ALP-Net: a segmentation-free approach for license plate recognition in unconstrained scenarios
Neural Computing and Applications(2024)
摘要
License plate recognition technology is of paramount importance in intelligent transportation. While ideal scenario license plate recognition technology has gradually matured, traditional methods still exhibit limitations in performance under unconstrained scenarios. Although network models based on the recurrent neural network structure address some shortcomings of traditional methods to a certain extent, they constraints in recognition speed. To address these issues, we introduce and implements ALP-Net, a novel structural model, aimed at enhancing the robustness and efficiency of license plate recognition in diverse and challenging scenarios. The model utilizes a fully convolutional structure and custom attention mechanism modules (GFE-Block, ALP-Attention) to ensure speed while significantly improving recognition for low-quality license plates. Introducing synthetic data and a dynamic random perturbation mechanism further enhances the model’s generalization ability. We believe this model can elevate performance while maintaining a low computational complexity. Extensive experiments indicate that our approach outperforms state-of-the-art methods on the CCPD dataset and demonstrates robust generalization capabilities on the CLPD and CRPD datasets. The code and datasets can be available from https://github.com/HeYaoting666/ALP-Net .
更多查看译文
关键词
License plate recognition,Convolutional network,Attention mechanism,Dynamic random perturbation
AI 理解论文
溯源树
样例
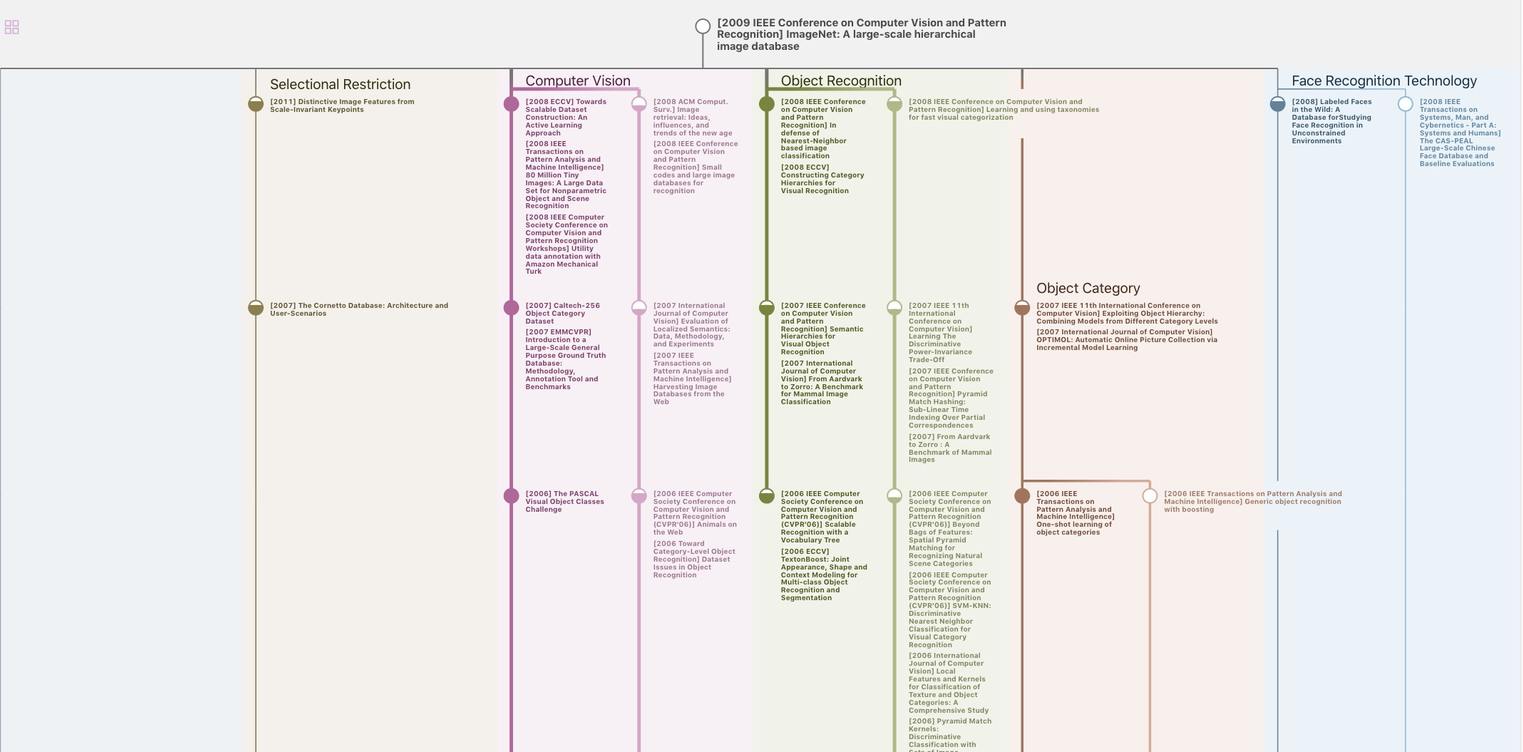
生成溯源树,研究论文发展脉络
Chat Paper
正在生成论文摘要