Enhancing the structure-function relationship in glaucoma using anatomical compensation of retinal nerve fibre layer
BRITISH JOURNAL OF OPHTHALMOLOGY(2024)
摘要
Background/aims To investigate whether compensating retinal nerve fibre layer (RNFL) thickness measurements for demographic and anatomical ocular factors can strengthen the structure-function relationship in patients with glaucoma. Methods 600 eyes from 412 patients with glaucoma (mean deviation of the visual field (MD VF) -6.53 +/- 5.55 dB) were included in this cross-sectional study. Participants underwent standard automated perimetry and spectral-domain optical coherence tomography imaging (Cirrus; Carl Zeiss Meditec). Compensated RNFL thickness was computed considering age, refractive error, optic disc parameters and retinal vessel density. The relationship between MD VF and RNFL thickness measurements, with or without demographic and anatomical compensation, was evaluated sectorally and focally. Results The superior arcuate sector exhibited the highest correlation between measured RNFL and MD VF, with a correlation of 0.49 (95% CI 0.37 to 0.59). Applying the compensated RNFL data increased the correlation substantially to 0.62 (95% CI 0.52 to 0.70; p<0.001). Only 61% of the VF locations showed a significant relationship (Spearman's correlation of at least 0.30) between structural and functional aspects using measured RNFL data, and this increased to 78% with compensated RNFL measurements. In the 10 degrees-20 degrees VF region, the slope below the breakpoint for compensated RNFL thickness demonstrated a more robust correlation (slope=1.66 +/- 0.18 m/dB; p<0.001) than measured RNFL (slope=0.27 +/- 0.67 m/dB; p=0.688). Conclusion Compensated RNFL data improve the correlation between RNFL measurements and VF parameters. This indicates that creating structure-to-function maps that consider anatomical variances may aid in identifying localised structural and functional loss in glaucoma.
更多查看译文
关键词
Glaucoma,Retina,Imaging
AI 理解论文
溯源树
样例
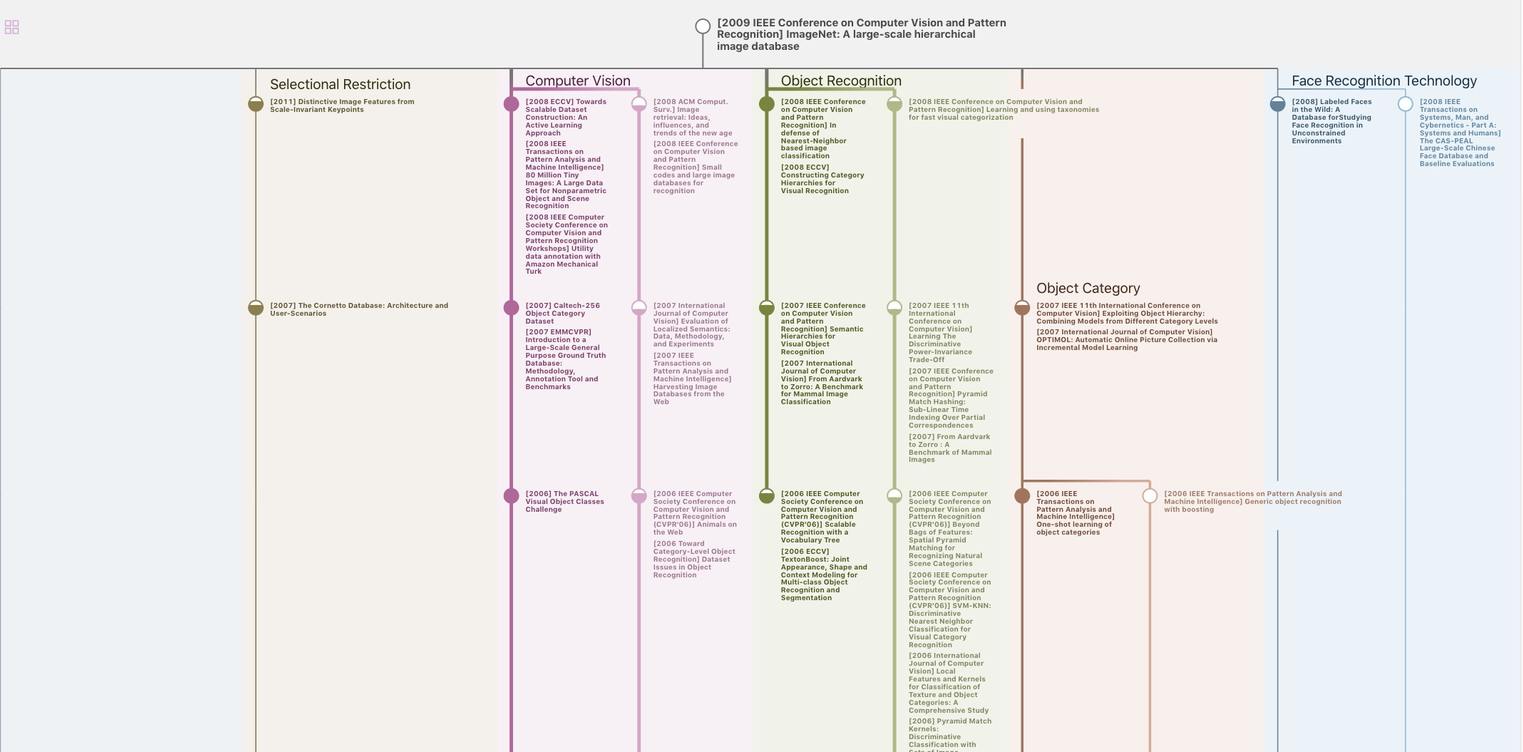
生成溯源树,研究论文发展脉络
Chat Paper
正在生成论文摘要