Power and Latency Collaborative Optimizations of Object Detection CNN on Edge Devices Under Limited Energy Budget.
International Conference on Advanced Cloud and Big Data(2023)
摘要
Real-time object detection with remote sensing technologies often requires drones and other battery-driven edge devices in situ to take images at high altitudes. In some cases, tasks are carried out where there exists no Internet access that is stable or reliable enough, making it impractical to transfer obtained data to a cloud server and have them processed there, especially during tasks that require real-time detection results. But processing remote sensing data on an edge device comes with many obstacles, with limited energy budget arguably being the biggest one of them. In this paper, we propose a method to adjust computational load on GPU and CPU on the fly when executing CNN inference, specifically that of YOLOX, on three common Nvidia MPSoC models, by making use of genetic algorithm to find an optimized deployment. We conducted extensive experiments to gather essential data and carried out simulations on the said device and observed uptime increase of up to over 158% compared to GPU-only deployment, with acceptable to no performance degradation and accurate detection results in line with the original implementation. In future work, we expect that it can be applied to more CNN-based models and algorithms, and with more adjustable variables to meet more various demands on diverse scenarios in the field of remote sensing.
更多查看译文
关键词
edge computing,object detection,remote sensing,CNN,power-consumption optimization,field research
AI 理解论文
溯源树
样例
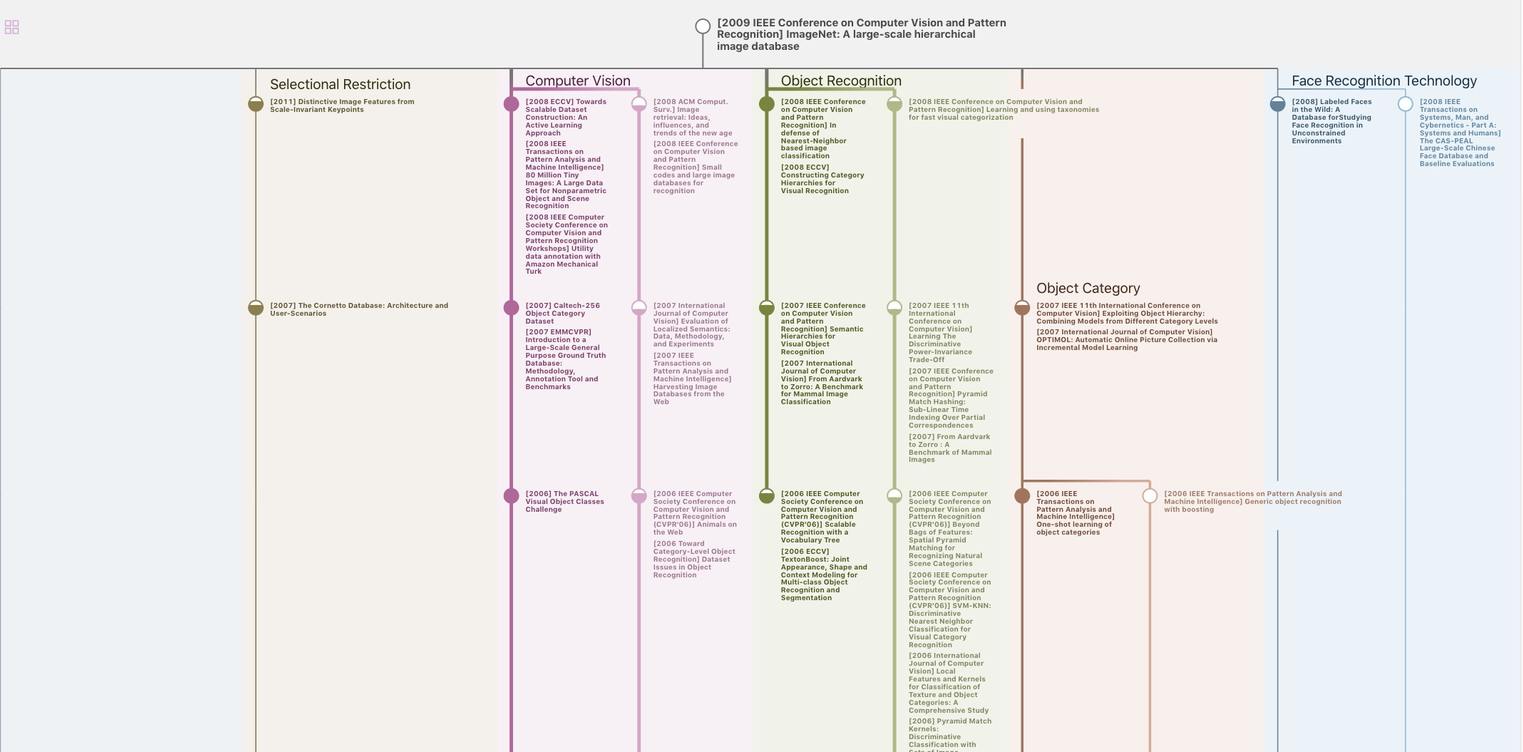
生成溯源树,研究论文发展脉络
Chat Paper
正在生成论文摘要