An end-cloud collaboration approach for online state-of-health estimation of lithium-ion batteries based on multi-feature and transformer
Journal of Power Sources(2024)
摘要
State-of-Health (SOH) estimation of lithium-ion batteries is critical for reliable and efficient energy storage and usage. Traditional SOH estimation approaches either compromise on real-time performance or on estimation accuracy. This paper introduces an end-cloud collaboration approach that synergistically combines a cloud-based deep learning model with an end-based empirical model to achieve both high-accuracy and real-time SOH estimation. Utilizing the Transformer architecture, the cloud-side model employs multiple features extracted through signal analysis technology. On the other hand, the end-side employs a fast, iterative double exponential empirical model that provides real-time estimates. Two methods of end-cloud collaborative updating are presented, both leveraging Kalman Filter and Unscented Kalman Filter (UKF) to integrate and iteratively update the models. Evaluation shows that the cloud-side model achieves a Root Mean Square Error (RMSE) and Mean Absolute Error (MAE) of around 0.8 %, while the end-cloud collaboration system maintains these metrics at 1 %, successfully capturing nonlinear capacity rebound phenomena. The work contributes to the domain by offering an approach that balances computational load and latency, making it suitable for online applications. Under the Cyber Hierarchy and Interactional Network (CHAIN) framework, this end-cloud collaboration architecture holds promise for high-accuracy, real-time health management of lithium-ion batteries across their full lifecycle.
更多查看译文
关键词
State of health,Data-driven,End-cloud collaboration,Transformer,Unscented Kalman filter
AI 理解论文
溯源树
样例
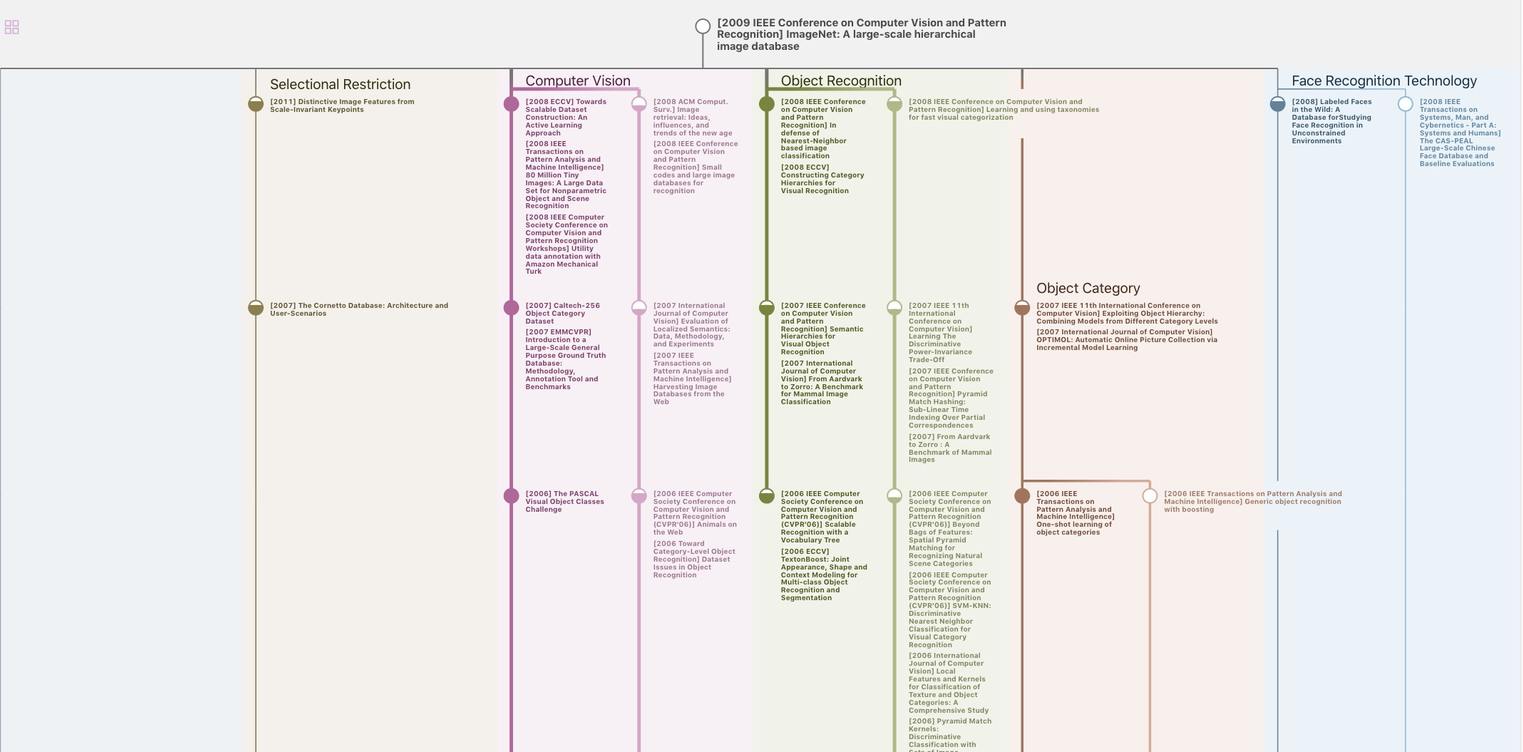
生成溯源树,研究论文发展脉络
Chat Paper
正在生成论文摘要