Data augmentation experiments with style-based quantum generative adversarial networks on trapped-ion and superconducting-qubit technologies
arxiv(2024)
摘要
In the current noisy intermediate scale quantum computing era, and after the
significant progress of the quantum hardware we have seen in the past few
years, it is of high importance to understand how different quantum algorithms
behave on different types of hardware. This includes whether or not they can be
implemented at all and, if so, what the quality of the results is. This work
quantitatively demonstrates, for the first time, how the quantum generator
architecture for the style-based quantum generative adversarial network (qGAN)
can not only be implemented but also yield good results on two very different
types of hardware for data augmentation: the IBM bm_torino quantum computer
based on the Heron chip using superconducting transmon qubits and the aria-1
IonQ quantum computer based on trapped-ion qubits. The style-based qGAN,
proposed in 2022, generalizes the state of the art for qGANs and allows for
shallow-depth networks. The results obtained on both devices are of comparable
quality, with the aria-1 device delivering somewhat more accurate results than
the ibm_torino device, while the runtime on ibm_torino is significantly shorter
than on aria-1. Parallelization of the circuits, using up to 48 qubits on IBM
quantum systems and up to 24 qubits on the IonQ system, is also presented,
reducing the number of submitted jobs and allowing for a substantial reduction
of the runtime on the quantum processor to generate the total number of
samples.
更多查看译文
AI 理解论文
溯源树
样例
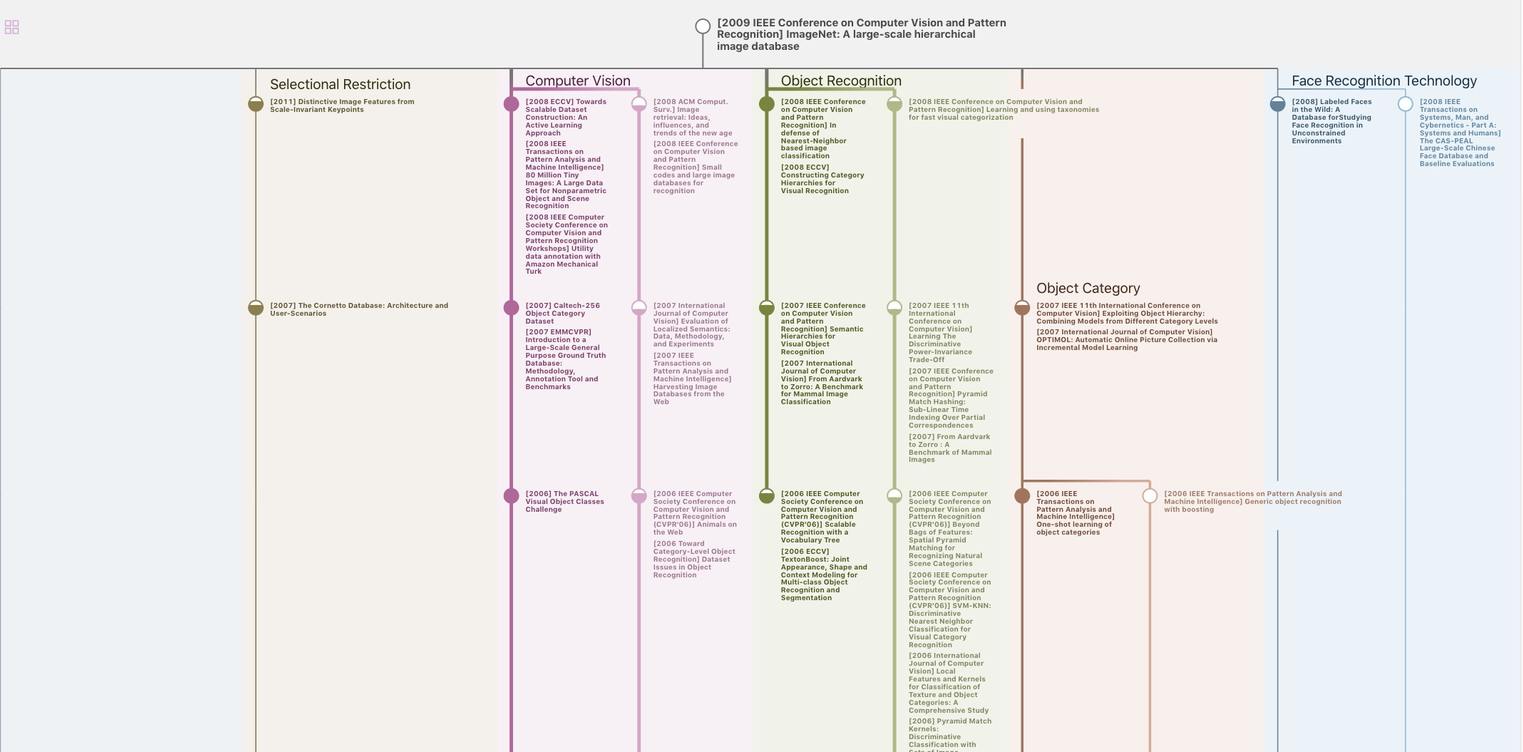
生成溯源树,研究论文发展脉络
Chat Paper
正在生成论文摘要