Exploring Amplified Heterogeneity Arising From Heavy-Tailed Distributions in Federated Learning
IEEE Transactions on Mobile Computing(2024)
摘要
Federated Learning (FL) has emerged as a privacy-preserving paradigm enabling collaborative model training among distributed clients. However, current FL methods operate under the closed-world assumption, i.e., all local training data originates from a global labeled dataset balanced across classes, which is often invalid for practical scenarios. In contrast, in many open-world settings, data have been observed to exhibit heavy-tailed distributions, particularly in the realm of mobile computing and Internet of Things (IoT). Heavy-tailed data can have a significant negative impact on the performance of learning algorithms due to amplifying the heterogeneity in the FL environment. To this end, we introduce a novel framework to counter biased training caused by diverse and imbalanced classes. This framework includes a balance-aware reward aggregation mechanism addressing local majority and global minority class disparities. Rewards are assigned based on client class prevalence for fair aggregation. A calibration module supplements global aggregation to manage conflicts from inconsistent data distribution among clients. Using reward aggregation and calibration, we effectively mitigate heavy-tailed distribution effects, enhancing FL model performance. This framework seamlessly integrates with leading FL methods, demonstrated through extensive experiments on benchmark and real-world datasets.
更多查看译文
关键词
Federated learning,heavy-tailed distributions,statistical heterogeneity
AI 理解论文
溯源树
样例
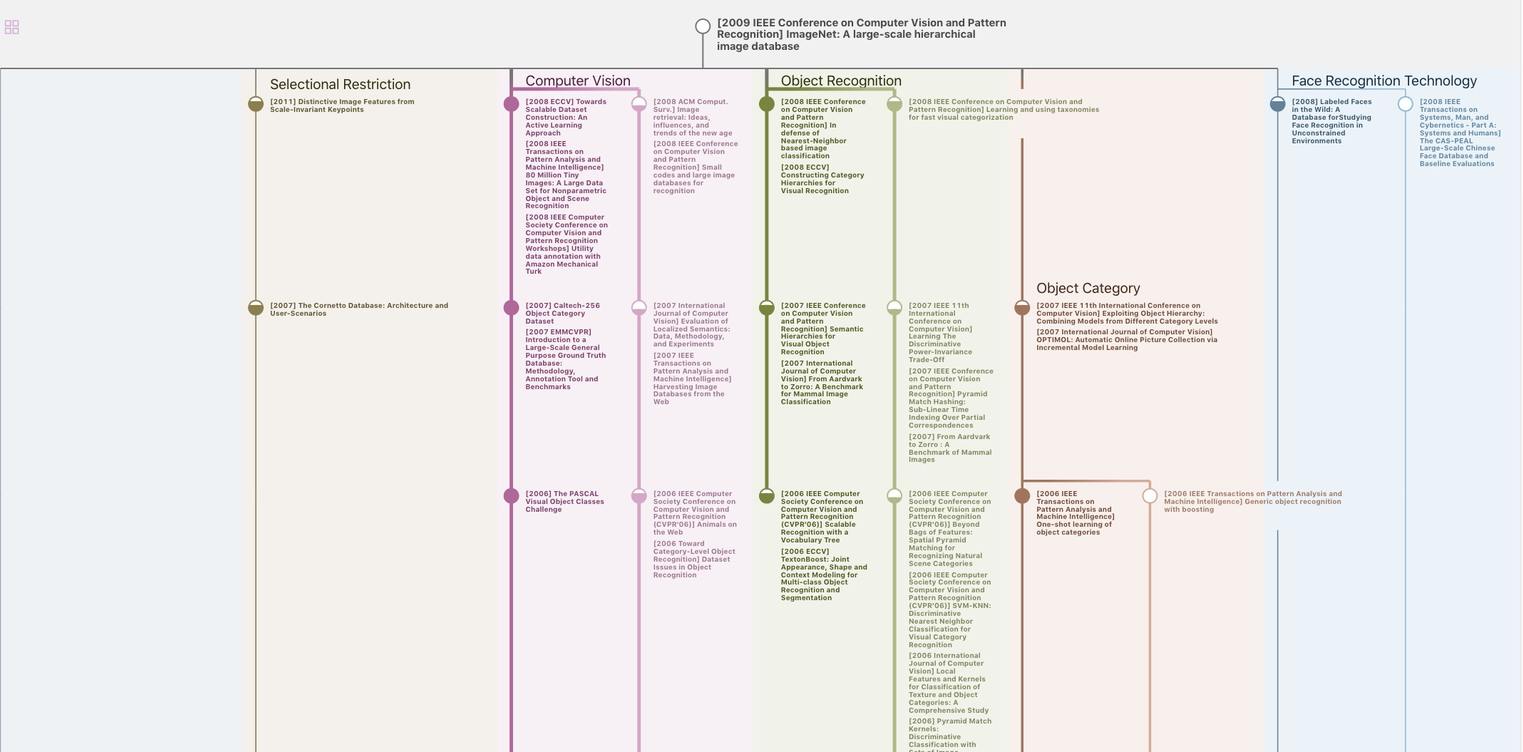
生成溯源树,研究论文发展脉络
Chat Paper
正在生成论文摘要