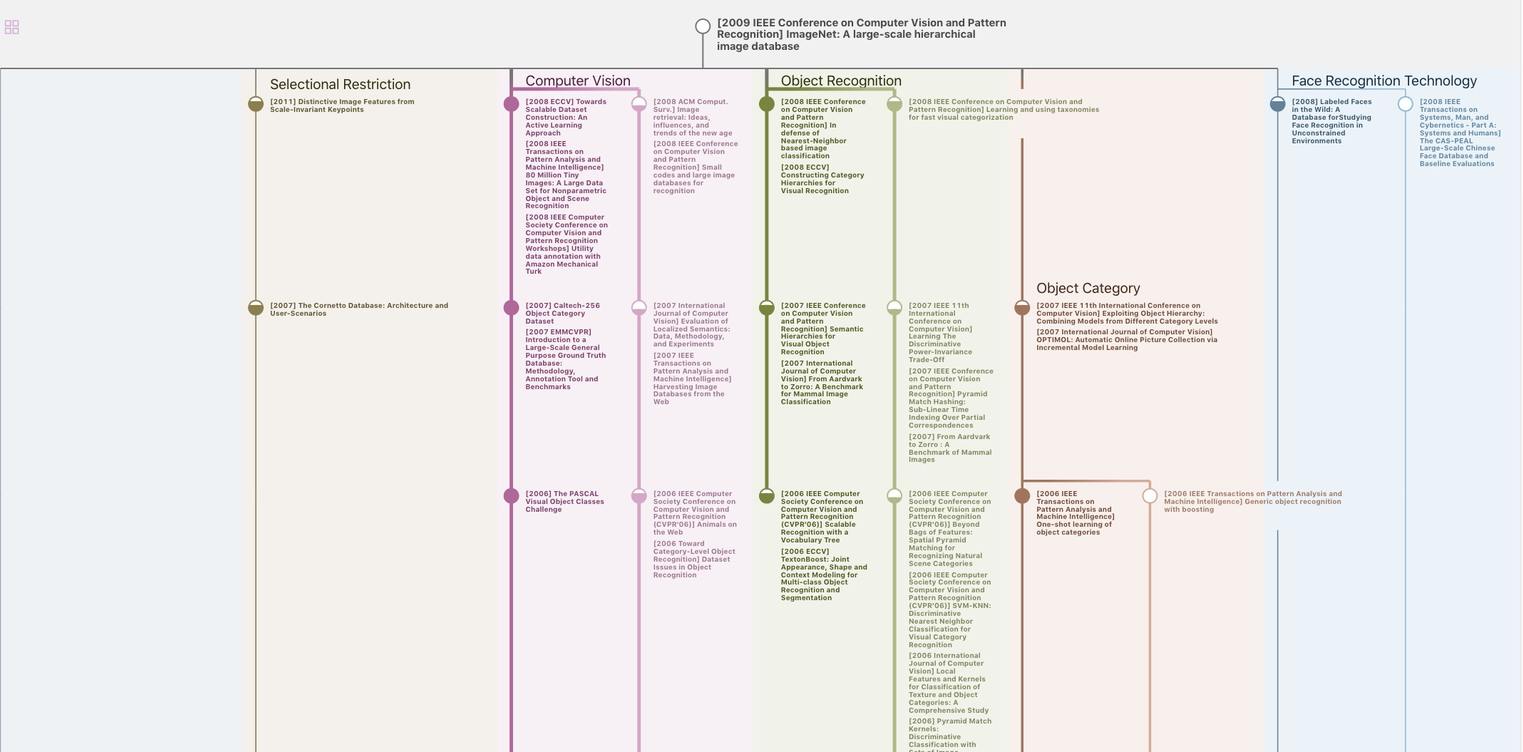
生成溯源树,研究论文发展脉络
Chat Paper
正在生成论文摘要