Robust image-based cross-sectional grain boundary detection and characterization using machine learning
Journal of Intelligent Manufacturing(2024)
摘要
Understanding the anisotropic sintering behavior of 3D-printed materials requires massive analytic studies on their grain boundary (GB) structures. Accurate characterization of the GBs is critical to study the metallurgical process. However, it is challenging and time-consuming for sintered 3D-printed materials due to immature etching and residual pores. In this study, we developed a machine learning-based method of characterizing GBs of sintered 3D-printed materials. The developed method is also generalizable and robust enough to characterize GBs from other non-3D-printed materials. This method can be applied to a small dataset because it includes a diffusion network that generate augmented images for training. The study compared various machine learning methods commonly used for segmentation, which include UNet, ResNeXt, and Ensemble of UNets. The comparison results showed that the Ensemble of UNets outperformed the other methods for the GB detection and characterization. The model is tested on unclear GBs from sintered 3D-printed samples processed with non-optimized etching and classifies the GBs with around 90
更多查看译文
关键词
Additive manufacturing,Binder jetting,Grain boundary,Porosity,Sintering,Machine learning,Materials characterization
AI 理解论文
溯源树
样例
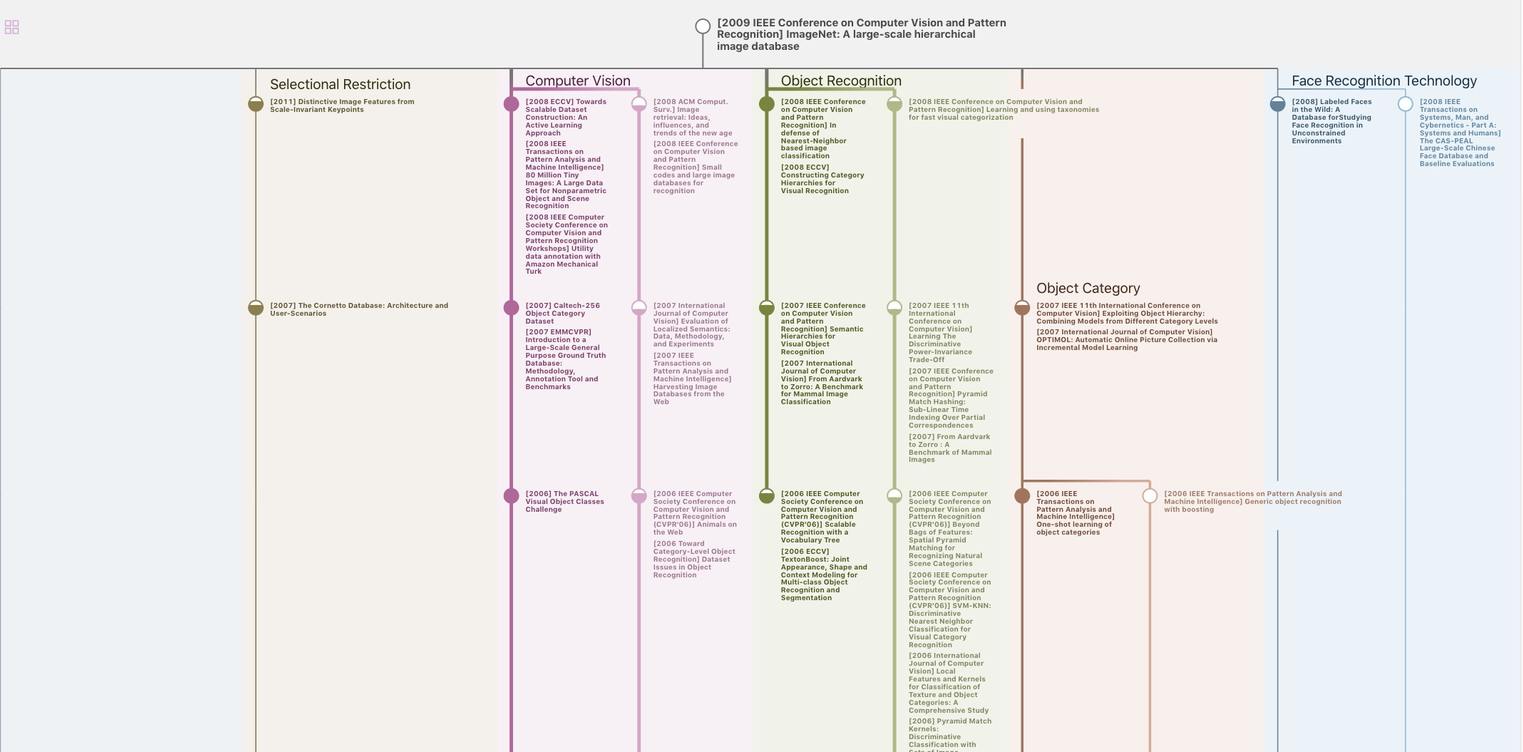
生成溯源树,研究论文发展脉络
Chat Paper
正在生成论文摘要