Nip in the Bud: Forecasting and Interpreting Post-exploitation Attacks in Real-time through Cyber Threat Intelligence Reports
arxiv(2024)
摘要
Advanced Persistent Threat (APT) attacks have caused significant damage
worldwide. Various Endpoint Detection and Response (EDR) systems are deployed
by enterprises to fight against potential threats. However, EDR suffers from
high false positives. In order not to affect normal operations, analysts need
to investigate and filter detection results before taking countermeasures, in
which heavy manual labor and alarm fatigue cause analysts miss optimal response
time, thereby leading to information leakage and destruction. Therefore, we
propose Endpoint Forecasting and Interpreting (EFI), a real-time attack
forecast and interpretation system, which can automatically predict next move
during post-exploitation and explain it in technique-level, then dispatch
strategies to EDR for advance reinforcement. First, we use Cyber Threat
Intelligence (CTI) reports to extract the attack scene graph (ASG) that can be
mapped to low-level system logs to strengthen attack samples. Second, we build
a serialized graph forecast model, which is combined with the attack provenance
graph (APG) provided by EDR to generate an attack forecast graph (AFG) to
predict the next move. Finally, we utilize the attack template graph (ATG) and
graph alignment plus algorithm for technique-level interpretation to
automatically dispatch strategies for EDR to reinforce system in advance. EFI
can avoid the impact of existing EDR false positives, and can reduce the attack
surface of system without affecting the normal operations. We collect a total
of 3,484 CTI reports, generate 1,429 ASGs, label 8,000 sentences, tag 10,451
entities, and construct 256 ATGs. Experimental results on both DARPA Engagement
and large scale CTI dataset show that the alignment score between the AFG
predicted by EFI and the real attack graph is able to exceed 0.8, the forecast
and interpretation precision of EFI can reach 91.8
更多查看译文
AI 理解论文
溯源树
样例
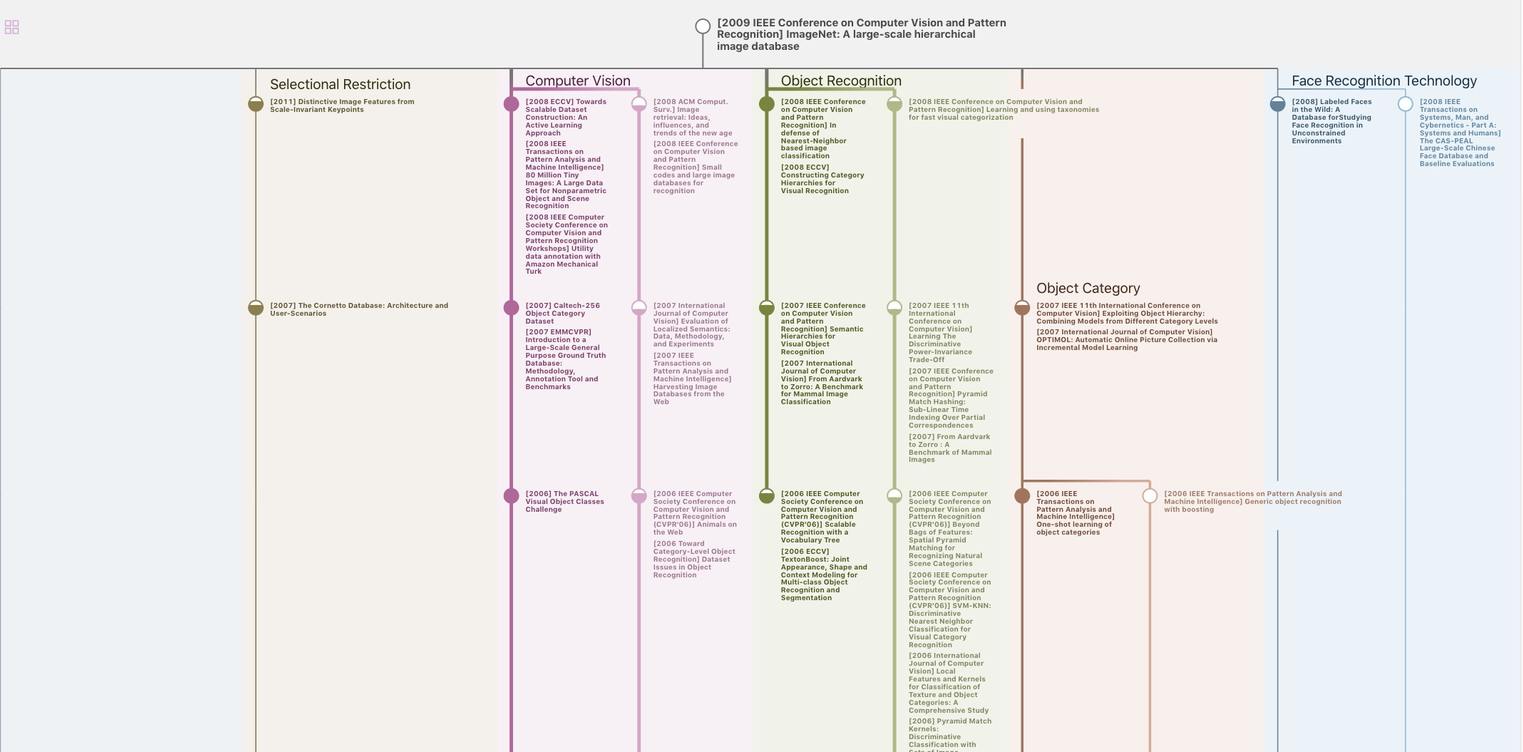
生成溯源树,研究论文发展脉络
Chat Paper
正在生成论文摘要