Adaptive Spatial–Temporal Aware Graph Learning for EEG-Based Emotion Recognition
Cyborg and Bionic Systems(2024)
摘要
An intelligent emotion recognition system based on electroencephalography (EEG) signals shows considerable potential in various domains such as healthcare, entertainment, and education, thanks to its portability, high temporal resolution, and real-time capabilities. However, the existing research in this field faces limitations stemming from the nonstationary nature and individual variability of EEG signals. In this study, we present a novel EEG emotion recognition model, named GraphEmotionNet, designed to enhance the accuracy of EEG-based emotion recognition through the incorporation of a spatiotemporal attention mechanism and transfer learning. The proposed GraphEmotionNet model can effectively learn the intrinsic connections between EEG channels and construct an adaptive graph. This graph’s adaptive nature is crucial in optimizing spatial–temporal graph convolutions, which in turn enhances spatial–temporal feature characterization and contributes to the process of emotion classification. Moreover, an integration of domain adaptation aligns the extracted features across different domains, further alleviating the impact of individual EEG variability. We evaluate the model performance on two benchmark databases, employing two types of cross-validation protocols: within-subject cross-validation and cross-subject cross-validation. The experimental results affirm the model’s efficacy in extracting EEG features linked to emotional semantics and demonstrate its promising performance in emotion recognition.
更多查看译文
AI 理解论文
溯源树
样例
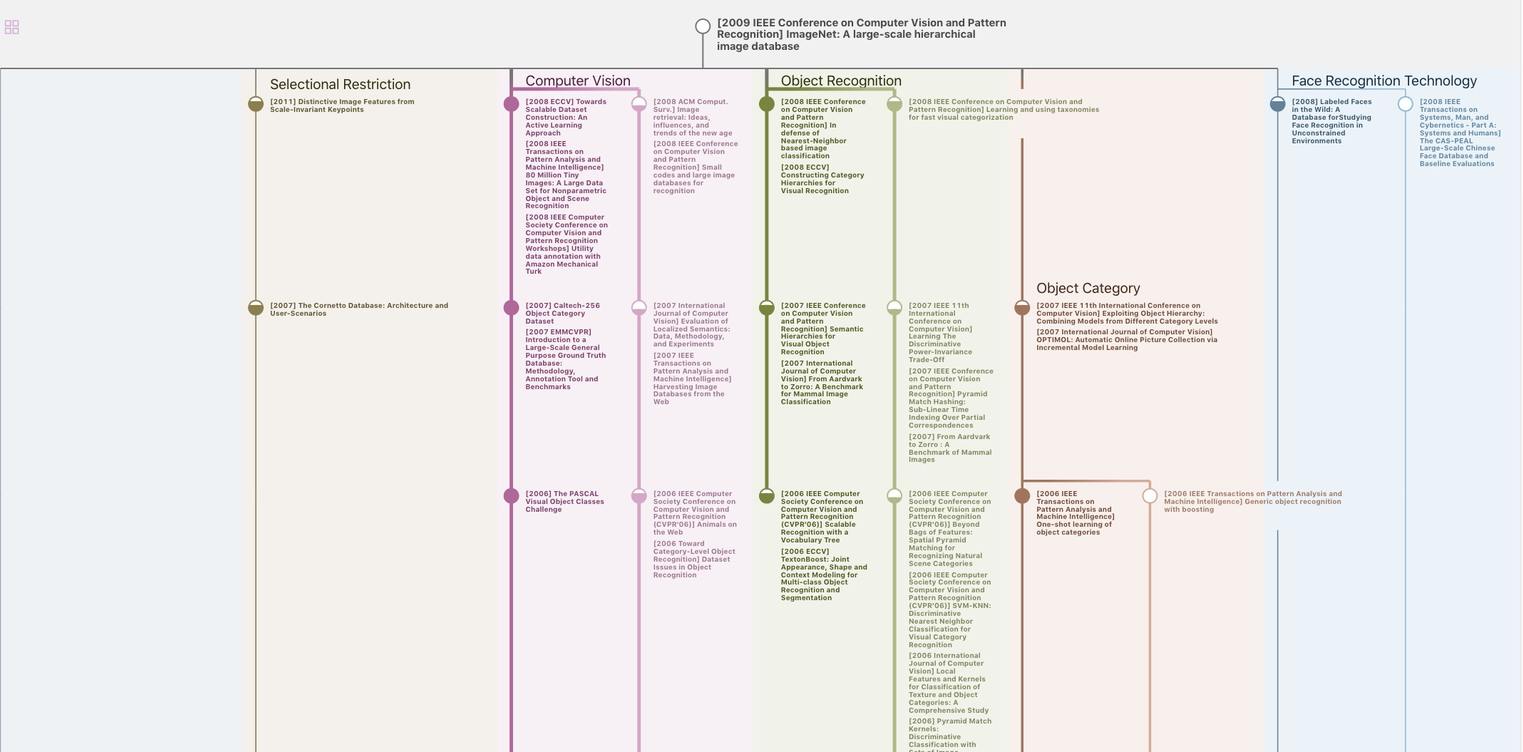
生成溯源树,研究论文发展脉络
Chat Paper
正在生成论文摘要