Diamond-Unet: A Novel Semantic Segmentation Network Based on U-Net Network and Transformer for Deep Space Rock Images.
IEEE Geosci. Remote. Sens. Lett.(2024)
摘要
Extracting rock objects from the surface of celestial bodies in deep space exploration environments is crucial for self-service path planning, navigation of detectors, and regional information evaluation. Most existing image saemantic segmentation frameworks decrease the spatial resolution of the feature maps as networks deepen, resulting in limitations in detecting small targets and the inability to accurately segment boundary regions. In this letter, we propose a novel semantic segmentation network based on U-Net network and Transformer for deep space rock images, referred to as Diamond-Unet. This model integrates overcomplete and undercomplete branches and incorporates a global-local feature extraction (GLFE) module based on Transformer and CNN technologies to effectively capture discriminative information. Furthermore, an innovative feature cross-fusion path (FCFP) is introduced to enhance information exchange between the dual-branch networks, enabling the capture of both fine-grained details and coarse-grained semantics in the full-scale image segmentation architecture. Experimental results demonstrate that the Diamond-Unet achieves
MIoU
scores of 79.32% and 93.43% on two public datasets, which are superior to the compared methods.
更多查看译文
关键词
Deep space exploration,image segmentation,transformer,feature cross-fusion
AI 理解论文
溯源树
样例
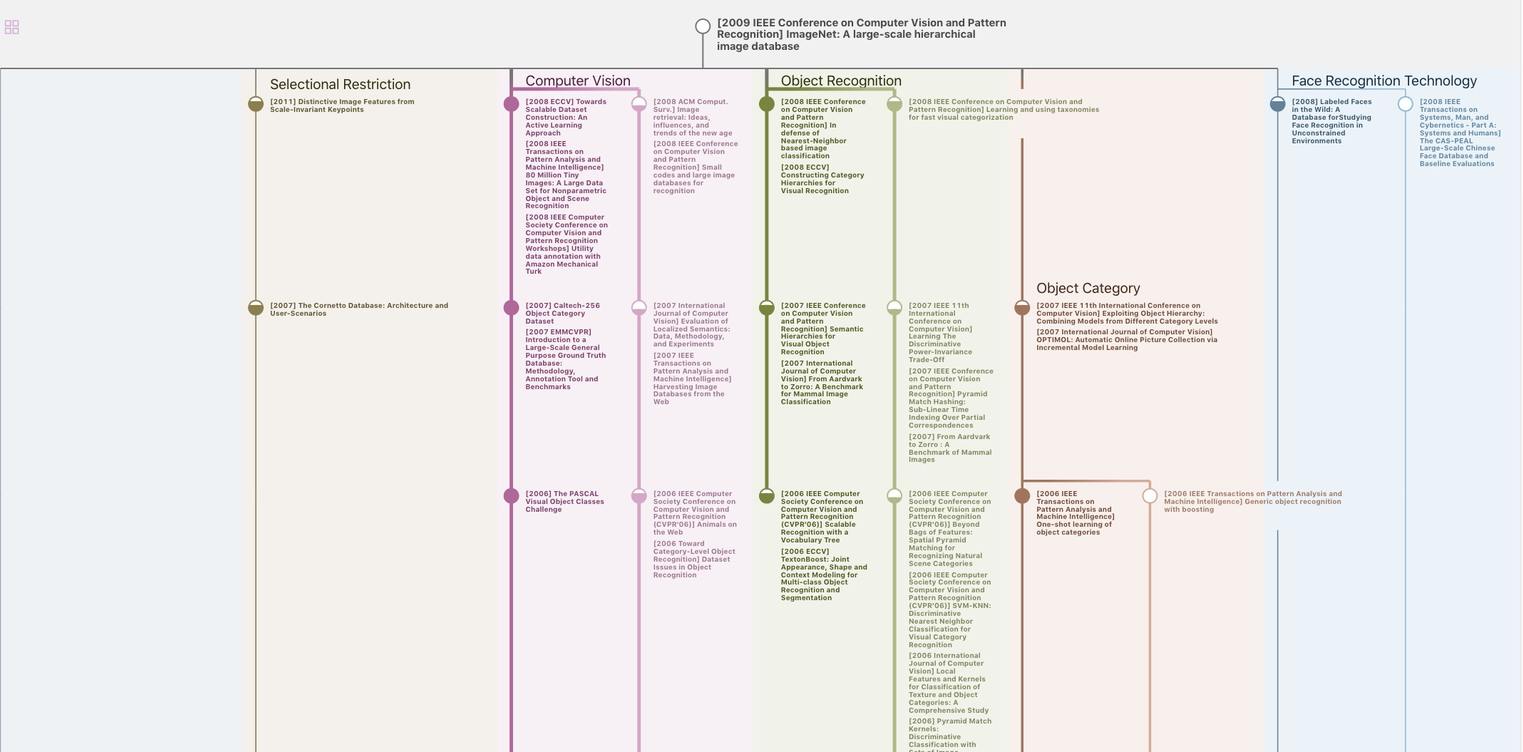
生成溯源树,研究论文发展脉络
Chat Paper
正在生成论文摘要