An efficient analytic solution for joint blind source separation
IEEE Transactions on Signal Processing(2024)
摘要
Joint blind source separation (JBSS) is a powerful methodology for analyzing multiple related datasets, able to jointly extract sources that describe statistical dependencies across the datasets. However, JBSS can be computationally prohibitive with high-dimensional data, thus there exists a key need for more efficient JBSS algorithms. JBSS algorithms typically rely on numerical solutions, which may be expensive due to their iterative nature. In contrast, analytic solutions follow consistent procedures that are often less expensive. In this paper, we introduce an efficient analytic solution for JBSS. Denoting a set of sources dependent across the datasets as a “source component vector” (SCV), our solution minimizes correlation among separate SCVs by minimizing distance of the SCV cross-covariance’s eigenvector matrix from a block diagonal matrix. Under the orthogonality constraint, this leads to a system of linear equations wherein each subproblem has an analytic solution. We derive identifiability conditions of our solution’s estimator, and demonstrate estimation performance and time efficiency in comparison with other JBSS algorithms that exploit source correlation across datasets. Results demonstrate that our solution achieves the lowest asymptotic computational complexity among JBSS algorithms, and is capable of superior estimation performance compared with algorithms of similar complexity.
更多查看译文
关键词
Joint Blind Source Separation,Independent Vector Analysis,Multiset Canonical Correlation Analysis
AI 理解论文
溯源树
样例
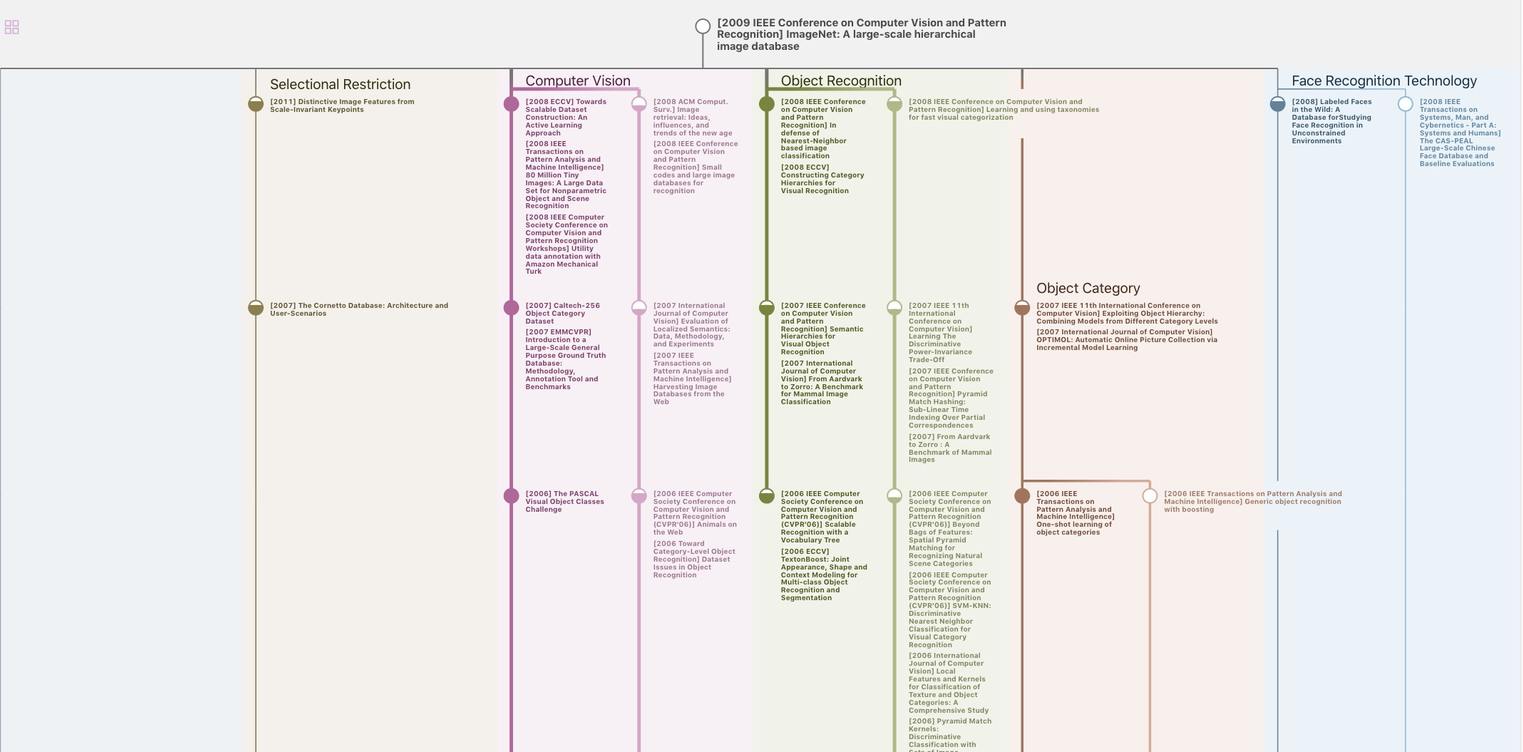
生成溯源树,研究论文发展脉络
Chat Paper
正在生成论文摘要