Annot-Mix: Learning with Noisy Class Labels from Multiple Annotators via a Mixup Extension
arxiv(2024)
摘要
Training with noisy class labels impairs neural networks' generalization
performance. In this context, mixup is a popular regularization technique to
improve training robustness by making memorizing false class labels more
difficult. However, mixup neglects that, typically, multiple annotators, e.g.,
crowdworkers, provide class labels. Therefore, we propose an extension of
mixup, which handles multiple class labels per instance while considering which
class label originates from which annotator. Integrated into our
multi-annotator classification framework annot-mix, it performs superiorly to
eight state-of-the-art approaches on eleven datasets with noisy class labels
provided either by human or simulated annotators. Our code is publicly
available through our repository at https://github.com/ies-research/annot-mix.
更多查看译文
AI 理解论文
溯源树
样例
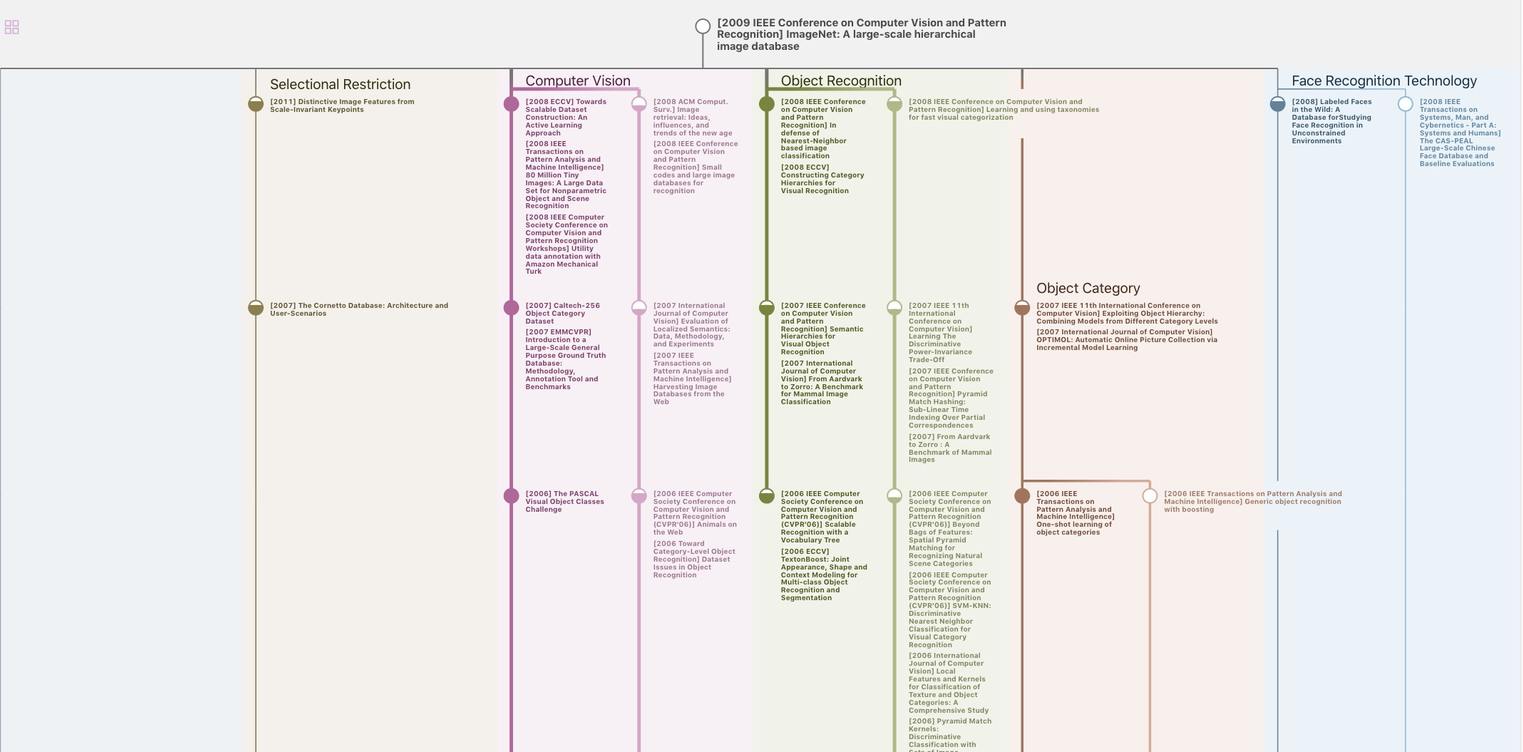
生成溯源树,研究论文发展脉络
Chat Paper
正在生成论文摘要