D3C2-Net: Dual-Domain Deep Convolutional Coding Network for Compressive Sensing
IEEE Transactions on Circuits and Systems for Video Technology(2024)
摘要
By mapping iterative optimization algorithms into neural networks (NNs), deep unfolding networks (DUNs) exhibit well-defined and interpretable structures and achieve remarkable success in the field of compressive sensing (CS). However, most existing DUNs solely rely on the image-domain unfolding, which restricts the information transmission capacity and reconstruction flexibility, leading to their loss of image details and unsatisfactory performance. To overcome these limitations, this paper develops a dual-domain optimization framework that combines the priors of (1) image- and (2) convolutional-coding-domains and offers generality to CS and other inverse imaging tasks. By converting this optimization framework into deep NN structures, we present a Dual-Domain Deep Convolutional Coding Network (D
3
C
2
-Net), which enjoys the ability to efficiently transmit high-capacity self-adaptive convolutional features across all its unfolded stages. Our theoretical analyses and experiments on simulated and real captured data, covering 2D and 3D natural, medical, and scientific signals, demonstrate the effectiveness, practicality, superior performance, and generalization ability of our method over other competing approaches and its significant potential in achieving a balance among accuracy, complexity, and interpretability. Code is available at https://github.com/lwq20020127/D3C2-Net.
更多查看译文
关键词
Compressive sensing,inverse imaging problem,image restoration,convolutional coding,deep unfolding network
AI 理解论文
溯源树
样例
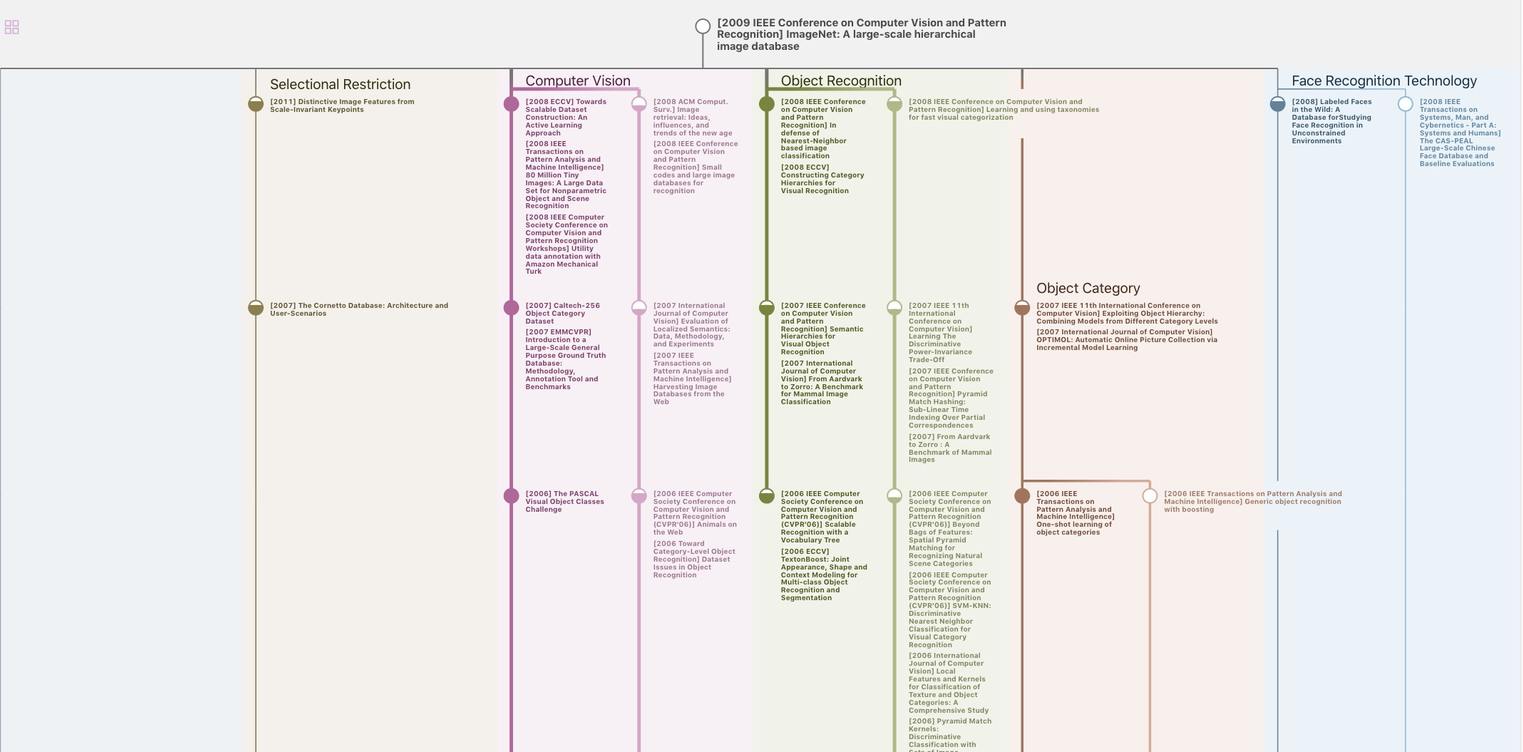
生成溯源树,研究论文发展脉络
Chat Paper
正在生成论文摘要