End-to-End Reinforcement Learning of Curative Curtailment with Partial Measurement Availability
CoRR(2024)
摘要
In the course of the energy transition, the expansion of generation and
consumption will change, and many of these technologies, such as PV systems,
electric cars and heat pumps, will influence the power flow, especially in the
distribution grids. Scalable methods that can make decisions for each grid
connection are needed to enable congestion-free grid operation in the
distribution grids. This paper presents a novel end-to-end approach to
resolving congestion in distribution grids with deep reinforcement learning.
Our architecture learns to curtail power and set appropriate reactive power to
determine a non-congested and, thus, feasible grid state. State-of-the-art
methods such as the optimal power flow (OPF) demand high computational costs
and detailed measurements of every bus in a grid. In contrast, the presented
method enables decisions under sparse information with just some buses
observable in the grid. Distribution grids are generally not yet fully
digitized and observable, so this method can be used for decision-making on the
majority of low-voltage grids. On a real low-voltage grid the approach resolves
100% of violations in the voltage band and 98.8% of asset overloads. The
results show that decisions can also be made on real grids that guarantee
sufficient quality for congestion-free grid operation.
更多查看译文
AI 理解论文
溯源树
样例
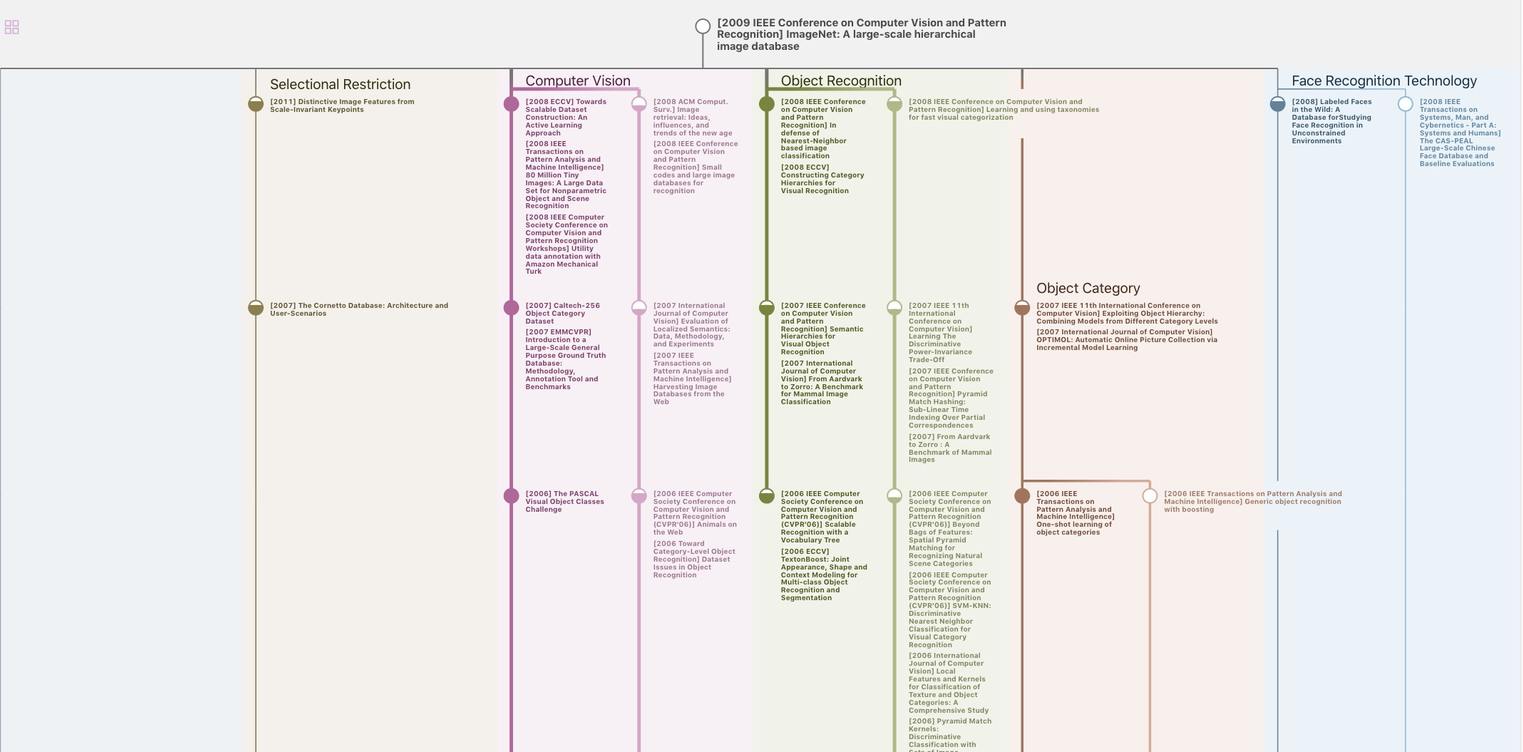
生成溯源树,研究论文发展脉络
Chat Paper
正在生成论文摘要