Investigating leatherback surface behavior using a novel tag design and machine learning
Journal of Experimental Marine Biology and Ecology(2024)
摘要
Understanding the surfacing behavior of marine wildlife is an important component for improving abundance estimates derived from visual surveys. We monitored the behavior of 18 leatherback sea turtles (Dermochelys coriacea) in coastal habitats off Massachusetts, USA, using a high-resolution camera and satellite tag package (HiCAS - High Resolution Camera and Satellite) that we assembled from commercially available components which work independently. We used nine data streams derived from the multiple sensors and a video camera to explore four different depth thresholds defining surface zones. We compared classification of video images by a human to classification of those images by a machine learning algorithm. We calculated four metrics to describe surface behavior for each of the nine data streams. The mean percent time at the surface was the only behavior metric that changed systematically as data streams were used to assess different visible depth thresholds, increasing as the depth threshold increased. Other behavior metrics (mean surface duration, mean dive duration and number of surfacing events per hour) were less similar across data streams, making them unreliable for estimating surface availability. This study highlights the need for sustained data collection to better inform the availability bias estimates used to calculate abundance from visual observations.
更多查看译文
关键词
Sea turtle,Availability,Behavior,Video,Machine learning
AI 理解论文
溯源树
样例
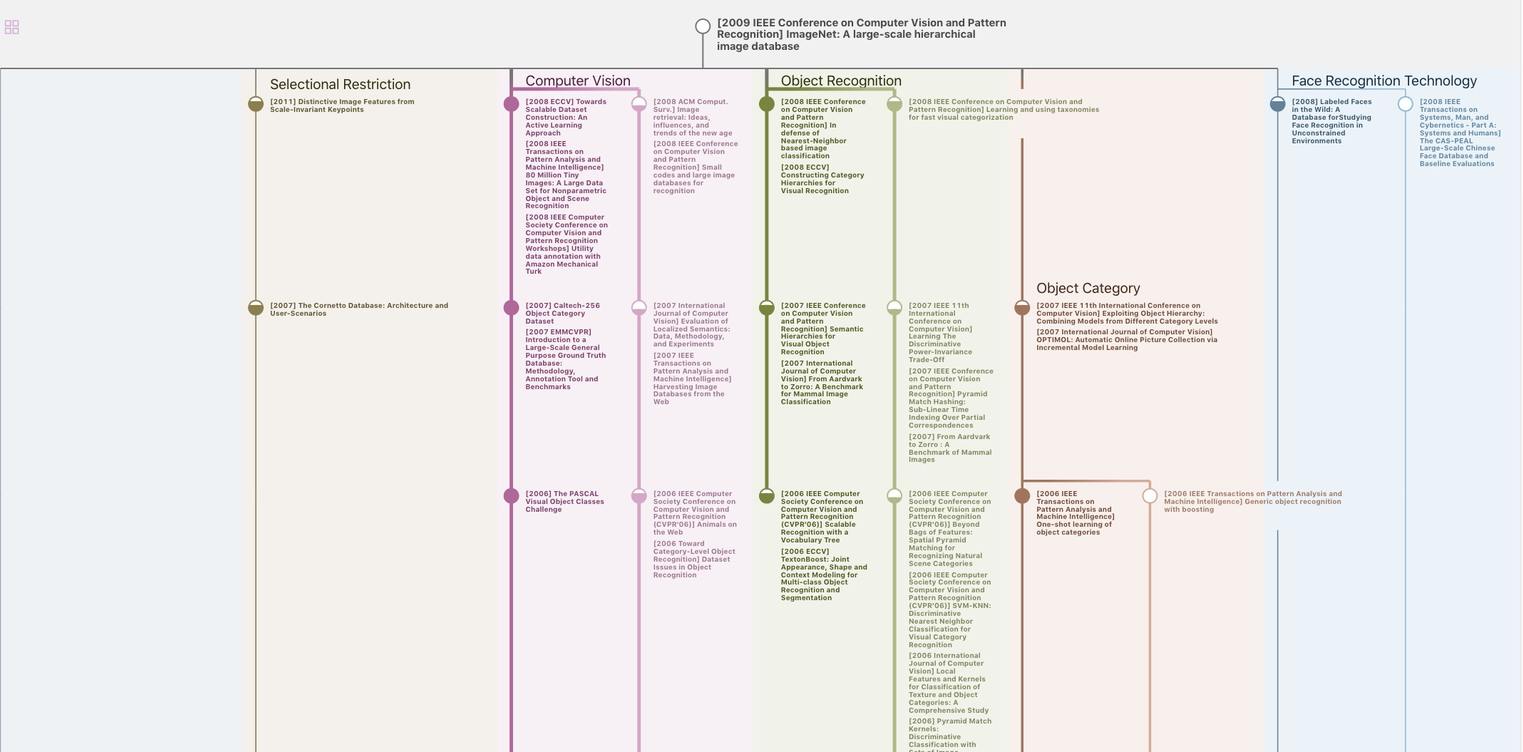
生成溯源树,研究论文发展脉络
Chat Paper
正在生成论文摘要