Performance prediction and operating parameters optimization for proton exchange membrane fuel cell based on data-driven surrogate model and particle swarm optimization
International Journal of Hydrogen Energy(2024)
摘要
The operating parameters of proton exchange membrane fuel cell (PEMFC) are critical to its performance and working life. This study presents a data-driven modeling approach combining a surrogate model with the particle swarm optimization algorithm to optimize operating parameters for PEMFC and obtain the maximum power density. The results show that the operating parameters significantly influence power density under high current densities, with inlet temperature having the most significant effect. Lower inlet temperature, relative humidity in the cathode and anode, along with higher operating pressure yield improved output performance. The Genetic Algorithm-Backpropagation Neural Network based surrogate model exhibits excellent predictive performance with correlation coefficients of 0.99896 and 0.99815 for the training and test sets, respectively. Optimized conditions achieve a 3.3% increase in power density compared to initial settings, with only a 0.15% error in simulation calculations. This data-driven approach provides valuable insights for maximizing PEMFC efficiency and performance.
更多查看译文
关键词
PEMFC,Data-driven,Particle swarm optimization,Operating condition,Maximum power density
AI 理解论文
溯源树
样例
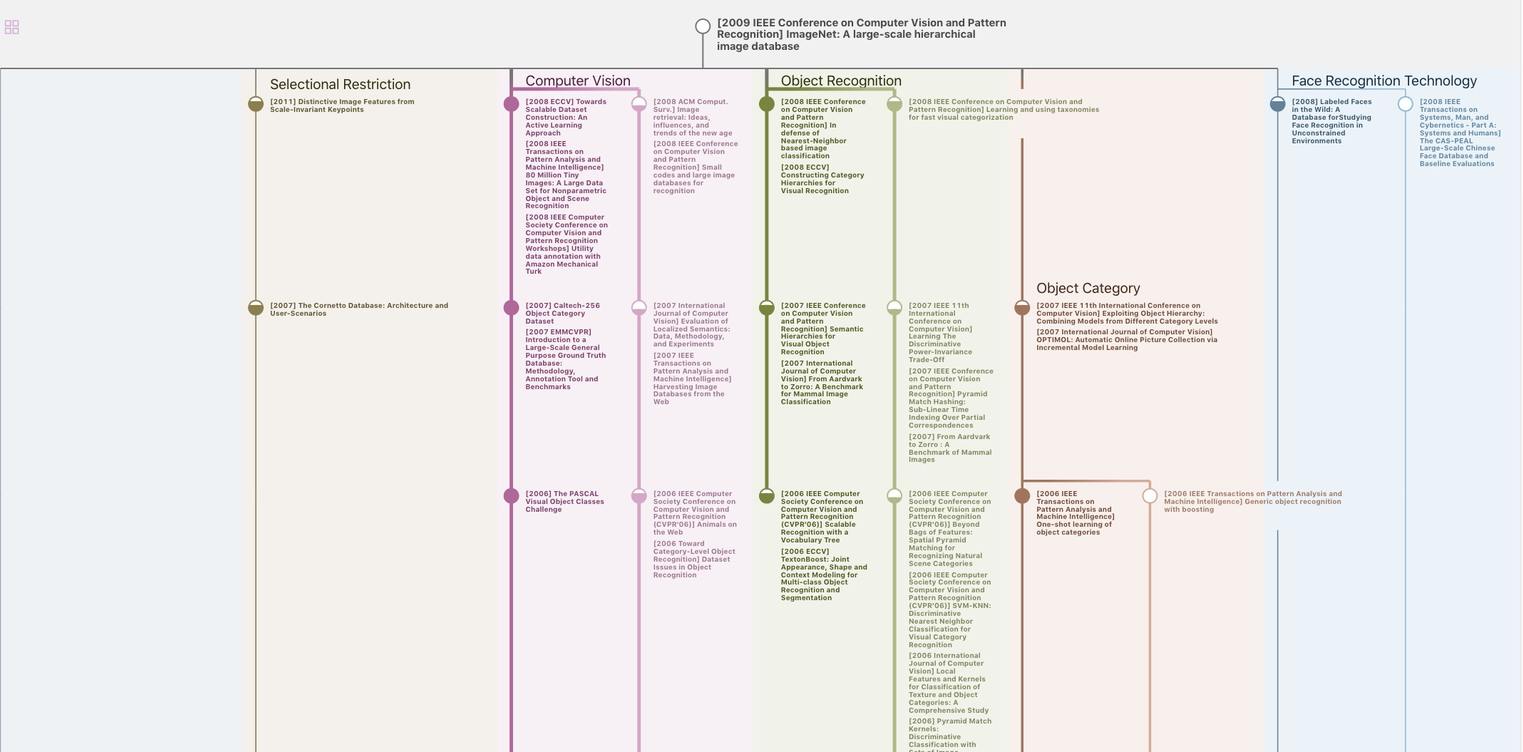
生成溯源树,研究论文发展脉络
Chat Paper
正在生成论文摘要