Deep Unrolled Single Snapshot Phase Retrieval via Non-Convex Formulation and Phase Mask Design
IEEE Journal of Selected Topics in Signal Processing(2024)
摘要
Phase retrieval (PR) consists of recovering the phase information from captured intensity measurements, known as coded diffraction patterns (CDPs). Non-convex algorithms for addressing the PR problem require a proper initialization that is refined through a gradient descent approach. These PR algorithms have proven to be robust for different scenarios. Despite deep models showing surprising results in this area, these approaches lack interpretability in their neural architectures. This work proposes unrolling the initialization and iterative reconstruction algorithm for the PR problem using the near-field model based on a non-convex formulation; resulting in an interpretable deep neural network (DNN) that can be trained in an end-to-end (E2E) manner. Furthermore, the proposed method can jointly optimize the phase mask for the CDP acquisition and the DNN parameters. Simulation results demonstrate that the proposed E2E method provides high-quality reconstruction using a learned phase mask from a single projection. Also, the proposed method is tested over an experimental optical setup that incorporates the learned phase mask via an only-phase spatial light modulator.
更多查看译文
关键词
Coded diffraction patterns,end-to-end optimization,phase mask,phase retrieval,unrolled networks,and deep learning
AI 理解论文
溯源树
样例
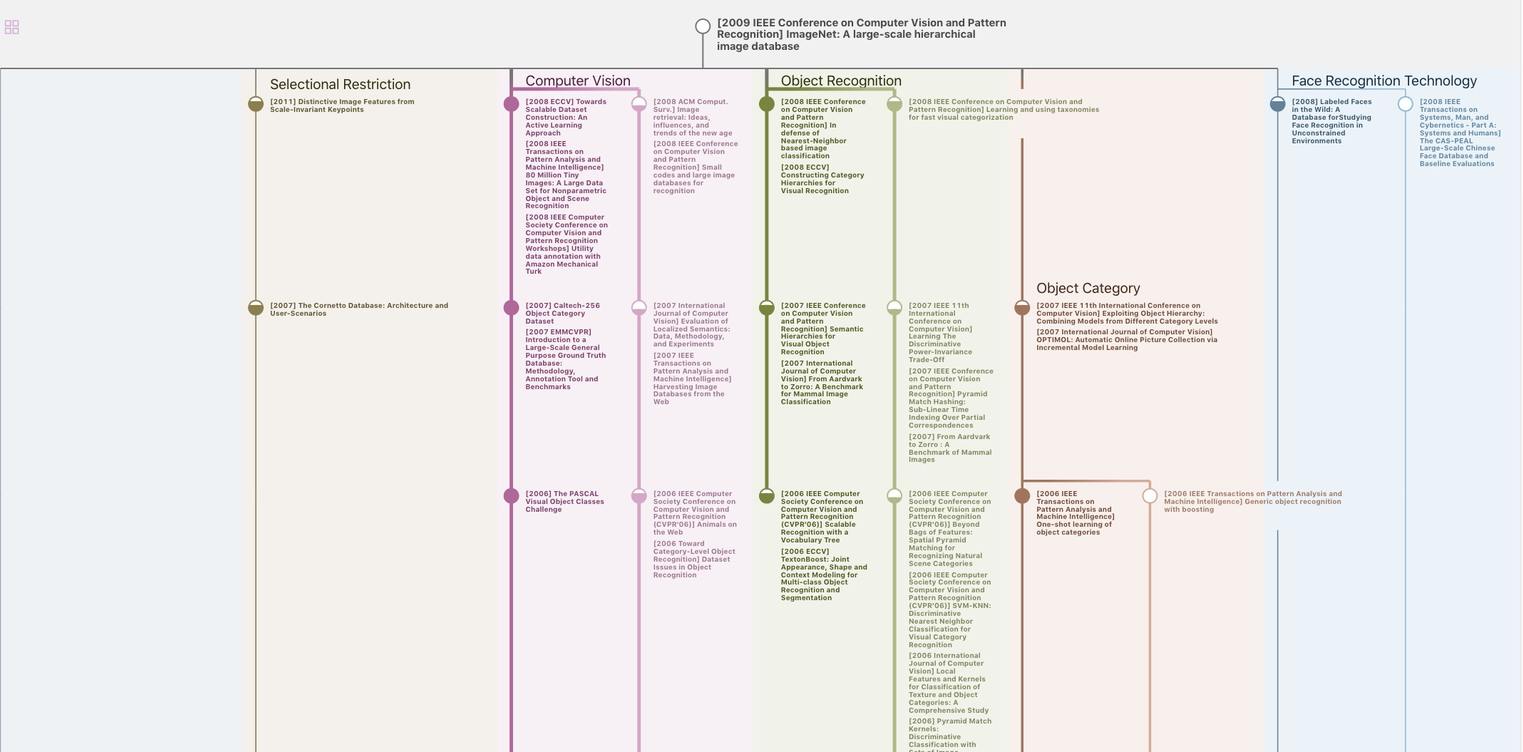
生成溯源树,研究论文发展脉络
Chat Paper
正在生成论文摘要