Transfer Learning via Random Forests: A One-Shot Federated Approach
Computational Statistics & Data Analysis(2024)
摘要
A one-shot federated transfer learning method using random forests (FTRF) is developed to improve the prediction accuracy at a target data site by leveraging information from auxiliary sites. Both theoretical and numerical results show that the proposed federated transfer learning approach is at least as accurate as the model trained on the target data alone regardless of possible data heterogeneity, which includes imbalanced and non-IID data distributions across sites and model mis-specification. FTRF has the ability to evaluate the similarity between the target and auxiliary sites, enabling the target site to autonomously select more similar site information to enhance its predictive performance. To ensure communication efficiency, FTRF adopts the model averaging idea that requires a single round of communication between the target and the auxiliary sites. Only fitted models from auxiliary sites are sent to the target site. Unlike traditional model averaging, FTRF incorporates predicted outcomes from other sites and the original variables when estimating model averaging weights, resulting in a variable-dependent weighting to better utilize models from auxiliary sites to improve prediction. Five real-world data examples show that FTRF reduces the prediction error by 2-40% compared to methods not utilizing auxiliary information.
更多查看译文
关键词
Adaptive weighting,Federated learning,Model averaging,Stacking ensemble,Transfer learning
AI 理解论文
溯源树
样例
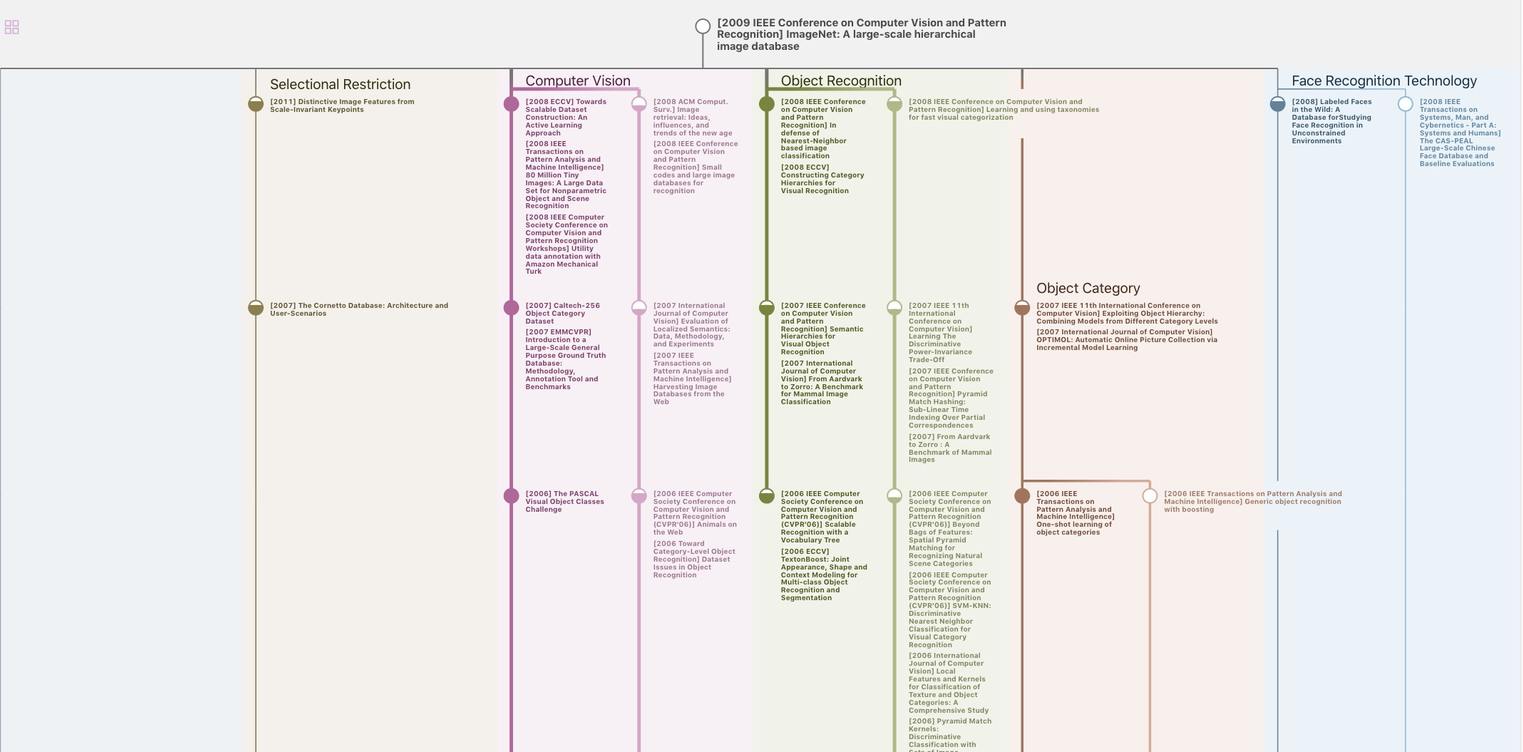
生成溯源树,研究论文发展脉络
Chat Paper
正在生成论文摘要