DMA-HPCNet: Dual Multi-level Attention Hybrid Pyramid Convolution Neural Network for Alzheimer's Disease Classification.
IEEE transactions on neural systems and rehabilitation engineering : a publication of the IEEE Engineering in Medicine and Biology Society(2024)
摘要
Computer-aided diagnosis (CAD) plays a crucial role in the clinical application of Alzheimer's disease (AD). In particular, convolutional neural network (CNN)-based methods are highly sensitive to subtle changes caused by brain atrophy in medical images (e.g., magnetic resonance imaging, MRI). Due to computational resource constraints, most CAD methods focus on quantitative features in specific regions, neglecting the holistic nature of the images, which poses a challenge for a comprehensive understanding of pathological changes in AD. To address this issue, we propose a lightweight dual multi-level hybrid pyramid convolutional neural network (DMA-HPCNet) to aid clinical diagnosis of AD. Specifically, we introduced ResNet as the backbone network and modularly extended the hybrid pyramid convolution (HPC) block and the dual multi-level attention (DMA) module. Among them, the HPC block is designed to enhance the acquisition of information at different scales, and the DMA module is proposed to sequentially extract different local and global representations from the channel and spatial domains. Our proposed DMA-HPCNet method was evaluated on baseline MRI slices of 443 subjects from the ADNI dataset. Experimental results show that our proposed DMA-HPCNet model performs efficiently in AD-related classification tasks with low computational cost.
更多查看译文
关键词
Alzheimer’s disease,pyramid convolution,computer-aided diagnosis,attention mechanism,MRI
AI 理解论文
溯源树
样例
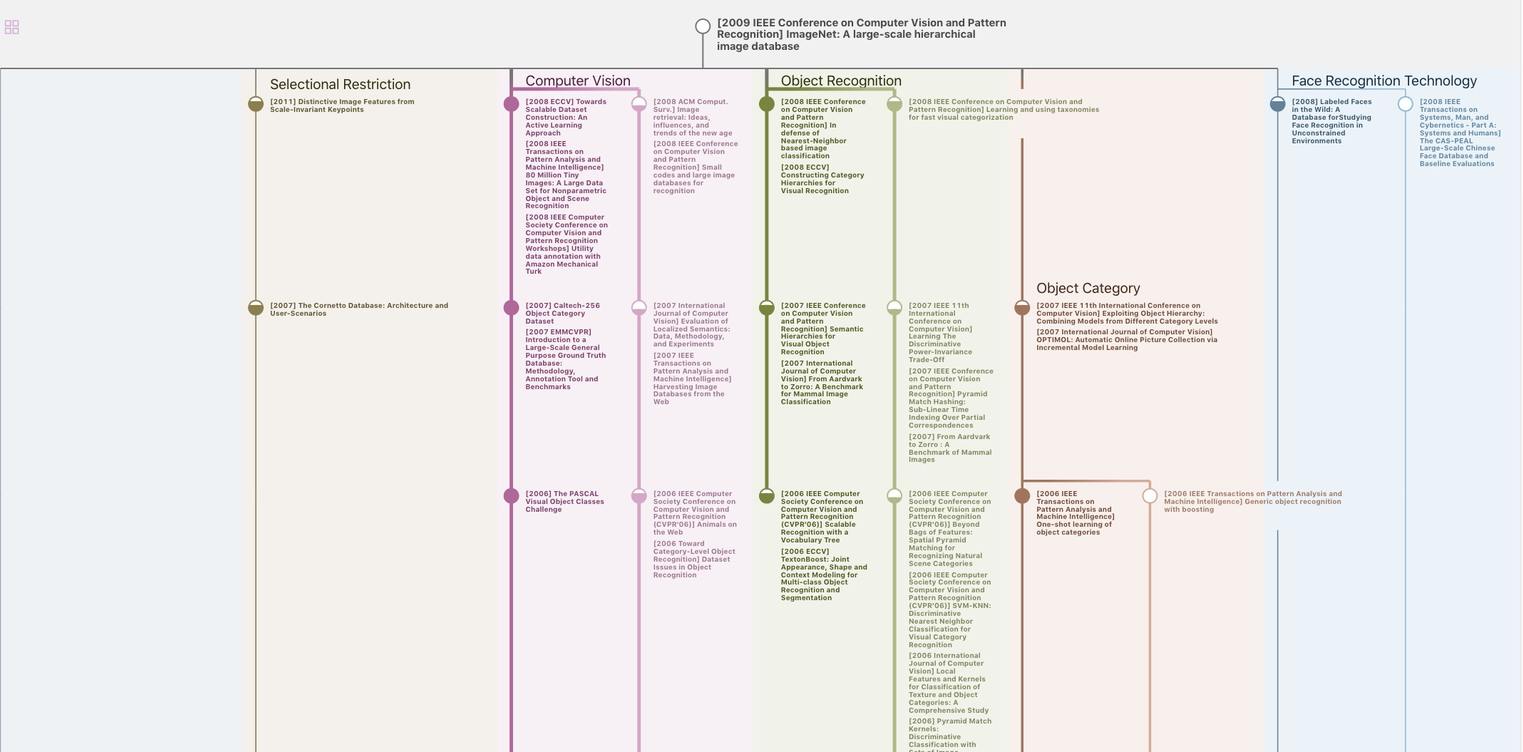
生成溯源树,研究论文发展脉络
Chat Paper
正在生成论文摘要