Attention-Augmented MADDPG in NOMA-Based Vehicular Mobile Edge Computational Offloading
IEEE Internet of Things Journal(2024)
摘要
Vehicular mobile edge computing (vMEC) and non-orthogonal multiple access (NOMA) have emerged as promising technologies for enabling low-latency and high-throughput applications in vehicular networks. In this paper, we propose a novel multi-agent deep deterministic policy gradient (MADDPG) approach for resource allocation in NOMA-based vMEC systems. Our approach leverages deep reinforcement learning (DRL) to enable vehicles to offload computation-intensive tasks to nearby edge servers, optimizing resource allocation decisions while ensuring low-latency communication. We introduce an attention mechanism within the MADDPG model to dynamically focus on relevant information from the input state and joint actions, enhancing the model’s predictive accuracy. Additionally, we propose an attention-based experience replay method to expedite network convergence. The simulation results highlight the effectiveness of multi-agent reinforcement learning (MARL) algorithms, such as MADDPG with attention, in achieving better convergence and performance in various scenarios. The influence of different model parameters, such as input data volumes, task load levels, and resource configurations, on optimization results is also evident. The decision making processes of agents are dynamic and depend on factors specific to the task and environment.
更多查看译文
关键词
Deep Reinforce Learning,NOMA,vMEC,Computational Offloading,MADDPG,Attention Mechanism
AI 理解论文
溯源树
样例
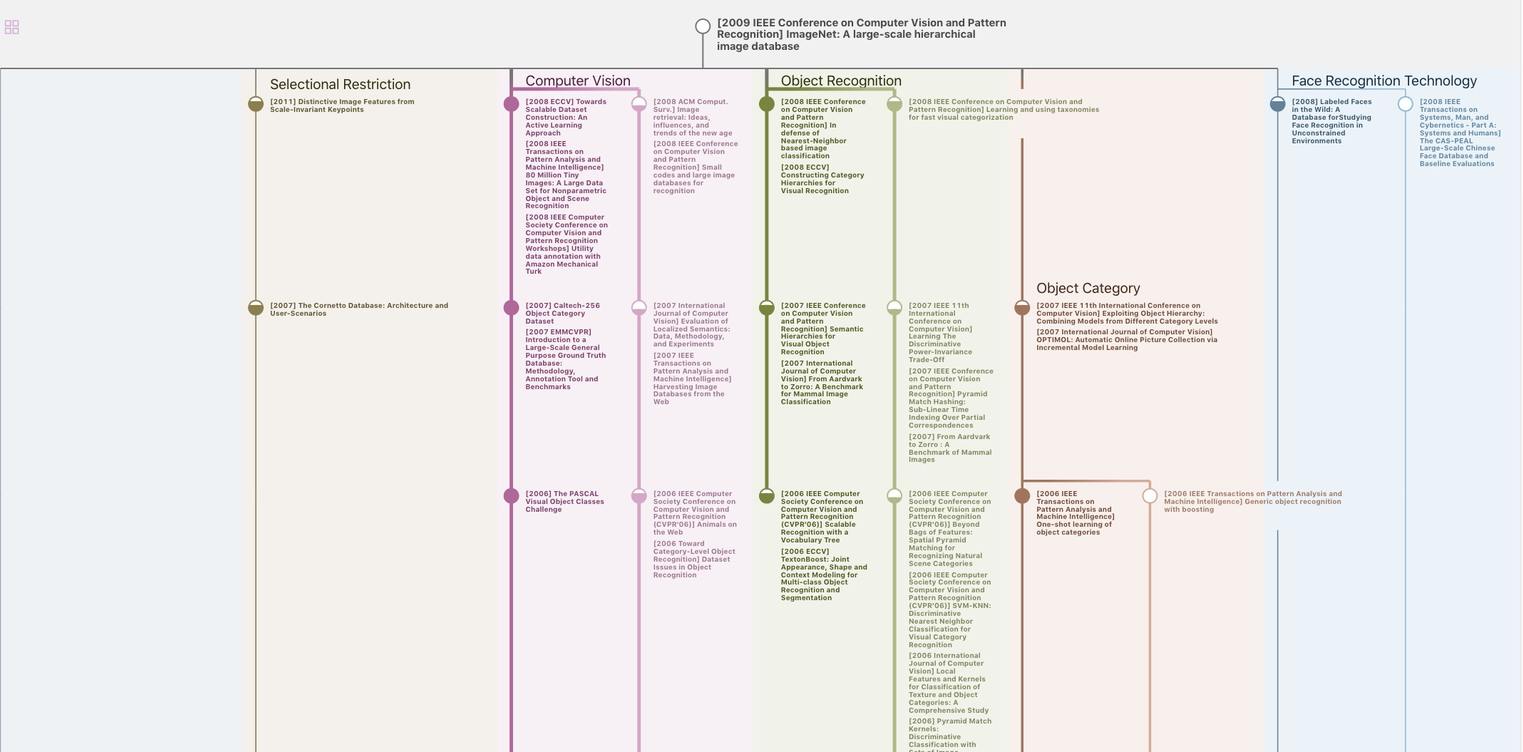
生成溯源树,研究论文发展脉络
Chat Paper
正在生成论文摘要