Adaptive and Interactive Multi-Level Spatio-Temporal Network for Traffic Forecasting
IEEE Transactions on Intelligent Transportation Systems(2024)
摘要
Traffic forecasting is a challenging research topic due to the complex spatial and temporal dependencies among different roads. Though great efforts have been made on traffic forecasting, existing works still have the following shortcomings: i) Most methods only directly perform on the original road network topology which cannot accommodate the diverse traffic patterns and multi-granularity traffic forecasting requirements driven by the natural multi-level urban structure and layout, ii) The existing studies based on the spatio-temporal multi-granularity perspective ignore the interactions between the fine-grained information and coarse-grained information, resulting in the spatio-temporal correlation under multi-granularity inaccurately modeled. To solve the problems, we propose an Adaptive and Interactive Multi-level Spatio-Temporal network (AIMST) for traffic forecasting. Specifically, we first devise a learnable adaptive hierarchical clustering method to automatically generate more coarse-grained graphs from the initial road networks and the traffic data. Then, the spatio-temporal graph convolutional networks are executed on the constructed hierarchical traffic graph of each level correspondingly to capture the spatio-temporal patterns. Furthermore, a multi-level bidirectional interaction module is designed to emphasize the multi-grained interaction patterns among different levels. Extensive experiments on two real-world traffic datasets demonstrate that our framework is superior to several state-of-the-art baselines.
更多查看译文
关键词
Spatio-temporal data mining,traffic forecasting,multi-level traffic network,urban computing
AI 理解论文
溯源树
样例
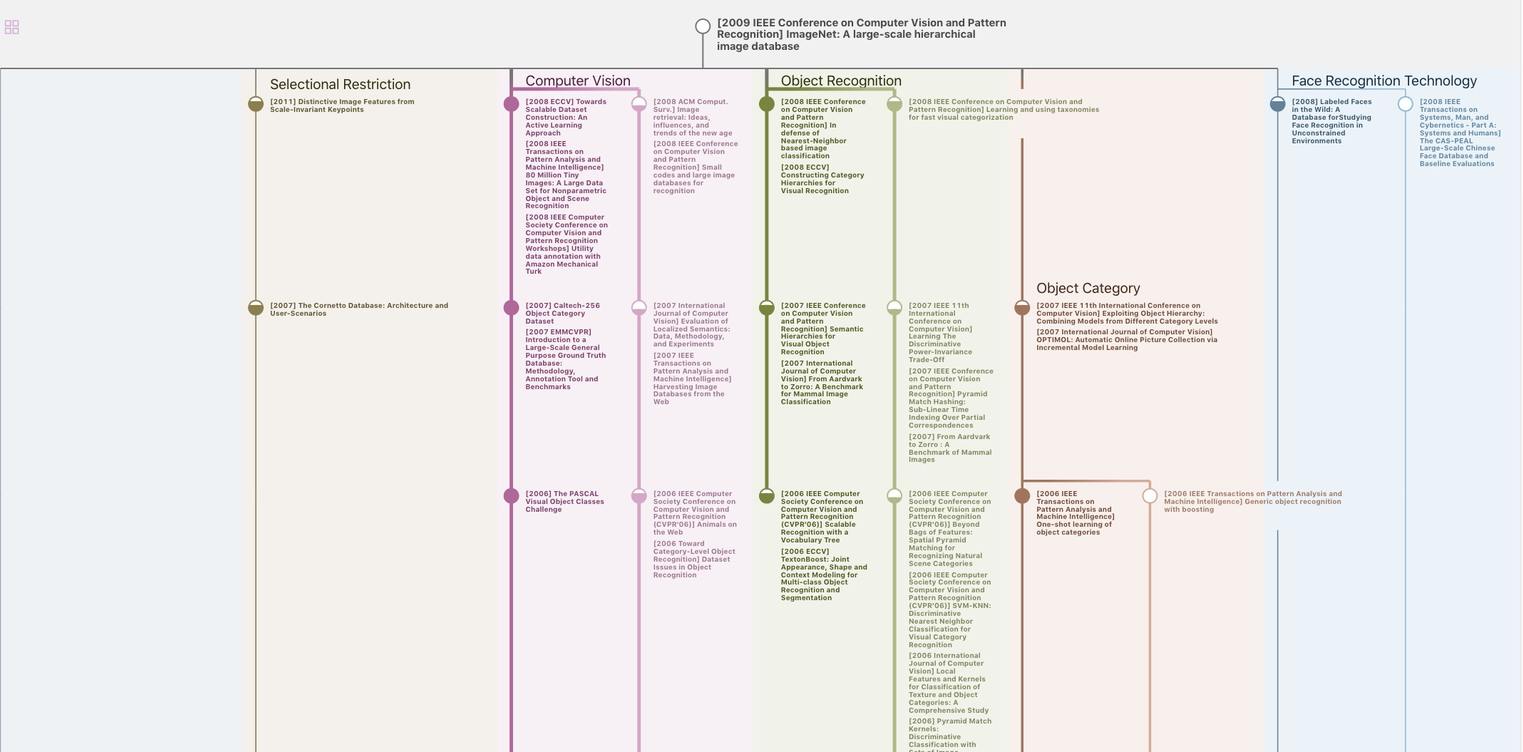
生成溯源树,研究论文发展脉络
Chat Paper
正在生成论文摘要