A Novel Small-Signal Ferroelectric Memcapacitor based Capacitive Computing-In-Memory for Area- and Energy-Efficient Quantized Neural Networks
2024 8th IEEE Electron Devices Technology & Manufacturing Conference (EDTM)(2024)
摘要
In this work, a novel small-signal ferroelectric (FE) memcapacitor (FeCap) based capacitive computing-in-memory (CIM) for quantized neural networks (QNNs) is proposed and experimentally demonstrated. By utilizing the non-monotonic FE small-signal capacitance for signed weight storage and non-destructive readout for input activation, the linearly non-separable signed binary multiplication is implemented in single one FeCap without static DC power consumption, enabling binary neural network with binarized weight and activation. Moreover, by further utilizing the multilevel FE small-signal capacitance with different polarization states, the signed multiplication operation with 3-bit weights is experimentally demonstrated in one FeCap, enabling higher precision of quantized-weight. Based on the proposed FE capacitive CIM, high-accuracy pattern recognition of QNN is demonstrated with $280 \times / 200 \times / 40 \times$ lower energy consumption compared with SRAM-/RRAM-/FeFET-based resistive CIM, showing its high potential for area- and energy-efficient edge AI.
更多查看译文
关键词
Ferroelectric small-signal capacitance,computing in memory,quantized neural network
AI 理解论文
溯源树
样例
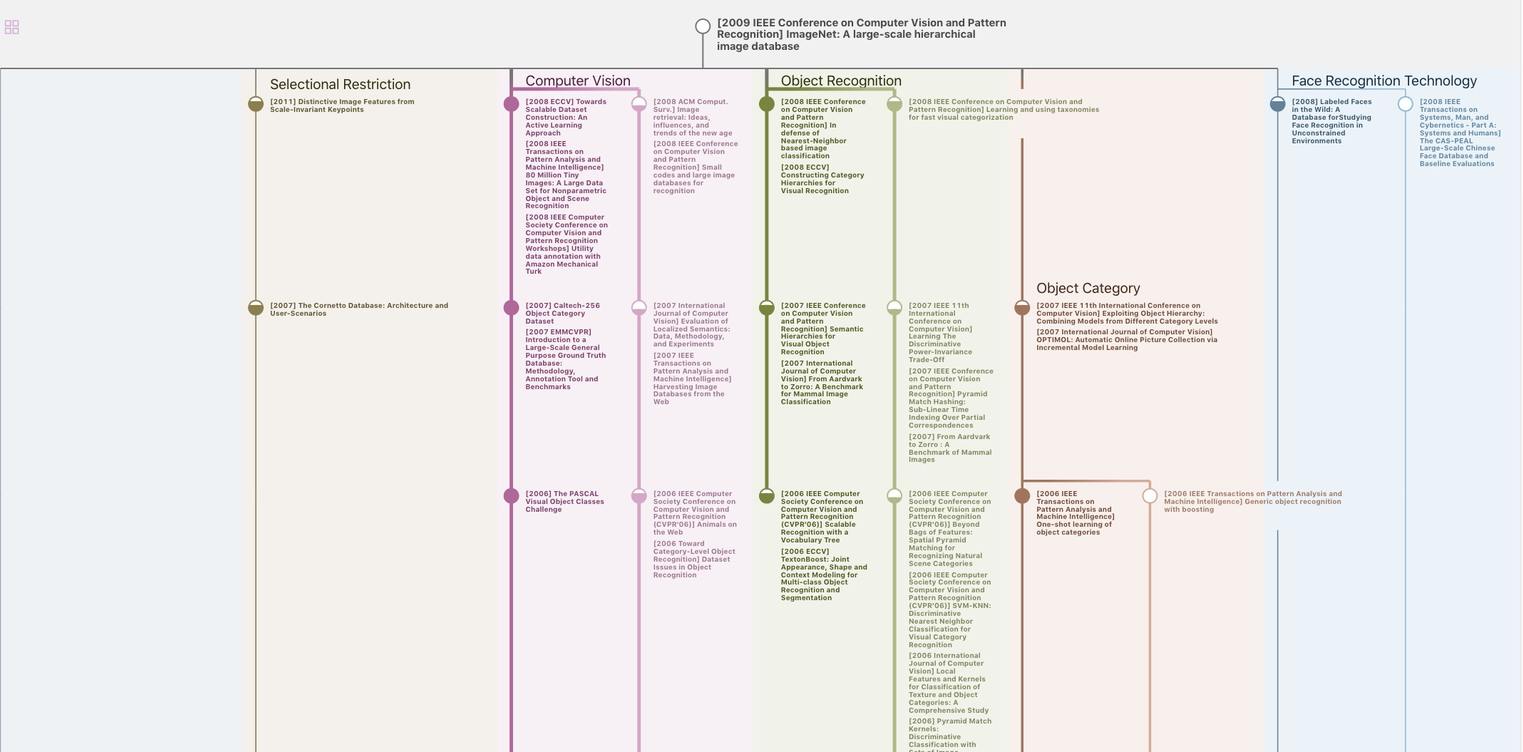
生成溯源树,研究论文发展脉络
Chat Paper
正在生成论文摘要