Machine learning for predicting halogen radical reactivity toward aqueous organic chemicals
Journal of Hazardous Materials(2024)
摘要
The rapid development of machine learning (ML) provides fast, accurate, and widely applicable methods for predicting the fate of micropollutants in water treatment processes. In this work, we developed a series of ML models for four different halogen radicals using Morgan fingerprint (MF) and Mordred descriptor (MD) to predict their secondary rate constants (k). The findings highlight that making accurate predictions for various datasets depended on an effective combination of descriptors and algorithms. To further alleviate the challenge of limited sample size, we introduced a data combination strategy that improved prediction accuracy and mitigated overfitting by combining different datasets. The LightGBM with MF (MF-LightGBM) and RF with MD (MD-RF) models based on the unified dataset were finally selected as the optimal models. Shapley additive interpretation was used to explain the models: the MF-LightGBM model successfully captured the influence of electron-withdrawing/donating groups, while autocorrelation, walk count and information content descriptors in the MD-RF model were identified as key features. Furthermore, the important contribution of pH was emphasized. The results of the applicability domain analysis further supported that the developed model can make reliable predictions for query compounds across a broader range. Finally, a practical web application for k calculations was built.
Environmental implication
Hundreds of thousands of chemicals are annually introduced to the global market, posing potential risks to organisms and the environment through their by-products. Assessing the degradation behavior of pollutants is crucial, and reaction rate constants serve as key indicators for this evaluation. This study aims to predict the secondary rate constants of four halogen radicals reacting with aqueous phase pollutants. The prediction is achieved by using molecular fingerprinting, molecular descriptor in combination with machine learning algorithms. Accurate predicting results contribute to fostering positive environmental impacts within the realms of wastewater treatment and water purification.
更多查看译文
关键词
Halogen radical rate constants,Morgan fingerprint,Mordred descriptor,Machine learning,Web application
AI 理解论文
溯源树
样例
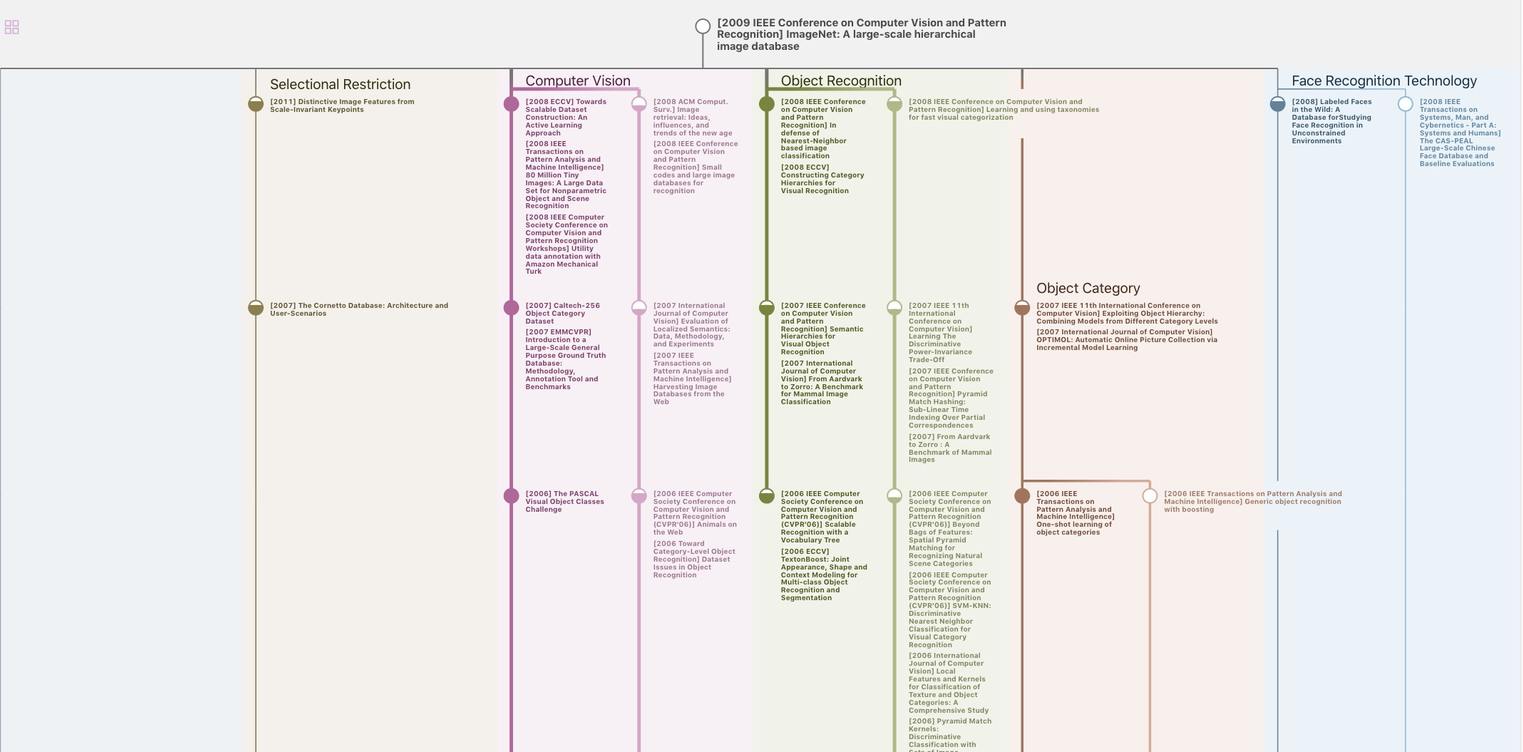
生成溯源树,研究论文发展脉络
Chat Paper
正在生成论文摘要