Transformers for Generalized Fast Shower Simulation
26TH INTERNATIONAL CONFERENCE ON COMPUTING IN HIGH ENERGY AND NUCLEAR PHYSICS, CHEP 2023(2024)
摘要
Recently, transformer-based foundation models have proven to be a generalized architecture applicable to various data modalities, ranging from text to audio and even a combination of multiple modalities. Transformers by design should accurately model the non-trivial structure of particle showers thanks to the absence of strong inductive bias, better modeling of long-range dependencies, and interpolation and extrapolation capabilities. In this paper, we explore a transformer-based generative model for detector-agnostic fast shower simulation, where the goal is to generate synthetic particle showers, i.e., the energy depositions in the calorimeter. When trained with an adequate amount and variety of showers, these models should learn better representations compared to other deep learning models, and hence should quickly adapt to new detectors. In this work, we will show the prototype of a transformer-based generative model for fast shower simulation, as well as explore certain aspects of transformer architecture such as input data representation, sequence formation, and the learning mechanism for our unconventional shower data.
更多查看译文
AI 理解论文
溯源树
样例
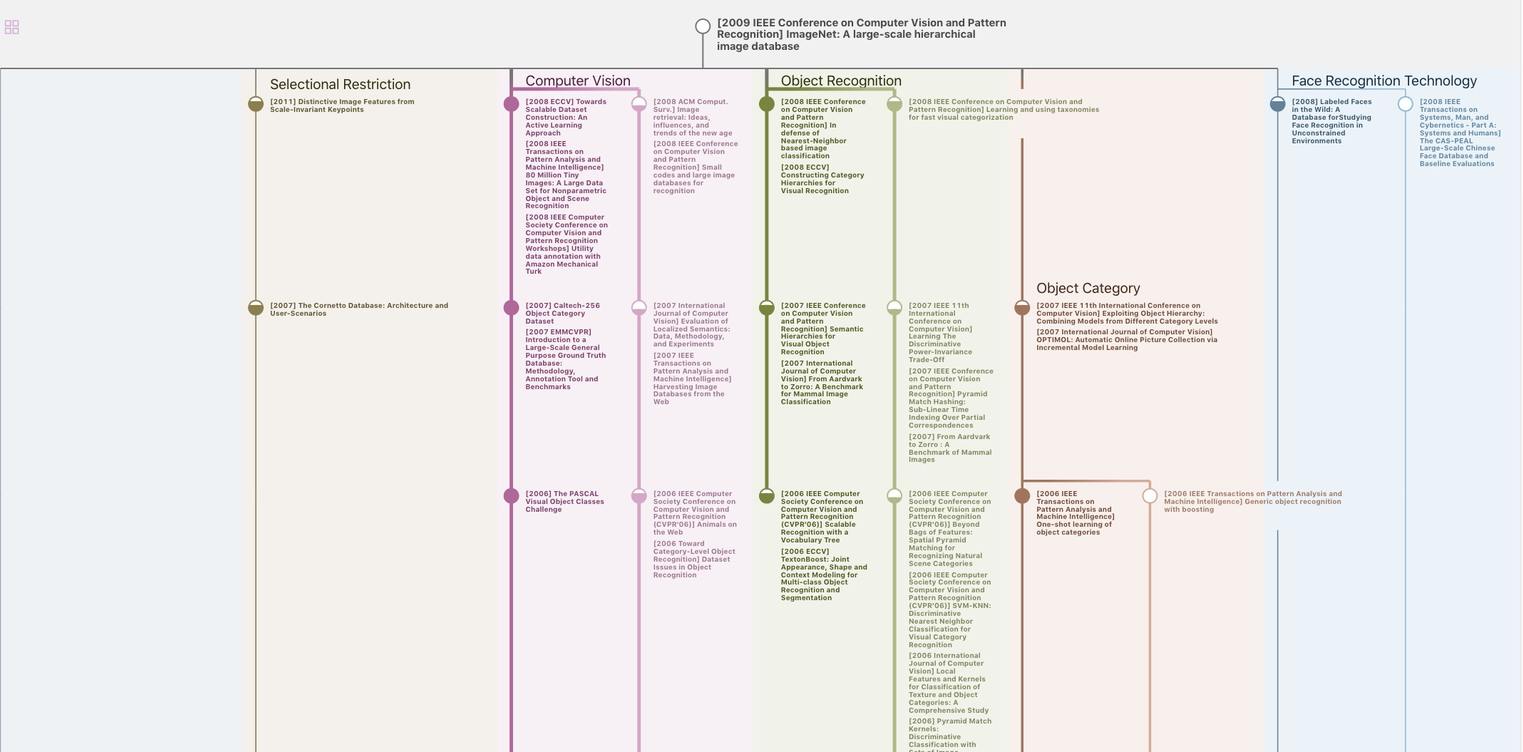
生成溯源树,研究论文发展脉络
Chat Paper
正在生成论文摘要