Fault-transformer: an automatic fault detection algorithm on seismic images using a transformer enhanced neural network
Interpretation(2024)
摘要
Seismic fault detection is a key step in seismic interpretation and reservoir characterization that often requires a large amount of human labor and interpretation time. Therefore, automatic seismic fault detection is critical for improving the efficiency of seismic data processing and interpretation. Existing AI methods are mostly based on convolutional neural networks (CNNs) with a U-shaped encoder-decoder structure, known as the U-net. However, the convolution is limited in modeling long-range correlative features. Instead, Transformers, utilizing self-attention mechanisms, avoid the local nature of the convolution, which has the potential to extract long-distance correlations. Transformers are proven to perform well in natural language processing, image classification, and segmentation tasks in precision and recall. Here, we develop a new deep neural network with transformers and a U-net-like structure: Fault-Transformer, to perform the fault detection task. The new network outperforms traditional U-net in the application with synthetic data sets.
更多查看译文
AI 理解论文
溯源树
样例
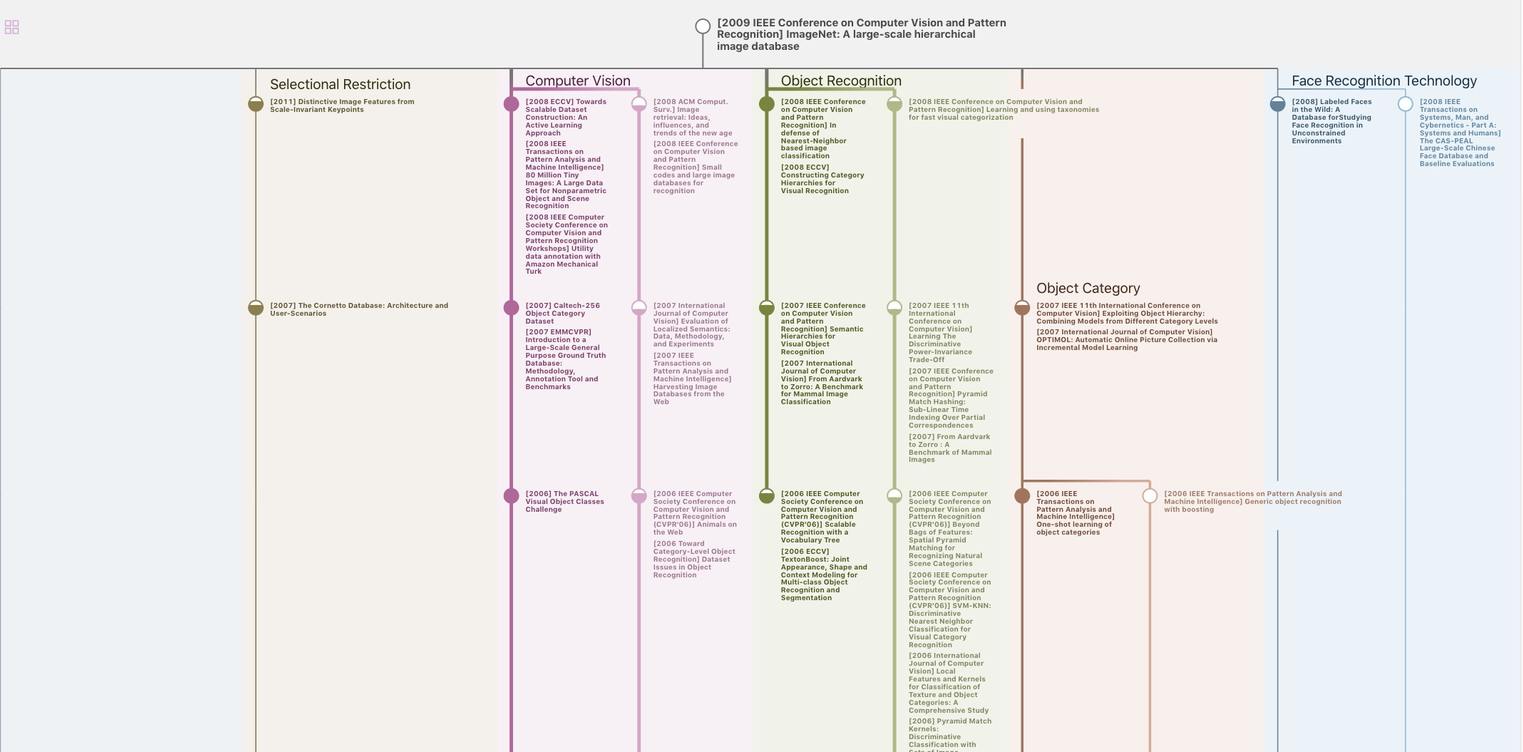
生成溯源树,研究论文发展脉络
Chat Paper
正在生成论文摘要