A fault diagnosis framework based on heterogeneous ensemble learning for air conditioning chiller with unbalanced samples
Measurement Science and Technology(2024)
摘要
Abstract The studies on air conditioning fault diagnosis based on big data have developed rapidly in recent years. However, the data imbalance phenomenon due to the small amount of fault data and the large amount of normal data shared in engineering will trigger problems such as poor model prediction and low accuracy. In order to solve the problem of imbalance fault diagnosis of air-conditioning chillers, this paper proposes an ensemble learning-based diagnostic model, which achieves diagnosis by combining multiple base models and through a majority voting method. The method uses four classification models, random forest model, decision tree model, k-nearest neighbor model, and isomorphic ensemble model, as base classifiers and synthesizes the four base classifiers into a heterogeneous ensemble algorithm through ensemble learning, and performs diagnostic detection of seven types of typical faults of chiller units using the majority voting method of integrated learning. The effectiveness of the proposed algorithm is verified on the RP-1043 dataset, and the experimental results show that the accuracy of the heterogeneous ensemble model can reach 96.87%, which is a significant improvement compared with the accuracy of the other four base classifier models (81.04% to 96.25%). Therefore, it can be considered that ensemble learning model is a promising way to solve the problem of unbalanced data fault diagnosis in engineering.
更多查看译文
AI 理解论文
溯源树
样例
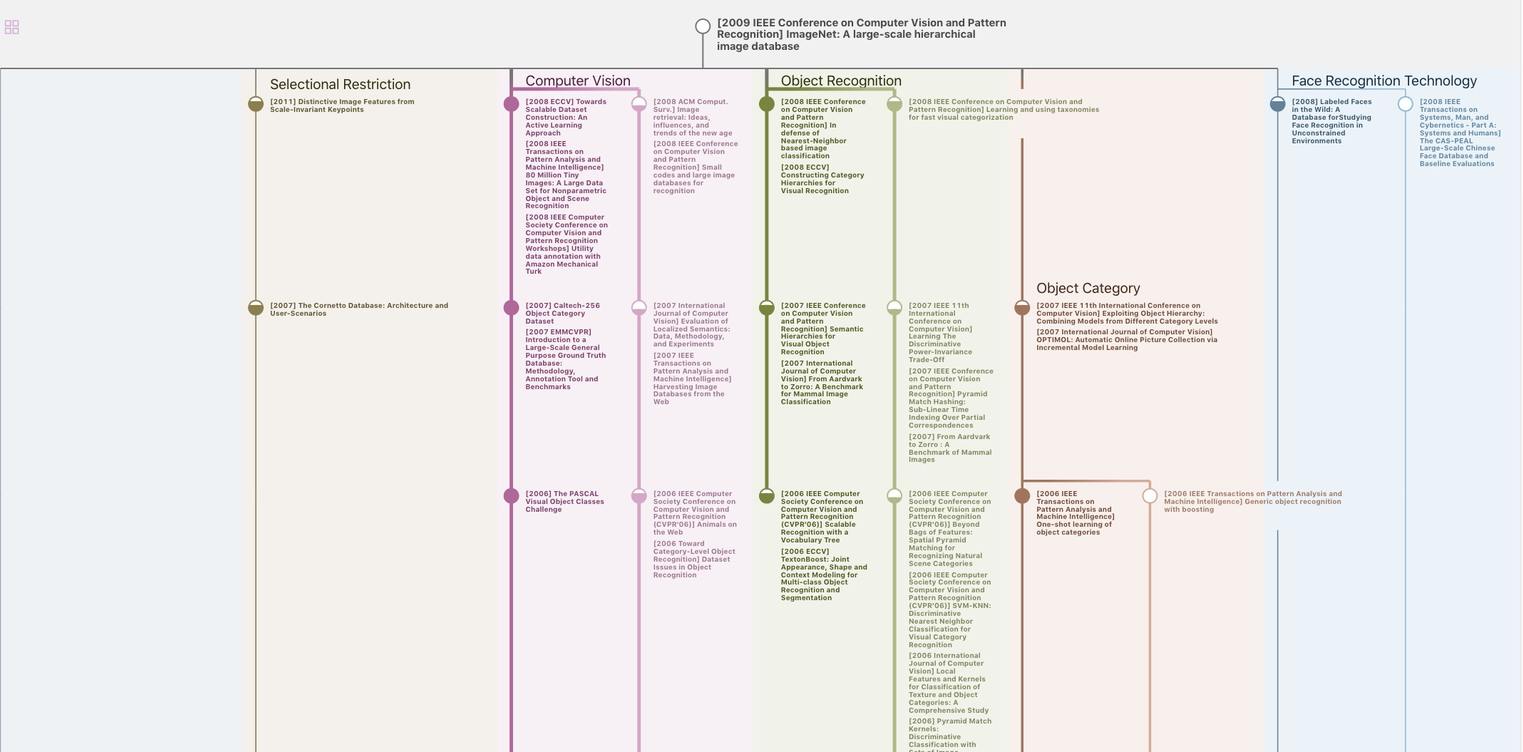
生成溯源树,研究论文发展脉络
Chat Paper
正在生成论文摘要