Predictive capability of rough set machine learning in tetracycline adsorption using biochar
Carbon Research(2024)
摘要
Machine learning algorithms investigate relationships in data to deliver useful outputs. However, past models required complete datasets as a prerequisite. In this study, rough set-based machine learning was applied using real-world incomplete datasets to generate a prediction model of biochar’s adsorption capacity based on key attributes. The predictive model consists of if–then rules classifying properties by fulfilling certain conditions. The rules generated from both complete and incomplete datasets exhibit high certainty and coverage, along with scientific coherence. Based on the complete dataset model, optimal pyrolysis conditions, biomass characteristics and adsorption conditions were identified to maximize tetracycline adsorption capacity (> 200 mg/g) by biochar. This study demonstrates the capabilities of rough set-based machine learning using incomplete practical real-world data without compromising key features. The approach can generate valid predictive models even with missing values in datasets. Overall, the preliminary results show promise for applying rough set machine learning to real-world, incomplete data for generating biomass and biochar predictive models. However, further refinement and testing are warranted before practical implementation. • It is the first explainable AI-based rough set model to study the tetracycline adsorption capacity of biochar. • Usage of an incomplete Practical dataset through RSML evaded the biasness due to imputations. • Higher accuracy and precision of incomplete Practical datasets revealed the uniqueness of the model.
更多查看译文
关键词
Tetracycline,Adsorption,Rough set,Machine learning,Biochar
AI 理解论文
溯源树
样例
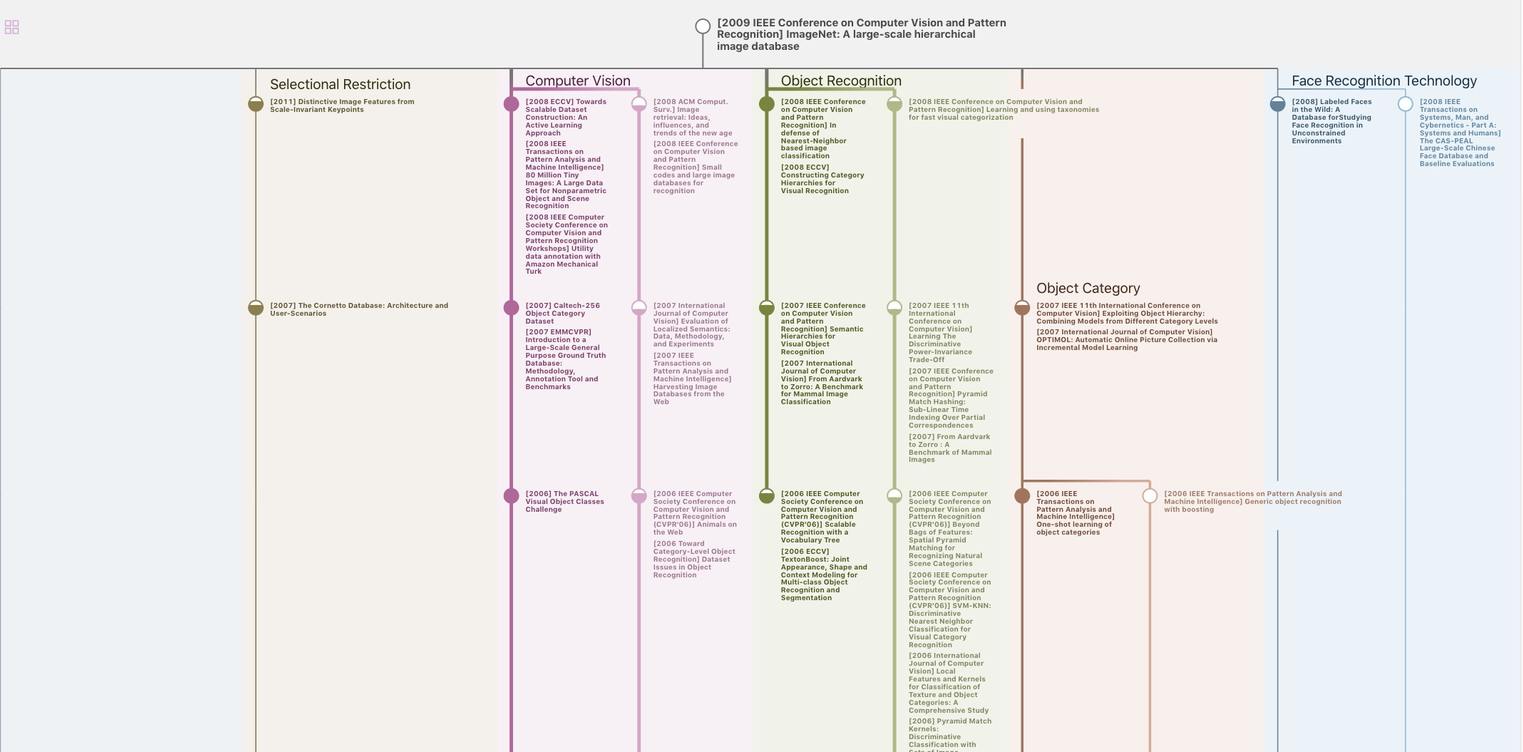
生成溯源树,研究论文发展脉络
Chat Paper
正在生成论文摘要