Bi-Level Graph Structure Learning for Next POI Recommendation
IEEE Transactions on Knowledge and Data Engineering(2024)
摘要
Next point-of-interest (POI) recommendation aims to predict a user's next destination based on sequential check-in history and a set of POI candidates. Graph neural networks (GNNs) have demonstrated a remarkable capability in this endeavor by exploiting the extensive global collaborative signals present among POIs. However, most of the existing graph-based approaches construct graph structures based on pre-defined heuristics, failing to consider inherent hierarchical structures of POI features such as geographical locations and visiting peaks, or suffering from noisy and incomplete structures in graphs. To address the aforementioned issues, this paper presents a novel
Bi
-level
G
raph
S
tructure
L
earning (
${\sf BiGSL}$
) for next POI recommendation.
${\sf BiGSL}$
first learns a hierarchical graph structure to capture the fine-to-coarse connectivity between POIs and prototypes, and then uses a pairwise learning module to dynamically infer relationships between POI pairs and prototype pairs. Based on the learned bi-level graphs, our model then employs a multi-relational graph network that considers both POI- and prototype-level neighbors, resulting in improved POI representations. Our bi-level structure learning scheme is more robust to data noise and incompleteness, and improves the exploration ability for recommendation by alleviating sparsity issues. Experimental results on three real-world datasets demonstrate the superiority of our model over existing state-of-the-art methods, with a significant improvement in recommendation accuracy and exploration performance.
更多查看译文
关键词
Contrastive multiview fusion,graph structure learning,hierarchical structure,next POI recommendation
AI 理解论文
溯源树
样例
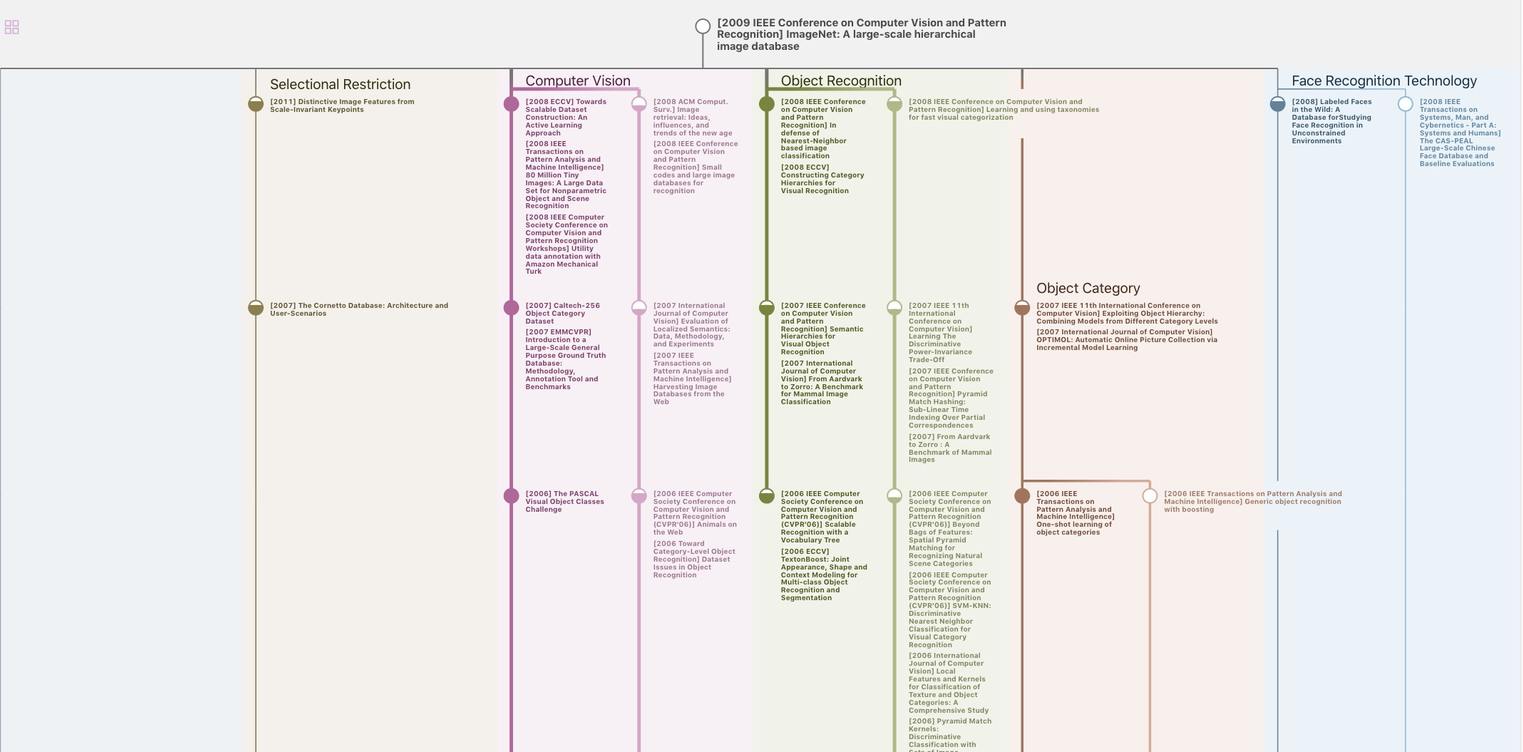
生成溯源树,研究论文发展脉络
Chat Paper
正在生成论文摘要