Federated Adaptation for Foundation Model-based Recommendations
IJCAI 2024(2024)
摘要
With the recent success of large language models, particularly foundation models with generalization abilities, applying foundation models for recommendations becomes a new paradigm to improve existing recommendation systems. It becomes a new open challenge to enable the foundation model to capture user preference changes in a timely manner with reasonable communication and computation costs while preserving privacy. This paper proposes a novel federated adaptation mechanism to enhance the foundation model-based recommendation system in a privacy-preserving manner. Specifically, each client will learn a lightweight personalized adapter using its private data. The adapter then collaborates with pre-trained foundation models to provide recommendation service efficiently with fine-grained manners. Importantly, users' private behavioral data remains secure as it is not shared with the server. This data localization-based privacy preservation is embodied via the federated learning framework. The model can ensure that shared knowledge is incorporated into all adapters while simultaneously preserving each user's personal preferences. Experimental results on four benchmark datasets demonstrate our method's superior performance. The code is available.
更多查看译文
关键词
Machine Learning -> ML: Federated learning,Data Mining -> DM: Privacy-preserving data mining,Data Mining -> DM: Recommender systems
AI 理解论文
溯源树
样例
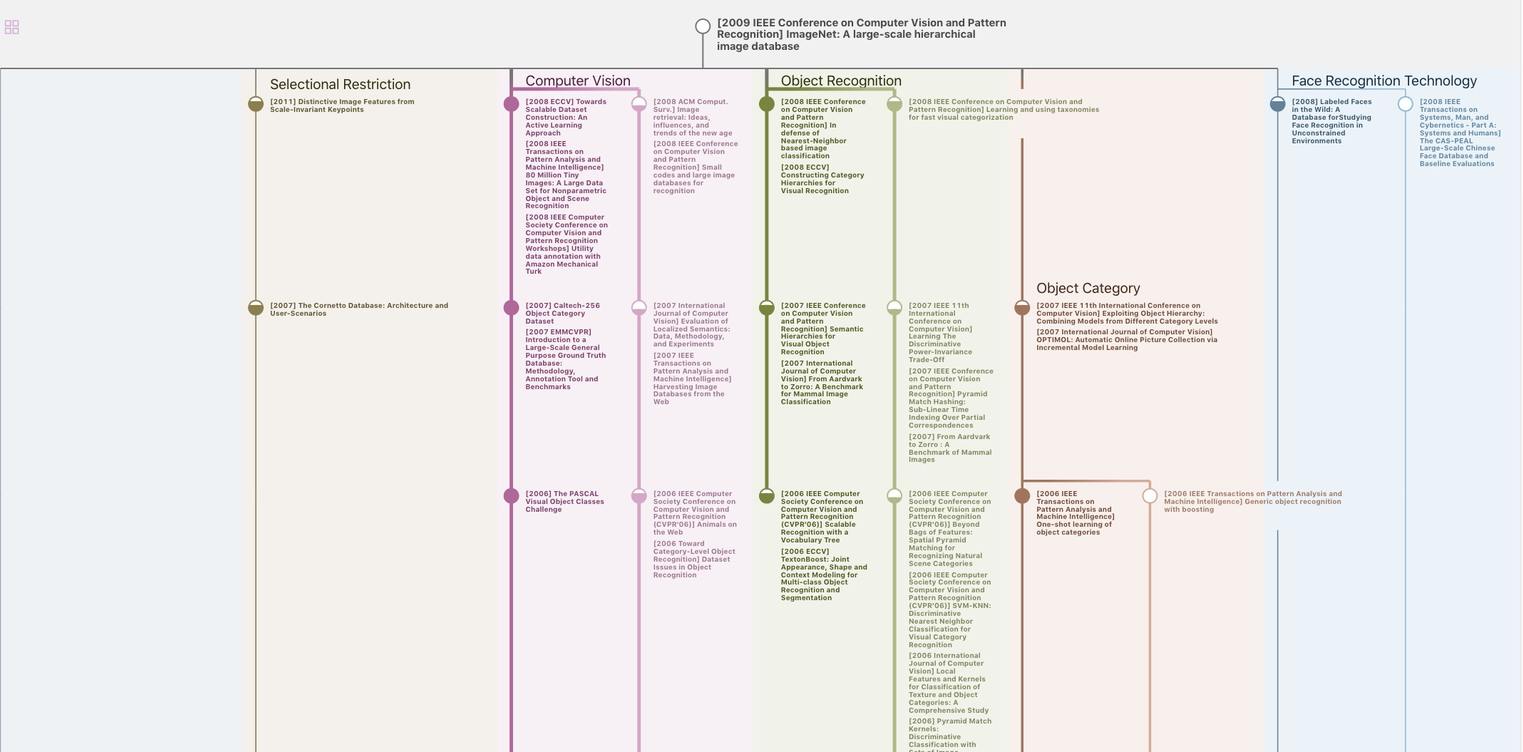
生成溯源树,研究论文发展脉络
Chat Paper
正在生成论文摘要