Attribute reduction for heterogeneous data based on monotonic relative neighborhood granularity
International Journal of Approximate Reasoning(2024)
摘要
The neighborhood rough set model serves as an important tool for handling attribute reduction tasks involving heterogeneous attributes. However, measuring the relationship between conditional attributes and decision in the neighborhood rough set model is a crucial issue. Most studies have utilized neighborhood information entropy to measure the relationship between attributes. When using neighborhood conditional information entropy to measure the relationships between the decision and conditional attributes, it lacks monotonicity, consequently affecting the rationality of the final attribute reduction subset. In this paper, we introduce the concept of neighborhood granularity and propose a new form of relative neighborhood granularity to measure the relationship between the decision and conditional attributes, which exhibits monotonicity. Moreover, our approach for measuring neighborhood granularity avoids the logarithmic function computation involved in neighborhood information entropy. Finally, we conduct comparative experiments on 12 datasets using two classifiers to compare the results of attribute reduction with six other attribute reduction algorithms. The comparison demonstrates the advantages of our measurement approach.
更多查看译文
关键词
Neighborhood rough set model,Attribute reduction,Granular computing,Neighborhood granularity
AI 理解论文
溯源树
样例
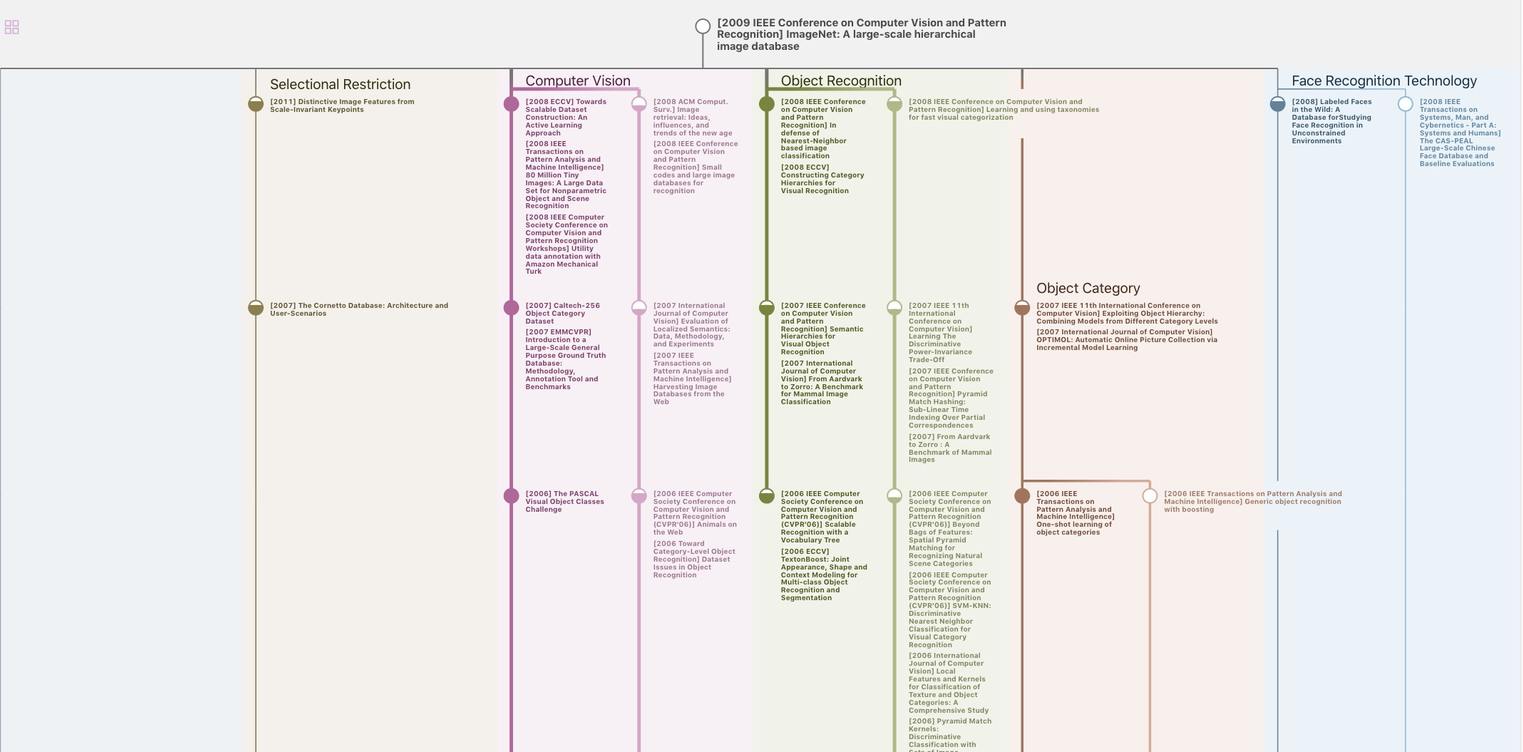
生成溯源树,研究论文发展脉络
Chat Paper
正在生成论文摘要