The combination of near-infrared spectroscopy with chemometrics in achieving rapid and accurate determination of rice mildew
Journal of Food Measurement and Characterization(2024)
摘要
This study addresses the escalating issue of rice spoilage during storage, which significantly affects rice quality. Utilizing near-infrared spectroscopy (NIRS) in conjunction with chemometrics, including partial least squares regression (PLSR), support vector machine regression (SVR), and back-propagation neural network (BPNN) algorithms, we aimed to quantify mold counts in three distinct rice varieties: Northern japonica rice Hongke 389, Southern japonica rice Huai rice No.5, and Indica rice Taiyo. Sample set partitioning methods, including sample set partitioning based on joint x-y distance (SPXY) and Kennard-Stone (KS), were employed for model construction. Various preprocessing techniques, such as maximum-minimum normalization (MMN), multiple scattering correction (MSC), standard normal variate (SNV), and Savitzky-Golay smoothing (SG), SG first-derivative (SG-FD), and SG second-derivative (SG-SD), were applied to enhance spectral data. The optimized models for each rice type were SPXY-SG-BPNN (Hongke 389: R²p = 0.9998, RMSEP = 0.0132), KS-SG-BPNN (Huai rice No.5: R²p = 0.9999, RMSEP = 0.0083), and SPXY-SG-BPNN (Taiyo rice: R²p = 0.9998, RMSEP = 0.0145), showcasing the superior performance of BPNN models in rapid and accurate mold detection. These results underscore the potential of NIRS-chemometrics integration for efficient quality control in stored rice.
更多查看译文
关键词
Near-infrared spectroscopy,Chemometrics,Successful projections algorithm,Mildew,Back-propagation neural network
AI 理解论文
溯源树
样例
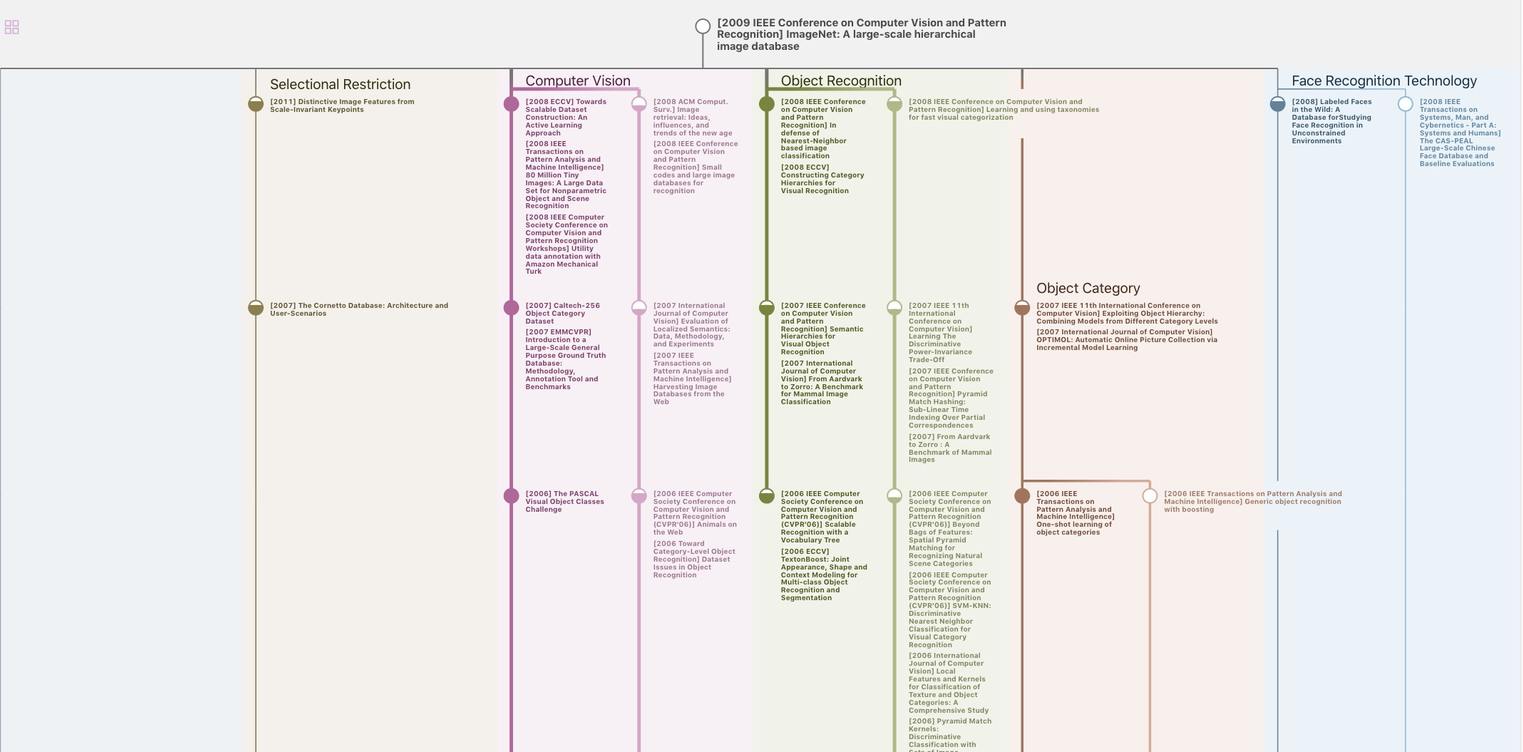
生成溯源树,研究论文发展脉络
Chat Paper
正在生成论文摘要